Face Verification with Disguise Variations via Deep Disguise Recognizer
2018 IEEE/CVF Conference on Computer Vision and Pattern Recognition Workshops (CVPRW)(2018)
摘要
The performance of current automatic face recognition algorithms is hindered by different covariates such as facial aging, disguises, and pose variations. Specifically, disguises are employed for intentional or unintentional modifications in the facial appearance for hiding one's own identity or impersonating someone else's identity. In this paper, we utilize deep learning based transfer learning approach for face verification with disguise variations. We employ Residual Inception network framework with center loss for learning inherent face representations. The training for the Inception-ResNet model is performed using a large-scale face database which is followed by inductive transfer learning to mitigate the impact of facial disguises. To evaluate the performance of the proposed Deep Disguise Recognizer (DDR) framework, Disguised Faces in the Wild and IIIT-Delhi Disguise Version 1 face databases are used. Experimental evaluation reveals that for the two databases, the proposed DDR framework yields 90.36% and 66.9% face verification accuracy at the false accept rate of 10%.
更多查看译文
关键词
deep learning based transfer learning approach,large-scale face database,face representations,face verification accuracy,automatic face recognition algorithms,deep disguise recognizer framework,inception-ResNet model,residual inception network framework,inductive transfer learning,DDR framework,IIIT-Delhi Disguise Version 1 face databases,Wild Disguise Version 1 face databases
AI 理解论文
溯源树
样例
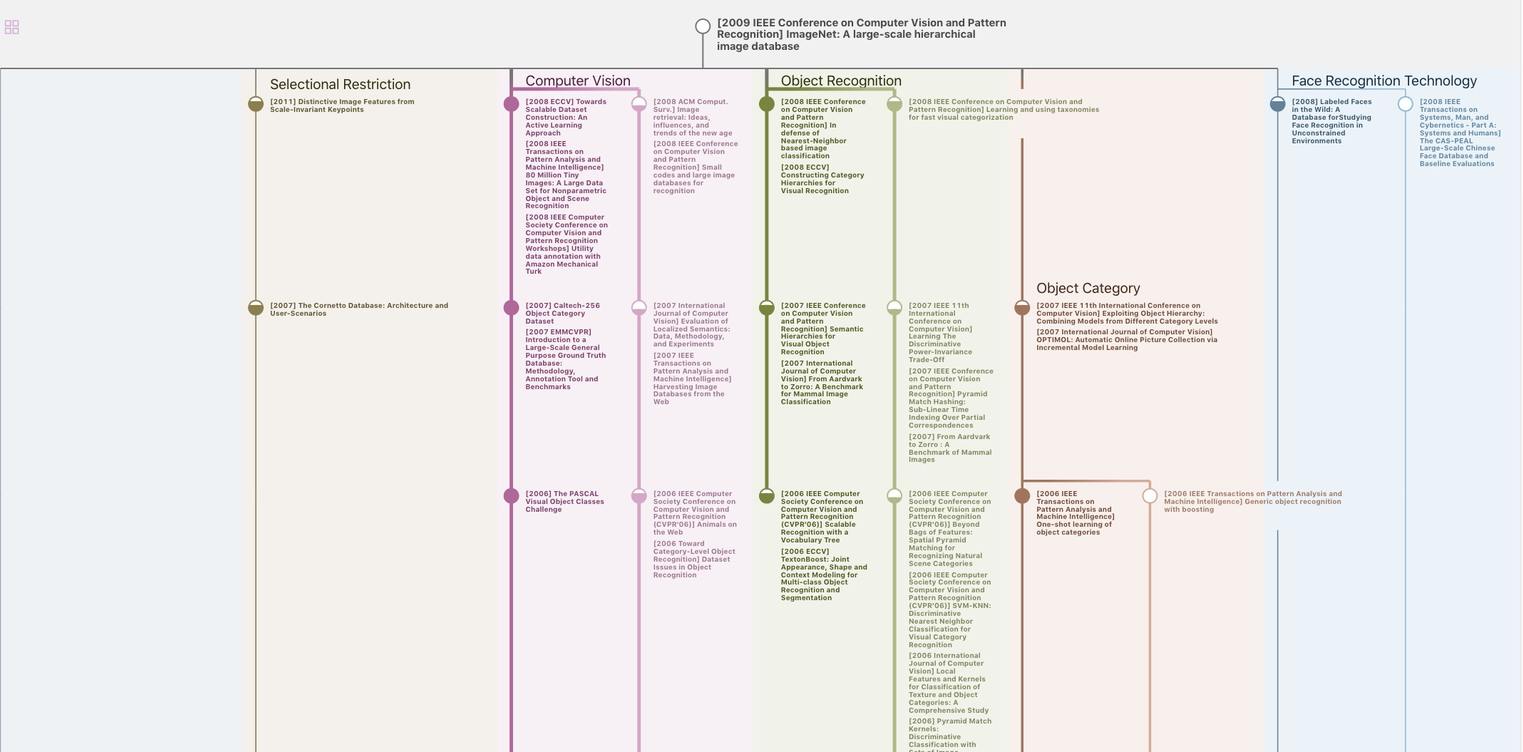
生成溯源树,研究论文发展脉络
Chat Paper
正在生成论文摘要