The Best of Both Worlds: Combining CNNs and Geometric Constraints for Hierarchical Motion Segmentation
2018 IEEE/CVF Conference on Computer Vision and Pattern Recognition(2018)
摘要
Traditional methods of motion segmentation use powerful geometric constraints to understand motion, but fail to leverage the semantics of high-level image understanding. Modern CNN methods of motion analysis, on the other hand, excel at identifying well-known structures, but may not precisely characterize well-known geometric constraints. In this work, we build a new statistical model of rigid motion flow based on classical perspective projection constraints. We then combine piecewise rigid motions into complex deformable and articulated objects, guided by semantic segmentation from CNNs and a second "object-level" statistical model. This combination of classical geometric knowledge combined with the pattern recognition abilities of CNNs yields excellent performance on a wide range of motion segmentation benchmarks, from complex geometric scenes to camouflaged animals.
更多查看译文
关键词
complex geometric scenes,hierarchical motion segmentation,powerful geometric constraints,high-level image understanding,modern CNN methods,motion analysis,rigid motion flow,classical perspective projection constraints,rigid motions,complex deformable objects,articulated objects,semantic segmentation,motion segmentation benchmarks
AI 理解论文
溯源树
样例
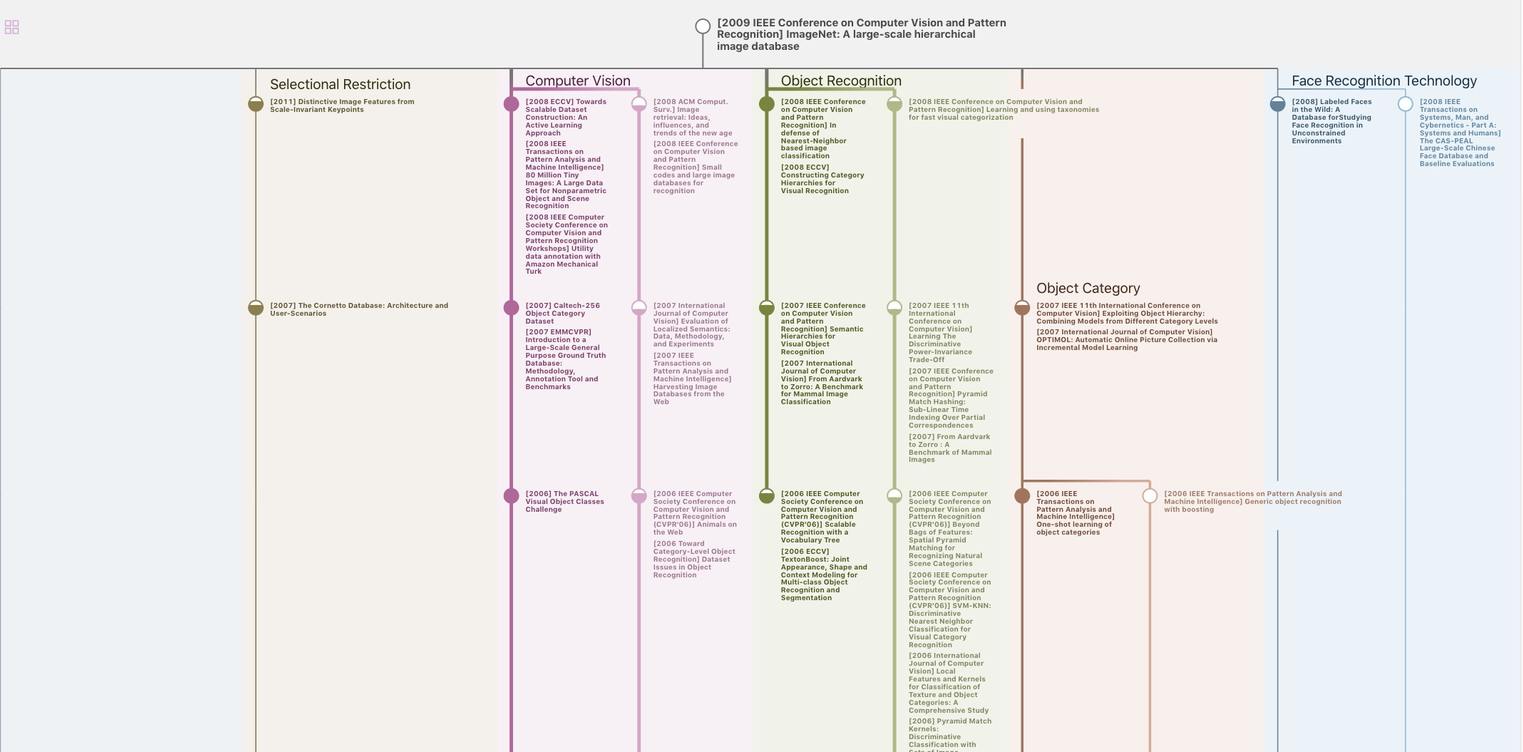
生成溯源树,研究论文发展脉络
Chat Paper
正在生成论文摘要