Modulated Convolutional Networks
2018 IEEE/CVF Conference on Computer Vision and Pattern Recognition(2018)
摘要
Despite great effectiveness of very deep and wide Convolutional Neural Networks (CNNs) in various computer vision tasks, the significant cost in terms of storage requirement of such networks impedes the deployment on computationally limited devices. In this paper, we propose new modulated convolutional networks (MCNs) to improve the portability of CNNs via binarized filters. In MCNs, we propose a new loss function which considers the filter loss, center loss and softmax loss in an end-to-end framework. We first introduce modulation filters (M-Filters) to recover the unbinarized filters, which leads to a new architecture to calculate the network model. The convolution operation is further approximated by considering intra-class compactness in the loss function. As a result, our MCNs can reduce the size of required storage space of convolutional filters by a factor of 32, in contrast to the full-precision model, while achieving much better performances than state-of-the-art binarized models. Most importantly, MCNs achieve a comparable performance to the full-precision Resnets and WideResnets. The code will be available publicly soon.
更多查看译文
关键词
modulated convolutional networks,CNNs,computer vision tasks,storage requirement,computationally limited devices,MCNs,binarized filters,loss function,filter loss,center loss,softmax loss,end-to-end framework,modulation filters,unbinarized filters,network model,convolution operation,convolutional filters,deep networks,convolutional neural networks,storage space
AI 理解论文
溯源树
样例
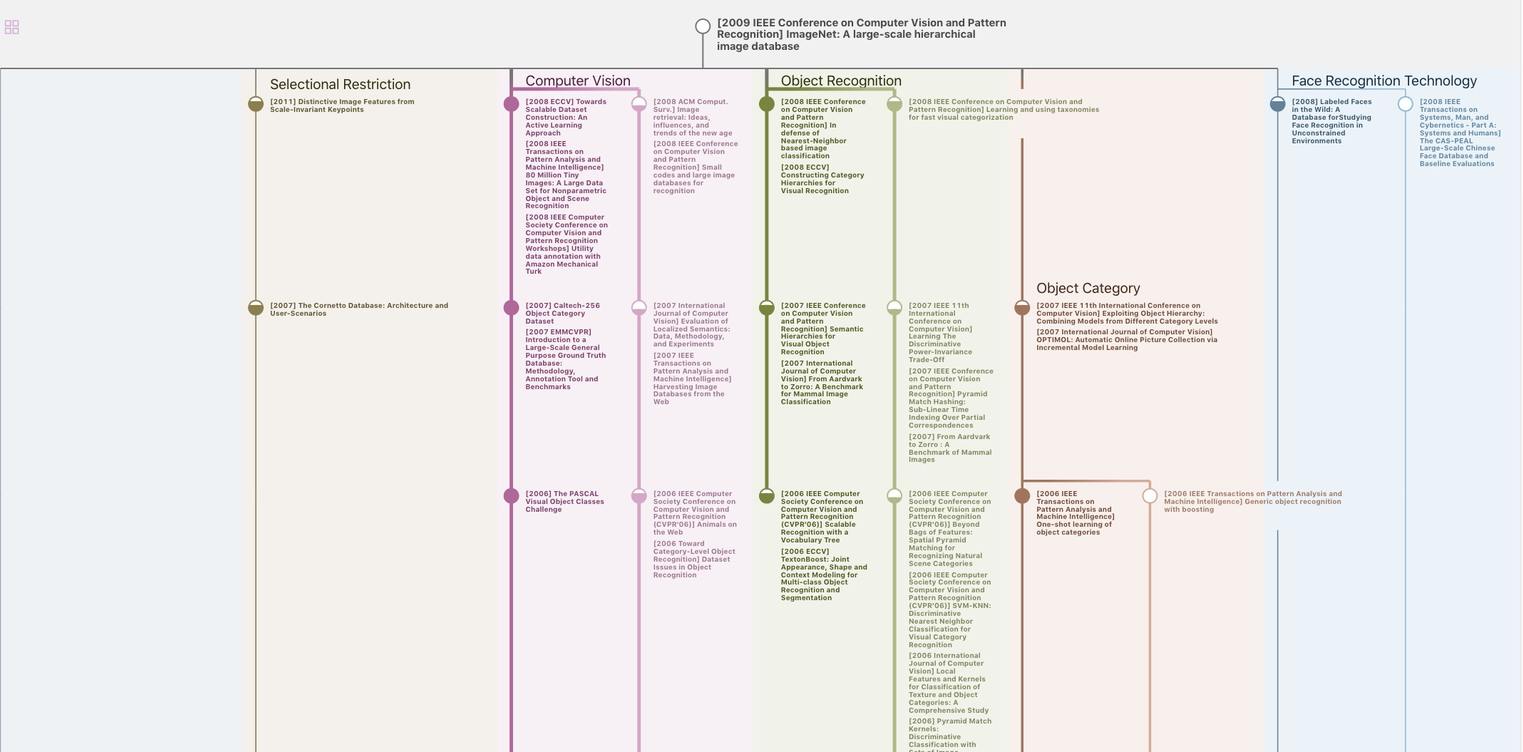
生成溯源树,研究论文发展脉络
Chat Paper
正在生成论文摘要