Hierarchical Recurrent Attention Networks for Structured Online Maps
2018 IEEE/CVF CONFERENCE ON COMPUTER VISION AND PATTERN RECOGNITION (CVPR)(2020)
摘要
In this paper, we tackle the problem of online road network extraction from sparse 3D point clouds. Our method is inspired by how an annotator builds a lane graph, by first identifying how many lanes there are and then drawing each one in turn. We develop a hierarchical recurrent network that attends to initial regions of a lane boundary and traces them out completely by outputting a structured polyline. We also propose a novel differentiable loss function that measures the deviation of the edges of the ground truth polylines and their predictions. This is more suitable than distances on vertices, as there exists many ways to draw equivalent polylines. We demonstrate the effectiveness of our method on a 90 km stretch of highway, and show that we can recover the right topology 92\% of the time.
更多查看译文
关键词
hierarchical recurrent attention networks,structured online,online road network extraction,sparse 3D point clouds,annotator,lane graph,hierarchical recurrent network,initial regions,lane boundary,structured poly-line,differentiable loss function,ground truth polylines,equivalent polylines
AI 理解论文
溯源树
样例
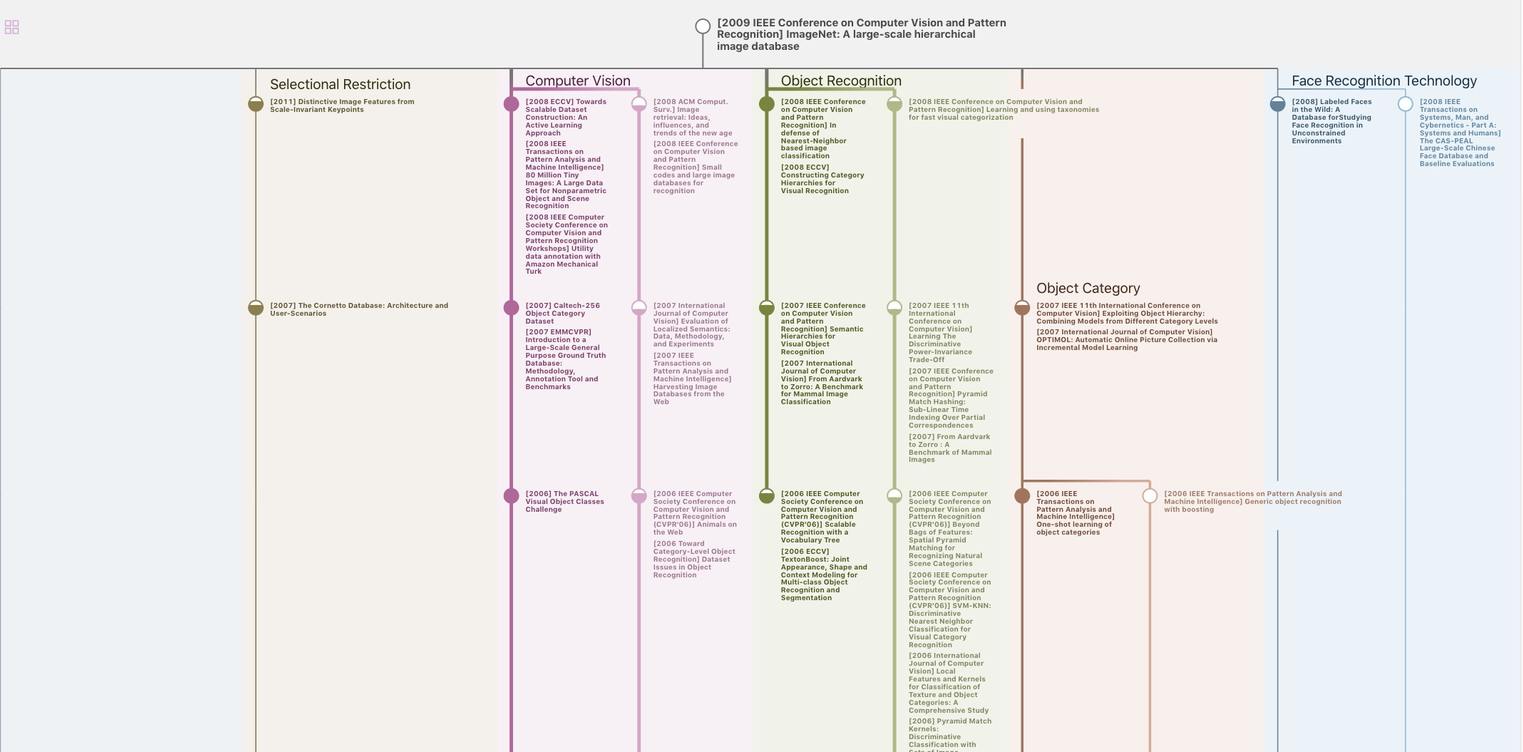
生成溯源树,研究论文发展脉络
Chat Paper
正在生成论文摘要