Denseaspp For Semantic Segmentation In Street Scenes
2018 IEEE/CVF CONFERENCE ON COMPUTER VISION AND PATTERN RECOGNITION (CVPR)(2018)
摘要
Semantic image segmentation is a basic street scene understanding task in autonomous driving, where each pixel in a high resolution image is categorized into a set of semantic labels. Unlike other scenarios, objects in autonomous driving scene exhibit very large scale changes, which poses great challenges for high-level feature representation in a sense that multi-scale information must be correctly encoded. To remedy this problem, atrous convolution[14] was introduced to generate features with larger receptive fields without sacrificing spatial resolution. Built upon atrous convolution, Atrous Spatial Pyramid Pooling (ASPP)[2] was proposed to concatenate multiple atrous-convolved features using different dilation rates into a final feature representation. Although ASPP is able to generate multi-scale features, we argue the feature resolution in the scale-axis is not dense enough for the autonomous driving scenario. To this end, we propose Densely connected Atrous Spatial Pyramid Pooling (DenseASPP), which connects a set of atrous convolutional layers in a dense way, such that it generates multi-scale features that not only cover a larger scale range, but also cover that scale range densely, without significantly increasing the model size. We evaluate DenseASPP on the street scene benchmark Cityscapes[4] and achieve state-of-the-art performance.
更多查看译文
关键词
spatial resolution,Atrous Spatial Pyramid Pooling,atrous-convolved features,final feature representation,multiscale features,feature resolution,scale-axis,autonomous driving scenario,DenseASPP,atrous convolutional layers,street scene benchmark Cityscapes,semantic image segmentation,high resolution image,semantic labels,autonomous driving scene,high-level feature representation,multiscale information,receptive fields,basic street scene understanding task,Densely connected Atrous Spatial Pyramid Pooling
AI 理解论文
溯源树
样例
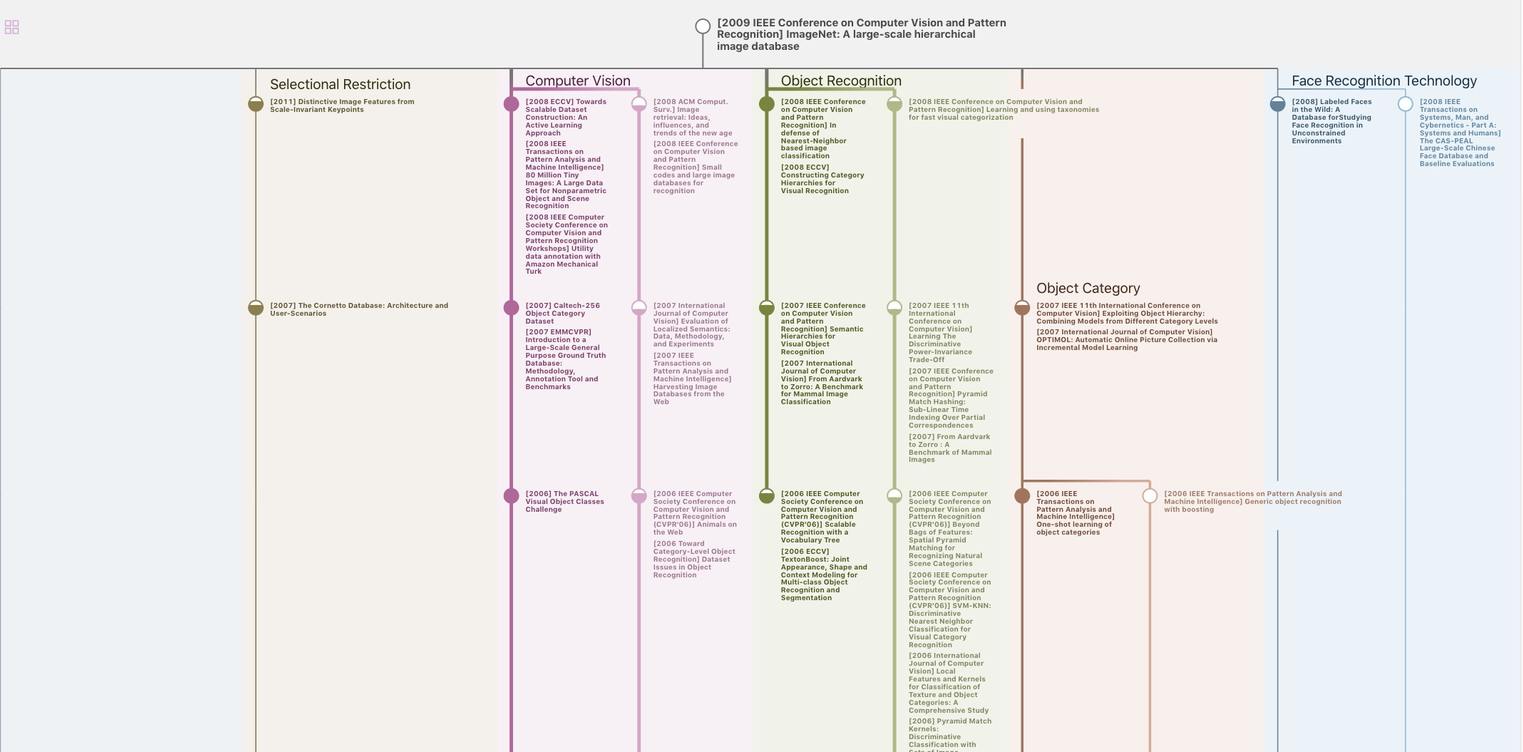
生成溯源树,研究论文发展脉络
Chat Paper
正在生成论文摘要