Interpolation-Based Object Detection Using Motion Vectors for Embedded Real-time Tracking Systems
2018 IEEE/CVF Conference on Computer Vision and Pattern Recognition Workshops (CVPRW)(2018)
摘要
Deep convolutional neural networks (CNNs) have achieved outstanding performance in object detection, a crucial task in computer vision. With the computational intensiveness due to repeated convolutions, they consume large amount of power, making them difficult to apply in power-constrained embedded platforms. In this work, we present MVint, a power-efficient detection and tracking framework. MVint combines motion-vector-based interpolator and CNN-based detector to simultaneously achieve high accuracy and energy efficiency by utilizing motion vectors obtained inexpensively in the environments wherein encoding is conducted at the cameras. Through evaluations using MOT16 benchmark that evaluates multiple object tracking, we show MVint maintains 88% MOTA while reducing detection frequency down to 1/12. An implemention of MVint as a system prototype on Xilinx Zynq Ultra-Scale+ MPSoC ZCU102 confirmed that MVint achieves an ideal 12x FPS compared with a vanilla detection approach.
更多查看译文
关键词
interpolation-based object detection,motion vectors,embedded real-time tracking systems,convolutional neural networks,computer vision,computational intensiveness,repeated convolutions,power-constrained embedded platforms,MVint,power-efficient detection,motion-vector-based interpolator,CNN-based detector,energy efficiency,MOT16 benchmark,multiple object tracking,detection frequency,vanilla detection approach
AI 理解论文
溯源树
样例
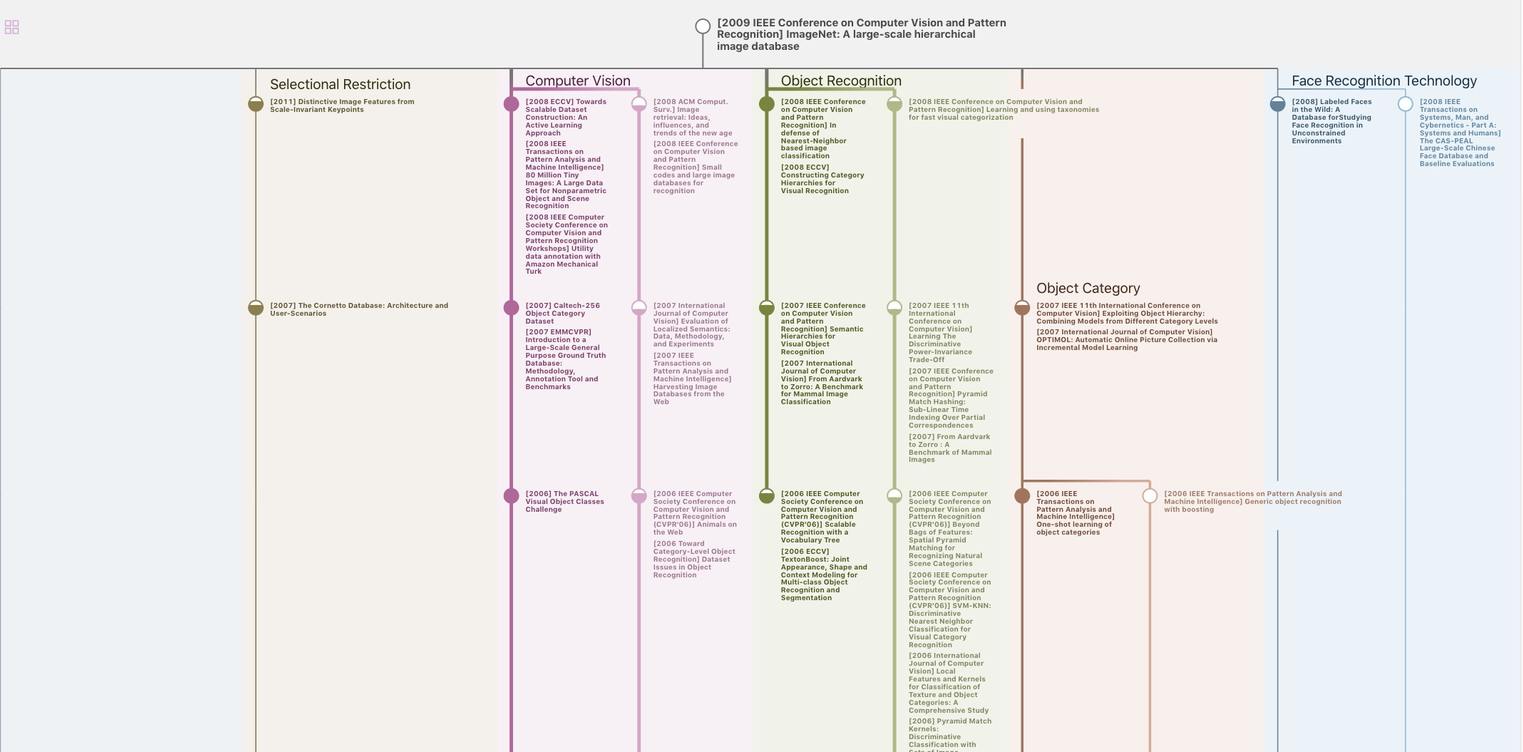
生成溯源树,研究论文发展脉络
Chat Paper
正在生成论文摘要