Deeply Learned Filter Response Functions For Hyperspectral Reconstruction
2018 IEEE/CVF CONFERENCE ON COMPUTER VISION AND PATTERN RECOGNITION (CVPR)(2018)
摘要
Hyperspectral reconstruction from RGB imaging has recently achieved significant progress via sparse coding and deep learning. However, a largely ignored fact is that existing RGB cameras are tuned to mimic human trichromatic perception, thus their spectral responses are not necessarily optimal for hyperspectral reconstruction. In this paper, rather than use RGB spectral responses, we simultaneously learn optimized camera spectral response functions (to be implemented in hardware) and a mapping for spectral reconstruction by using an end-to-end network. Our core idea is that since camera spectral filters act in effect like the convolution layer, their response functions could be optimized by training standard neural networks. We propose two types of designed filters: a three-chip setup without spatial mosaicing and a single-chip setup with a Bayer-style 2x2 filter array. Numerical simulations verify the advantages of deeply learned spectral responses compared to existing RGB cameras. More interestingly, by considering physical restrictions in the design process, we are able to realize the deeply learned spectral response functions by using modern film filter production technologies, and thus construct data-inspired multispectral cameras for snapshot hyperspectral imaging.
更多查看译文
关键词
hyperspectral reconstruction,RGB imaging,deep learning,human trichromatic perception,RGB spectral responses,spectral reconstruction,end-to-end network,camera spectral filters,Bayer-style 2x2 filter array,snapshot hyperspectral imaging,filter response functions,neural networks training,multispectral cameras,sparse coding,RGB cameras,camera spectral response functions optimization,film filter production technologies
AI 理解论文
溯源树
样例
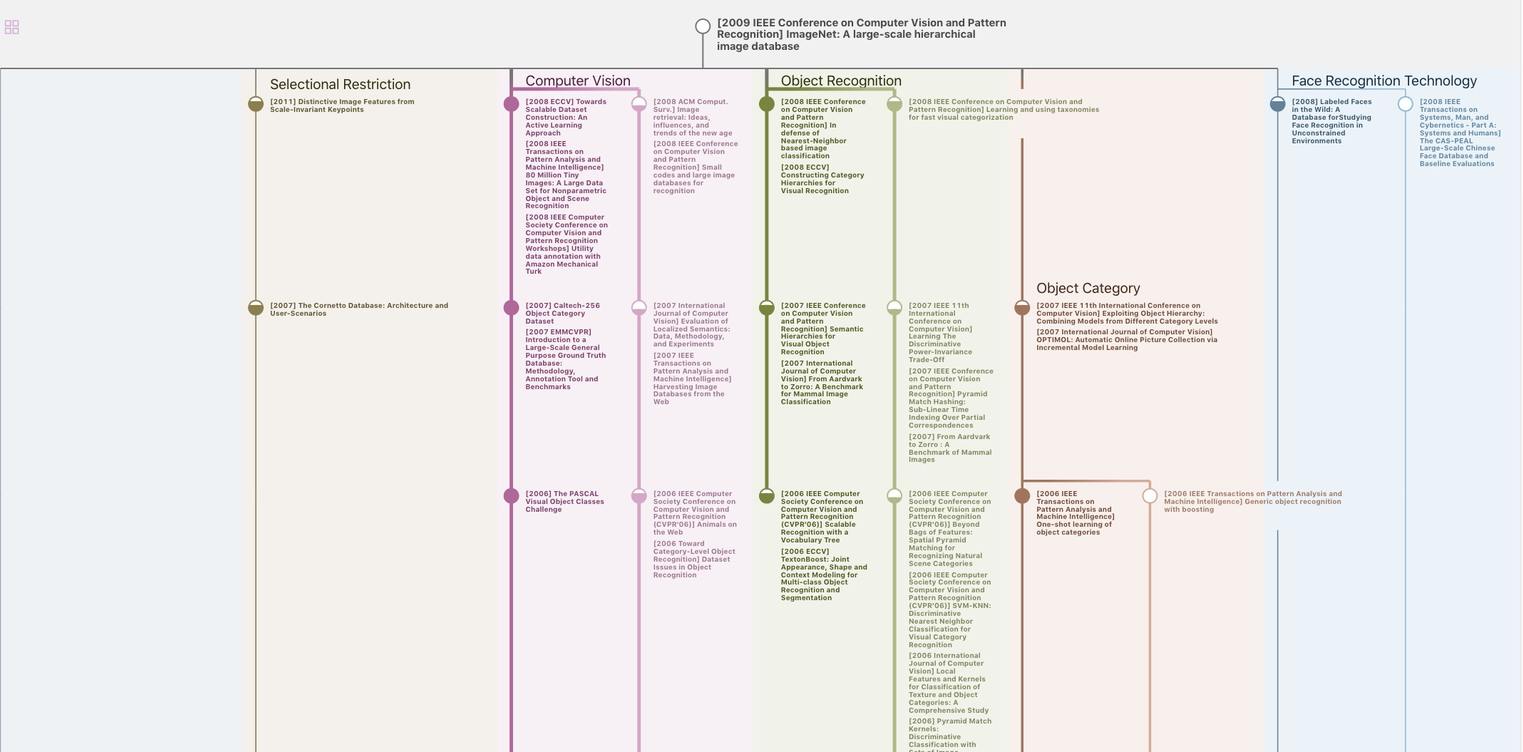
生成溯源树,研究论文发展脉络
Chat Paper
正在生成论文摘要