Detach and Adapt: Learning Cross-Domain Disentangled Deep Representation
2018 IEEE/CVF CONFERENCE ON COMPUTER VISION AND PATTERN RECOGNITION (CVPR)(2018)
摘要
While representation learning aims to derive interpretable features for describing visual data, representation disentanglement further results in such features so that particular image attributes can be identified and manipulated. However, one cannot easily address this task without observing ground truth annotation for the training data. To address this problem, we propose a novel deep learning model of Cross-Domain Representation Disentangler (CDRD). By observing fully annotated source-domain data and unlabeled target-domain data of interest, our model bridges the information across data domains and transfers the attribute information accordingly. Thus, cross-domain joint feature disentanglement and adaptation can be jointly performed. In the experiments, we provide qualitative results to verify our disentanglement capability. Moreover, we further confirm that our model can be applied for solving classification tasks of unsupervised domain adaptation, and performs favorably against state-of-the-art image disentanglement and translation methods.
更多查看译文
关键词
Cross-Domain Representation Disentangler,source-domain data,data domains,attribute information,cross-domain feature disentanglement,disentanglement capability,unsupervised domain adaptation,translation methods,deep Representation,representation learning,visual data,ground truth annotation,training data,deep learning model,image attributes,image disentanglement
AI 理解论文
溯源树
样例
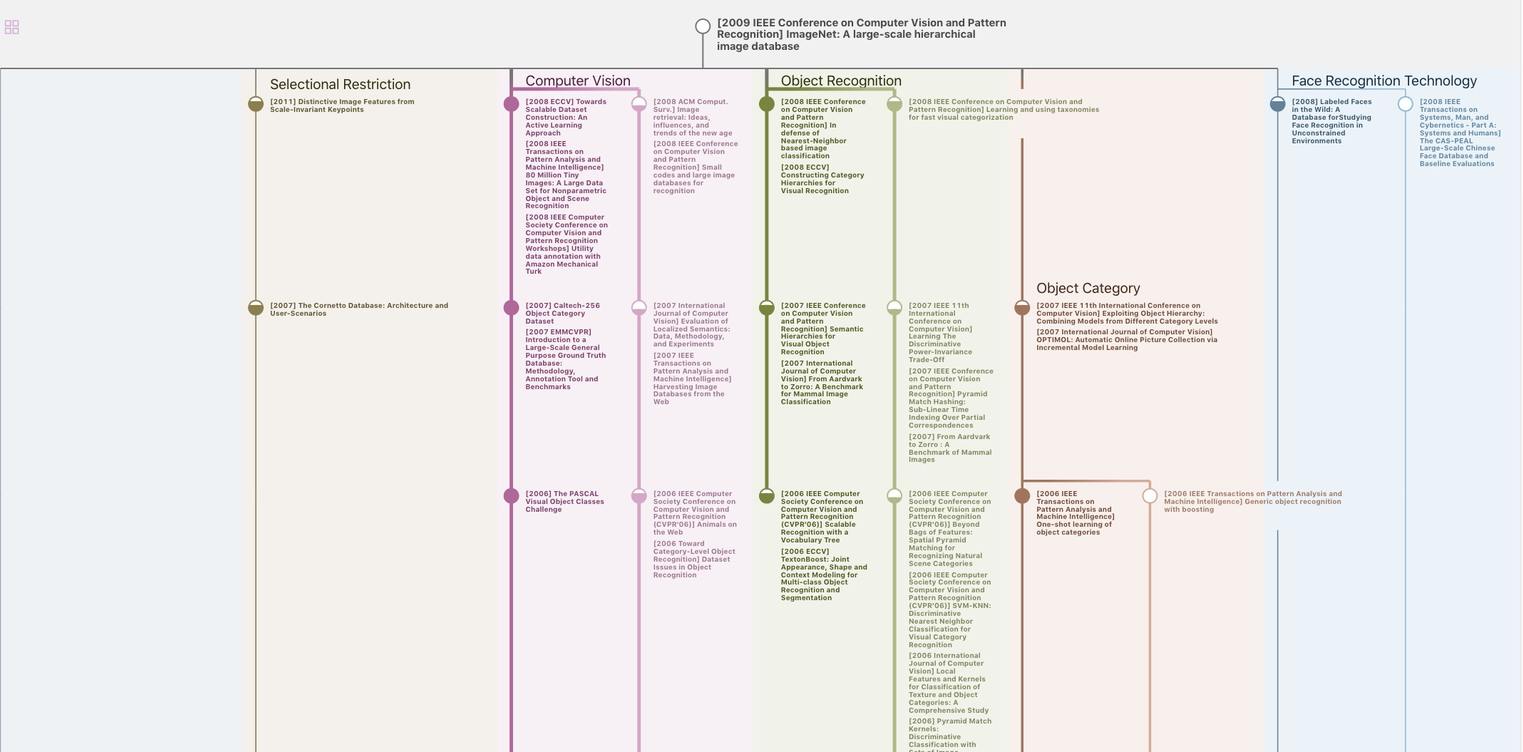
生成溯源树,研究论文发展脉络
Chat Paper
正在生成论文摘要