DETECTING INDUSTRIAL FOULING BY MONOTONICITY DURING ULTRASONIC CLEANING
2018 IEEE 28th International Workshop on Machine Learning for Signal Processing (MLSP)(2018)
摘要
High power ultrasound permits non-invasive cleaning of industrial equipment, but to make such cleaning systems energy efficient, one needs to recognize when the structure has been sufficiently cleaned without using invasive diagnostic tools. This can be done using ultrasound reflections generated inside the structure. This inverse modeling problem cannot be solved by forward modeling for irregular and complex structures, and it is difficult to tackle also with machine learning since human-annotated labels are hard get. We provide a deep learning solution that relies on the physical properties of the cleaning process. We rely on the fact that the amount of fouling is reduced as we clean more. Using this monotonicity property as indirect supervision we develop a semi-supervised model for detecting when the equipment has been cleaned.
更多查看译文
关键词
ultrasound,convolutional neural network,fouling detection,industrial cleaning
AI 理解论文
溯源树
样例
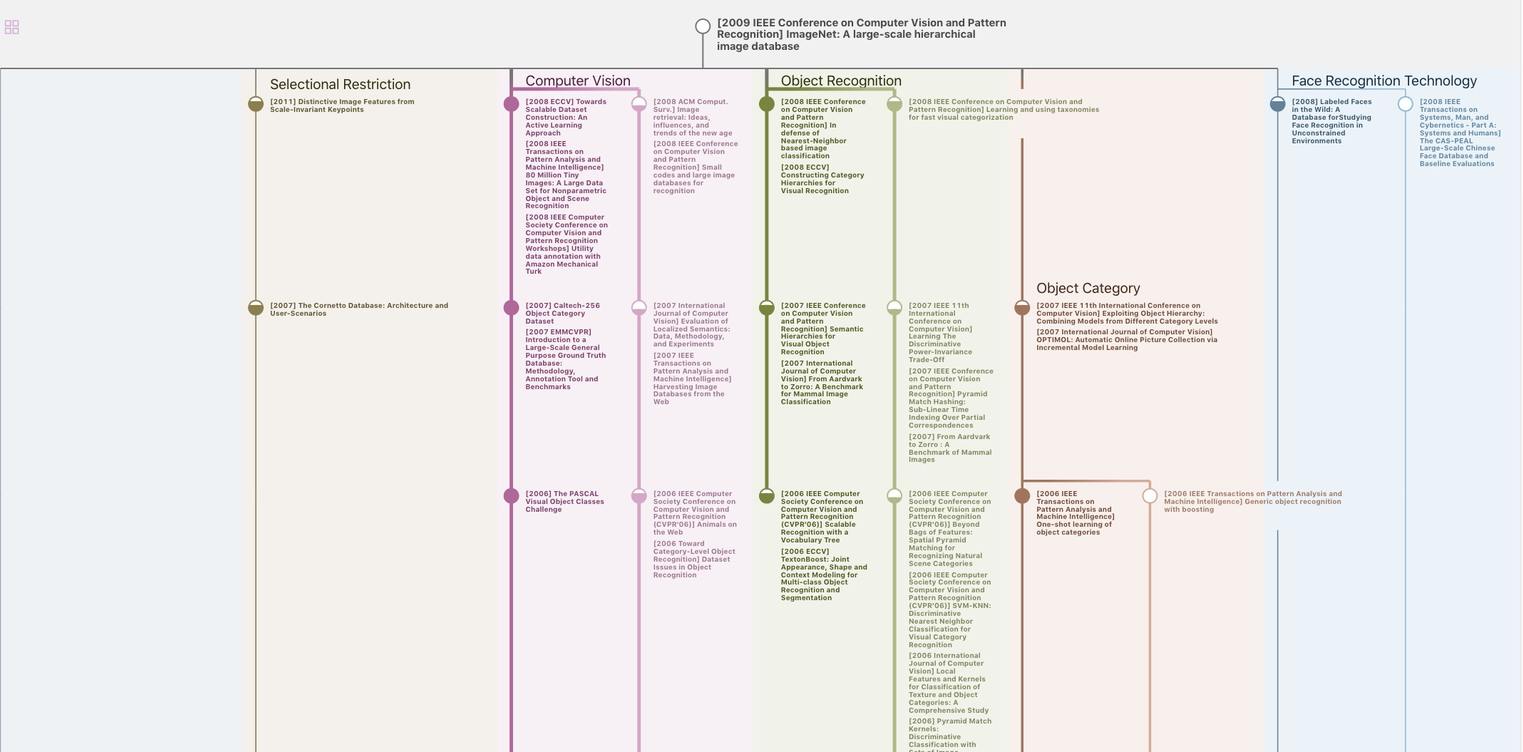
生成溯源树,研究论文发展脉络
Chat Paper
正在生成论文摘要