Improvements In Lte-Advanced Time Series Prediction With Dimensionality Reduction Algorithms
2018 IEEE 5G WORLD FORUM (5GWF)(2018)
摘要
Power consumption is a key challenge for LTE Advanced or future 5G mobile devices. Prediction of control channel signaling messages during an active connection with the network is a promising technique to improve the energy performance of LTE-A mobile devices and will also apply to future 5G devices due to the similarities between LTE-A and 5G New Radio (NR) standards in scheduling and controlling data transmissions. To reduce the prediction's computational complexity and thus, the power consumed by the predictor itself, various dimensionality reduction algorithms are evaluated in this paper. Specific windowing and normalization pre-processing steps are proposed to support the heterogeneous binary and integer time series data of LTE control channel messages. Using a simple Feed Forward Neural Network (FFNN) predictor, four dimensionality reduction algorithms, Principal Component Analysis (PCA), Independent Component Analysis (ICA), Autoencoder (AE), and Deep AE, are compared with respect to the prediction accuracy. Experiments based on live network data show that PCA achieves the best performance and allows to successfully reduce LTE-A control channel time series data from 450 to 45 dimensions without degrading the prediction accuracy compared to a FFNN predictor without dimensionality reduction.
更多查看译文
关键词
Dimensionality reduction, PCA, AE, deep AE, ICA, LTE, prediction
AI 理解论文
溯源树
样例
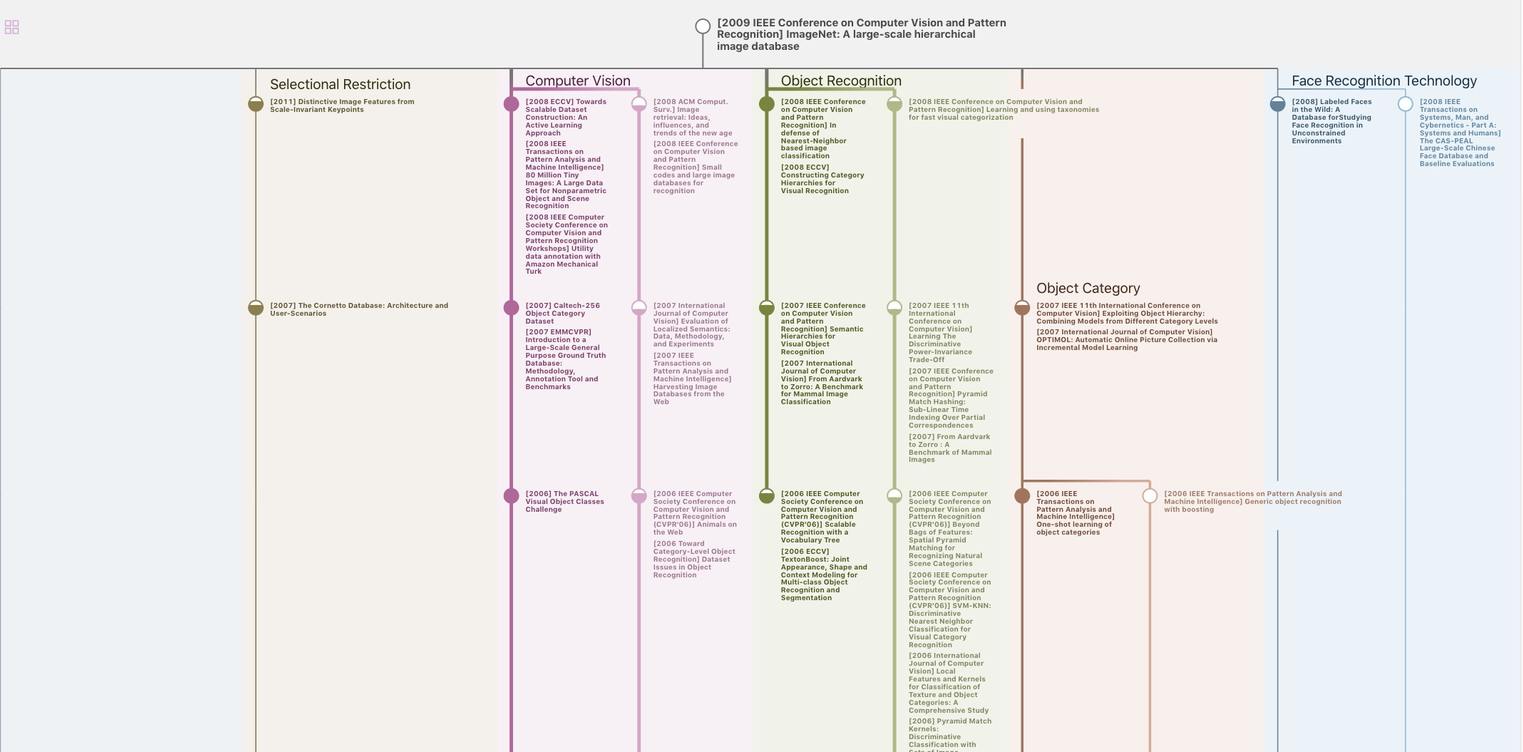
生成溯源树,研究论文发展脉络
Chat Paper
正在生成论文摘要