A Clustering-Based Deep Autoencoder For One-Class Image Classification
2017 IEEE LATIN AMERICAN CONFERENCE ON COMPUTATIONAL INTELLIGENCE (LA-CCI)(2017)
摘要
Anomaly detection in images is a topic of great interest in Computer Vision. It can be defined as an One-Class problem, where the goal is to detect deviations from known patterns, which are defined as normal. Recently, Deep Learning methods became popular due to their performance on classification tasks. This works presents an image anomaly detection classifier based on a previously known method, the Deep Embedded Clustering, which is based on a Deep Autoencoder. We show the effectiveness of the method through three different experiments. Results suggest that the method improves classification performance when compared to a Stacked Denoising Autoencoder in the image anomaly detection context.
更多查看译文
关键词
clustering-based deep autoencoder,deviation detection,deep learning methods,Stacked Denoising Autoencoder,classification performance,Deep Embedded Clustering,image anomaly detection classifier,classification tasks,One-Class problem,Computer Vision,one-class image classification
AI 理解论文
溯源树
样例
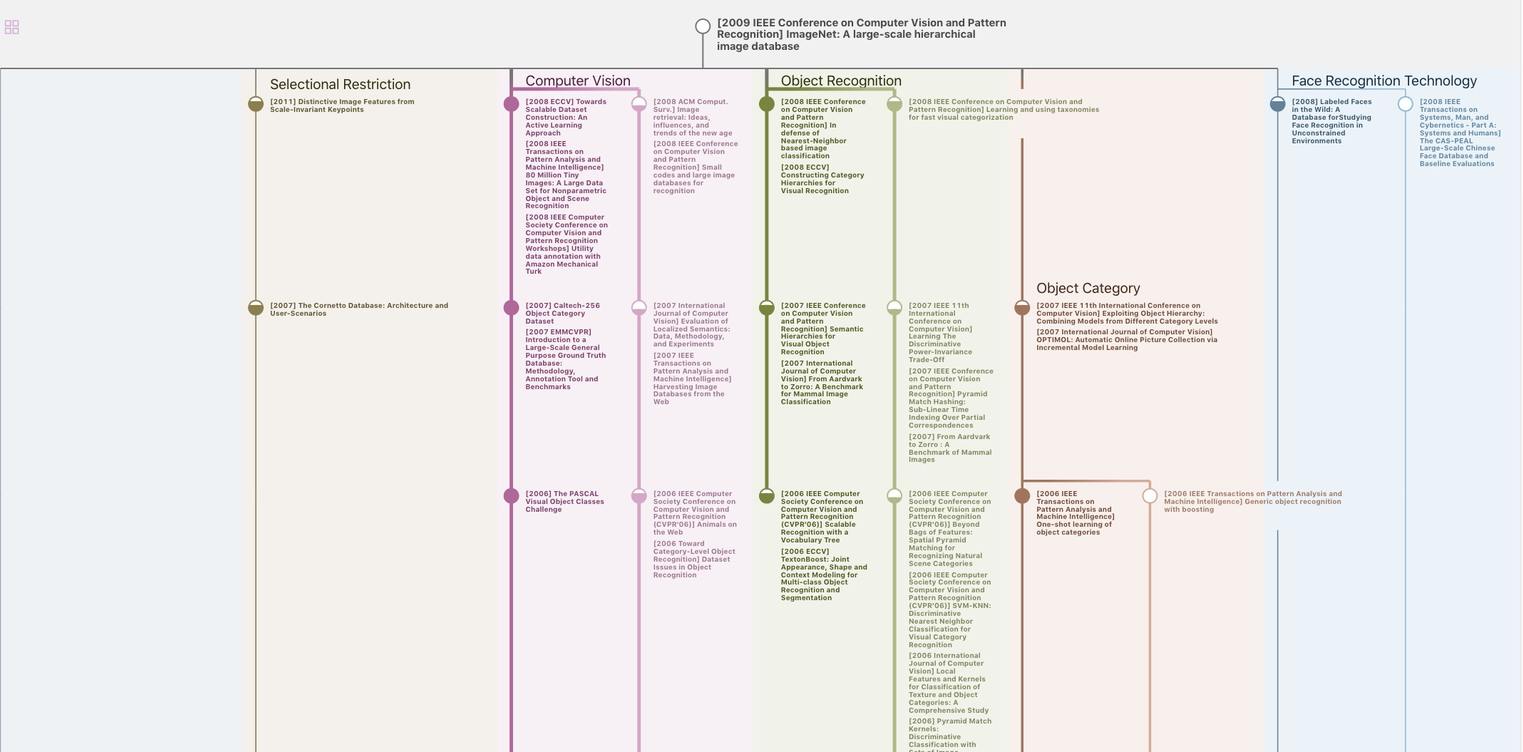
生成溯源树,研究论文发展脉络
Chat Paper
正在生成论文摘要