A Deep Bidirectional Long Short-Term Memory Approach Applied To The Protein Secondary Structure Prediction Problem
2017 IEEE LATIN AMERICAN CONFERENCE ON COMPUTATIONAL INTELLIGENCE (LA-CCI)(2017)
摘要
One of the most important open problems in science is the protein secondary structures prediction from the protein sequence of amino acids. This work presents an application of Deep Recurrent Neural Network with Bidirectional Long Short-Term Memory (DBLSTM) cells to this problem. We compare the performance of the proposed approach with the state-of-the-art approaches. Despite the lower complexity of the proposed approach (i.e. Neural Network architecture with fewer neurons), results showed that the DBLSTM could achieve a satisfactory level of accuracy when compared with the state-of-the-art approaches. We also studied the behavior of Gradient Optimizers applied to the DBLSTM. Furthermore, this paper concentrates on well-known quantitative analytical methods applied to evaluate the proposed approach.
更多查看译文
关键词
neural network architecture,deep bidirectional long short-term memory approach,Deep Recurrent Neural Network with Bidirectional Long Short-Term Memory cells,DBLSTM cells,gradient optimizers,amino acids,protein sequence,protein secondary structures prediction,protein secondary structure prediction problem
AI 理解论文
溯源树
样例
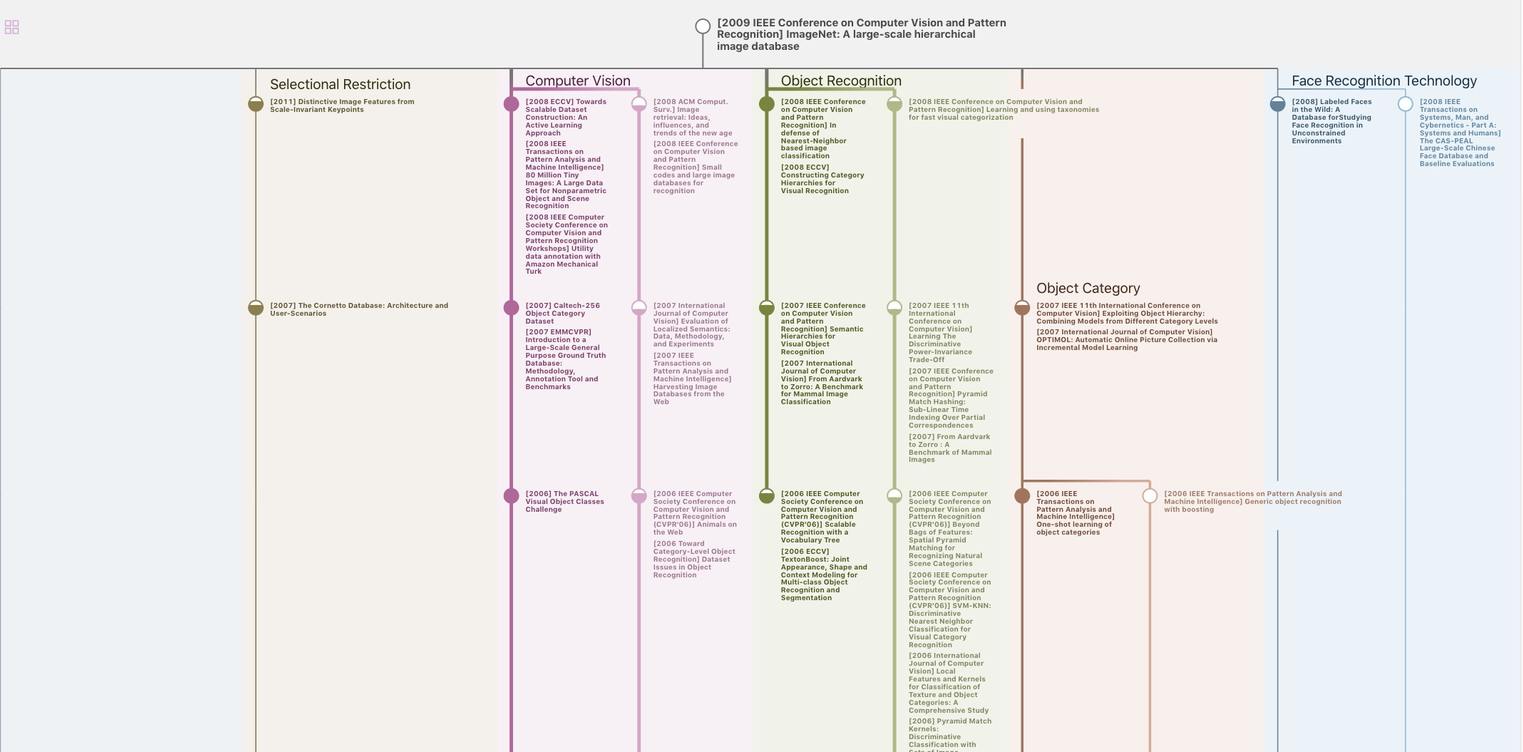
生成溯源树,研究论文发展脉络
Chat Paper
正在生成论文摘要