Renewable Energy Integration: Bayesian Networks for Probabilistic State Estimation.
DARE@PKDD/ECML(2018)
摘要
Increased availability of renewable energy sources, along with novel techniques for power flow control, open up a broad range of interesting challenges and opportunities in power flow optimization. This promises reduced power generation costs through better integration of renewable energy generators into the Smart Grid. Unfortunately, renewable generators are fundamentally variable and uncertain. This uncertainty motivates our study of probabilistic state estimation techniques in this paper. Specifically, we use probabilistic graphical models in the form of Bayesian networks to compute probabilities of power system states, thus enabling improved power flow control. Key differences between our probabilistic state estimation results as reported in this paper and similar previous efforts include: our use of Bayesian probabilistic but exact (rather than Monte Carlo) state estimation techniques; auto-generation of Bayesian networks for probabilistic state estimation; integration with corrective Security-Constrained Optimal Power Flow; and application to Distributed Flexible AC Transmission Systems. We present novel models and algorithms for probabilistic state estimation using auto-generated Bayesian networks compiled to junction trees, and report on experimental results that illustrate the scalability of our methods.
更多查看译文
关键词
Renewable energy, Optimal power flow, Power flow control, Smart wires, Transmission grid, Probabilistic state estimation, Bayesian networks, Junction trees, Exact inference
AI 理解论文
溯源树
样例
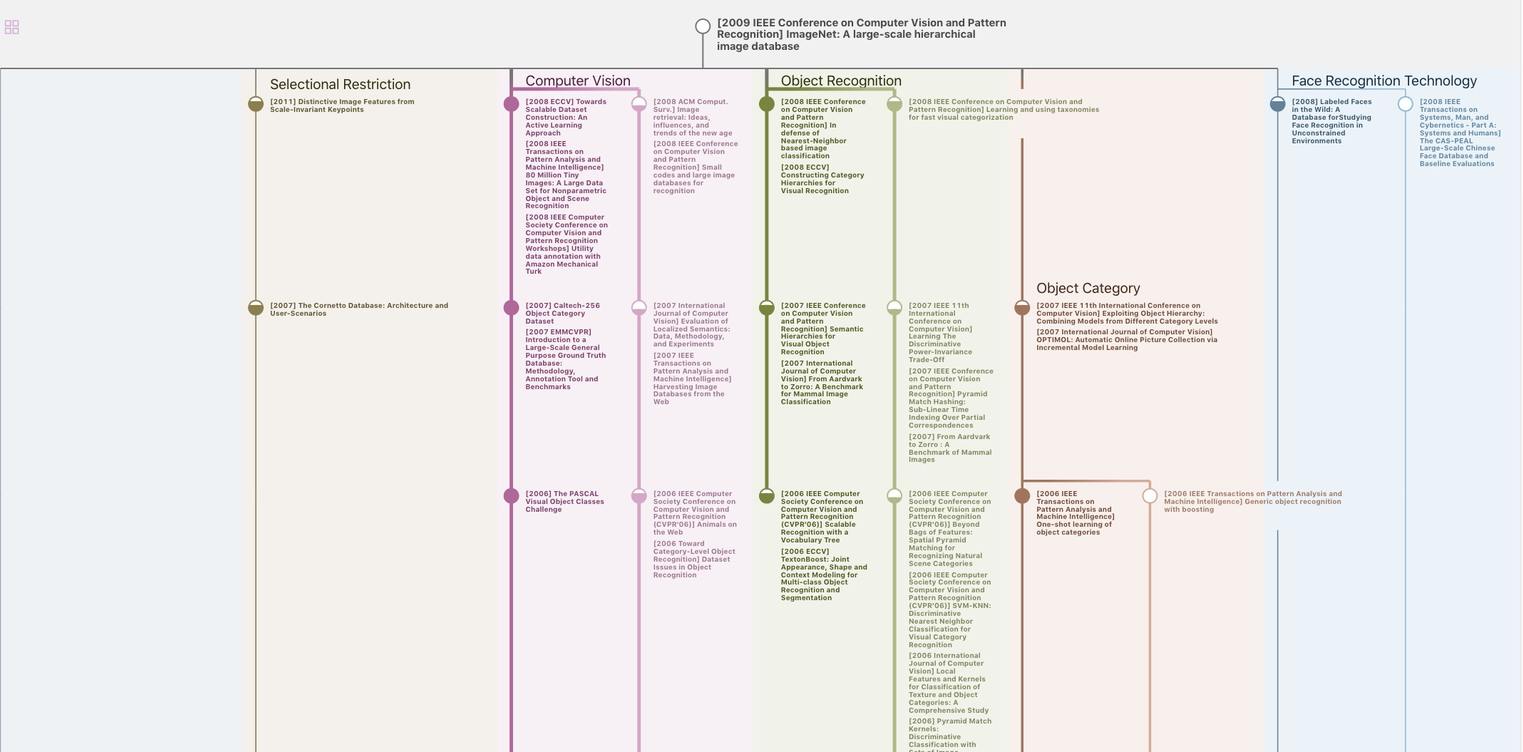
生成溯源树,研究论文发展脉络
Chat Paper
正在生成论文摘要