Comparing Manual and Machine Annotations of Emotions in Non-acted Speech.
EMBC(2018)
摘要
Psychological well-being at the workplace has increased the demand for detecting emotions with higher accuracies. Speech, one of the most non-obtrusive modes of capturing emotions at the workplace, is still in need of robust emotion annotation mechanisms for non-acted speech corpora. In this paper, we extend our experiments on our non-acted speech database in two ways. First, we report how participants themselves perceive the emotion in their voice after a long gap of about six months, and how a third person, who has not heard the clips earlier, perceives the emotion in the same utterances. Both annotators also rated the intensity of the emotion. They agreed better in neutral (84%) and negative clips (74%) than in positive ones (38%). Second, we restrict our attention to those samples that had agreement and show that the classification accuracy of 80% by machine learning, an improvement of 7% over the state-of-the-art results for speakerdependent classification. This result suggests that the high-level perception of emotion does translate to the low-level features of speech. Further analysis shows that the silently expressed positive and negative emotions are often misinterpreted as neutral. For the speaker-independent test set, we report an overall accuracy of 61%.
更多查看译文
关键词
Emotions,Humans,Machine Learning,Speech,Speech Perception,Voice
AI 理解论文
溯源树
样例
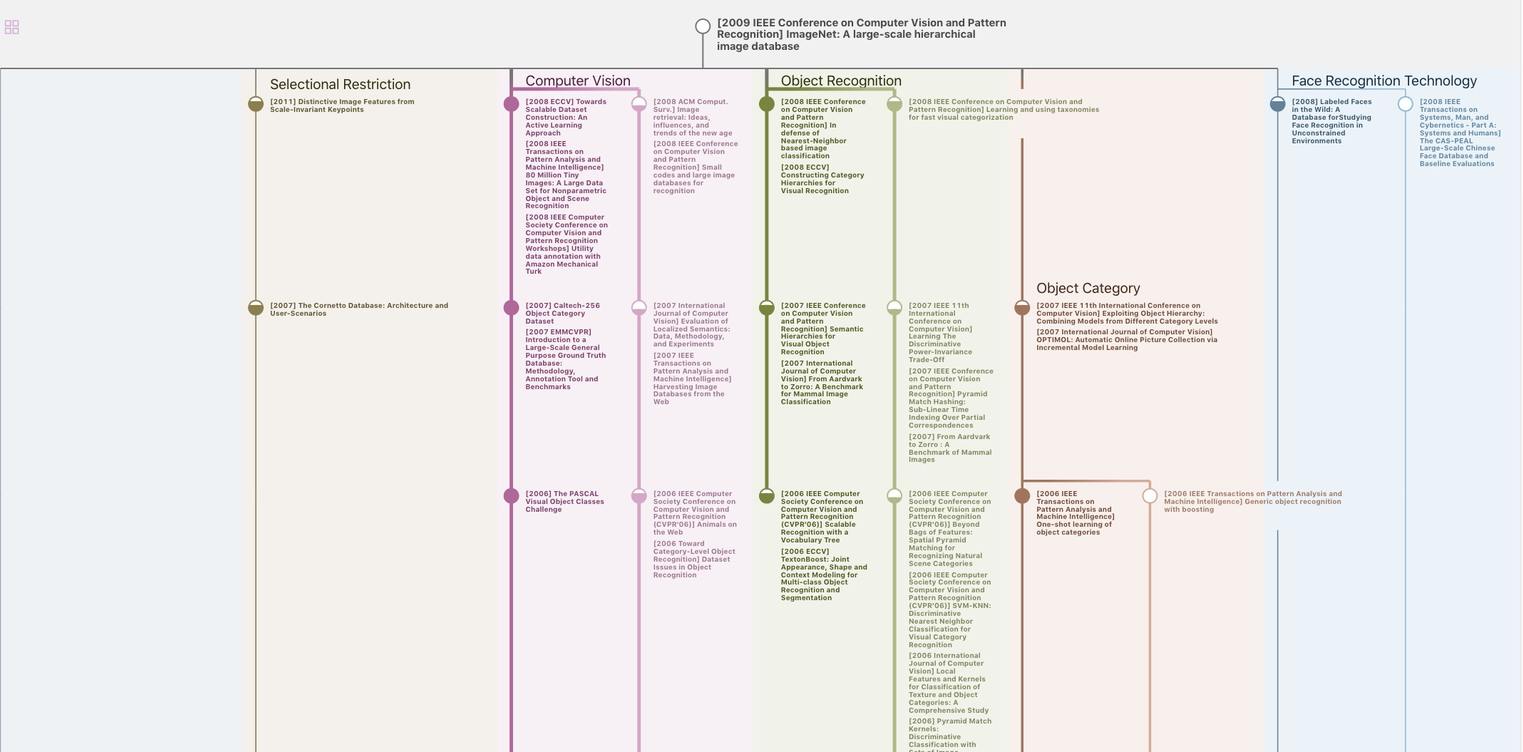
生成溯源树,研究论文发展脉络
Chat Paper
正在生成论文摘要