Learning Label-Specific Features For Multi-Label Classification With Missing Labels
2018 IEEE FOURTH INTERNATIONAL CONFERENCE ON MULTIMEDIA BIG DATA (BIGMM)(2018)
摘要
Existing multi-label learning approaches mainly assume that all the class labels are observed for all the training examples, and utilize an identical data representation composed of all the features in the discrimination of all the labels. However, in multi-label learning, each class label might be determined by some specific features of its own, and only a partial label set for each example can be obtained in many applications. This paper propose a new method to learn Label-Specific features for multi-label classification with Missing Labels, named LSML. First, we learn the label correlations which can be exploited to augment the incomplete label matrix and obtain a new supplementary label matrix. Then, we learn a label-specific data representation for each class label, which is composed of label-specific features for the corresponding class label, and build the multi-label classifier simultaneously. A comparative study with the state-of-the-art approaches manifests the effectiveness of our proposed method.
更多查看译文
关键词
label-specific features, missing labels, multi-label learning
AI 理解论文
溯源树
样例
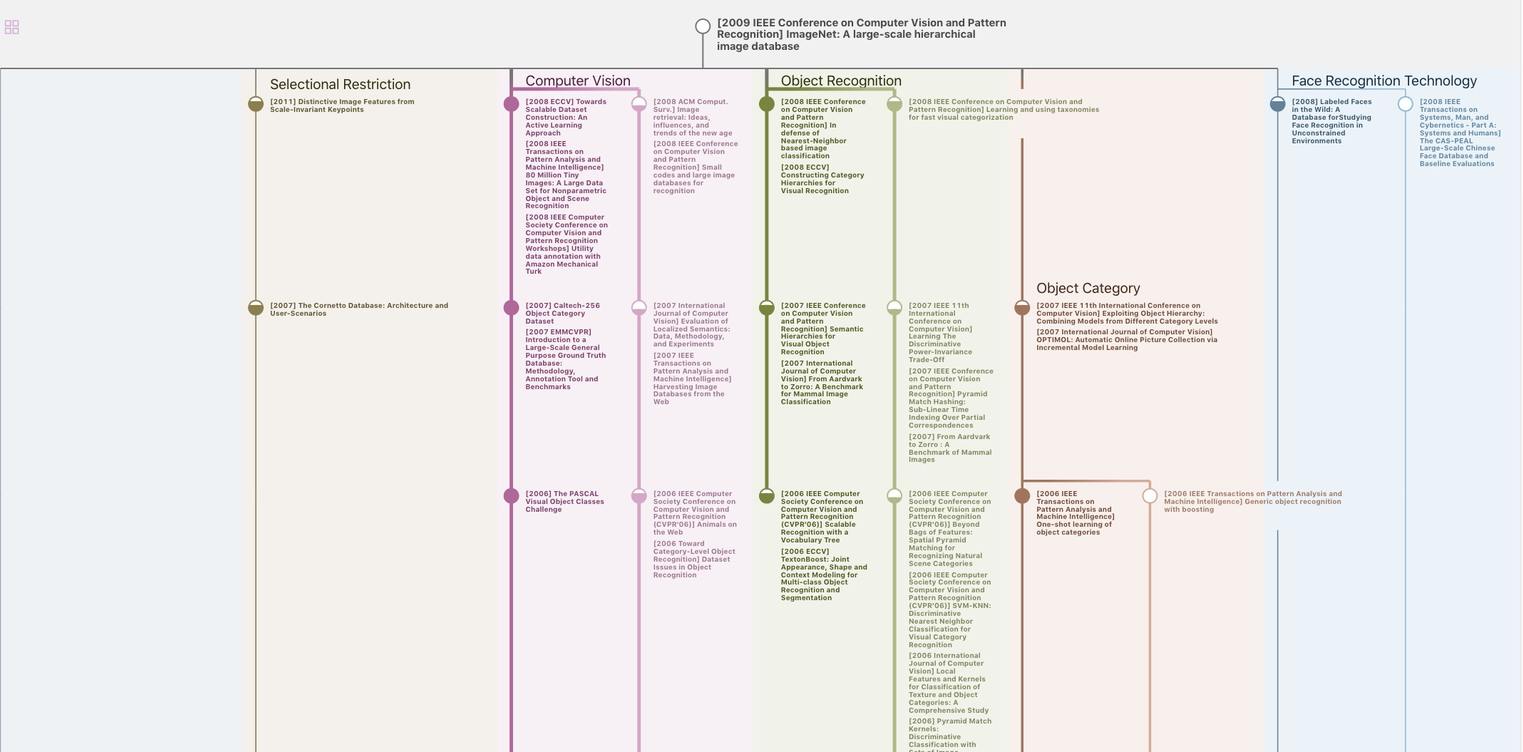
生成溯源树,研究论文发展脉络
Chat Paper
正在生成论文摘要