Understanding How Non-Experts Collect and Annotate Activity Data.
UbiComp '18: The 2018 ACM International Joint Conference on Pervasive and Ubiquitous Computing Singapore Singapore October, 2018(2018)
摘要
Training classifiers for human activity recognition systems often relies on large corpora of annotated sensor data. Crowd sourcing is one way to collect and annotate large amounts of sensor data. Crowd sourcing often depends on unskilled workers to collect and annotate the data. In this paper we explore machine learning of classifiers based on human activity data collected and annotated by non-experts. We consider the entire process starting from data collection through annotation including machine learning and ending with the final application implementation. We focus on three issues 1) can non-expert annotators overcome the technical challenges of data acquisition and annotation, 2) can they annotate reliably, and 3) to what extent might we expect their annotations to yield accurate and generalizable event classifiers. Our results suggest that non-expert users can collect video and data as well as produce annotations which are suitable for machine learning.
更多查看译文
关键词
data labeling, efficient data collection
AI 理解论文
溯源树
样例
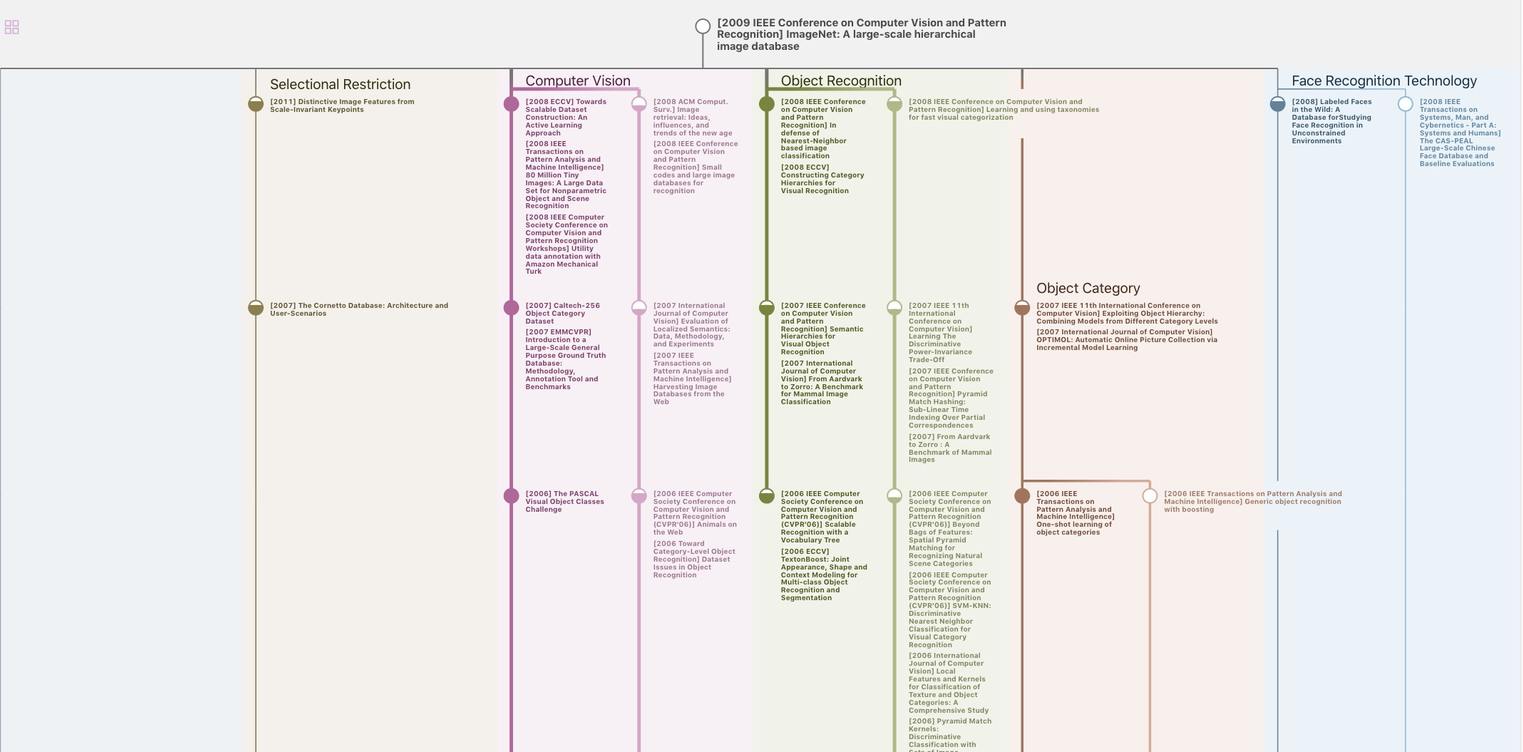
生成溯源树,研究论文发展脉络
Chat Paper
正在生成论文摘要