Inferring Types on Large Datasets Applying Ontology Class Hierarchy Classifiers: The DBpedia Case.
EKAW(2018)
摘要
Adding type information to resources belonging to large knowledge graphs is a challenging task, specially when considering those that are generated collaboratively, such as DBpedia, which usually contain errors and noise produced during the transformation process from different data sources. It is important to assign the correct type(s) to resources in order to efficiently exploit the information provided by the dataset. In this work we explore how machine learning classification models can be applied to solve this issue, relying on the information defined by the ontology class hierarchy. We have applied our approaches to DBpedia and compared to the state of the art, using a per-level analysis. We also define metrics to measure the quality of the results. Our results show that this approach is able to assign 56% more new types with higher precision and recall than the current DBpedia state of the art.
更多查看译文
关键词
DBpedia, Machine learning, Type prediction, Data quality, Linked data, Semantic web
AI 理解论文
溯源树
样例
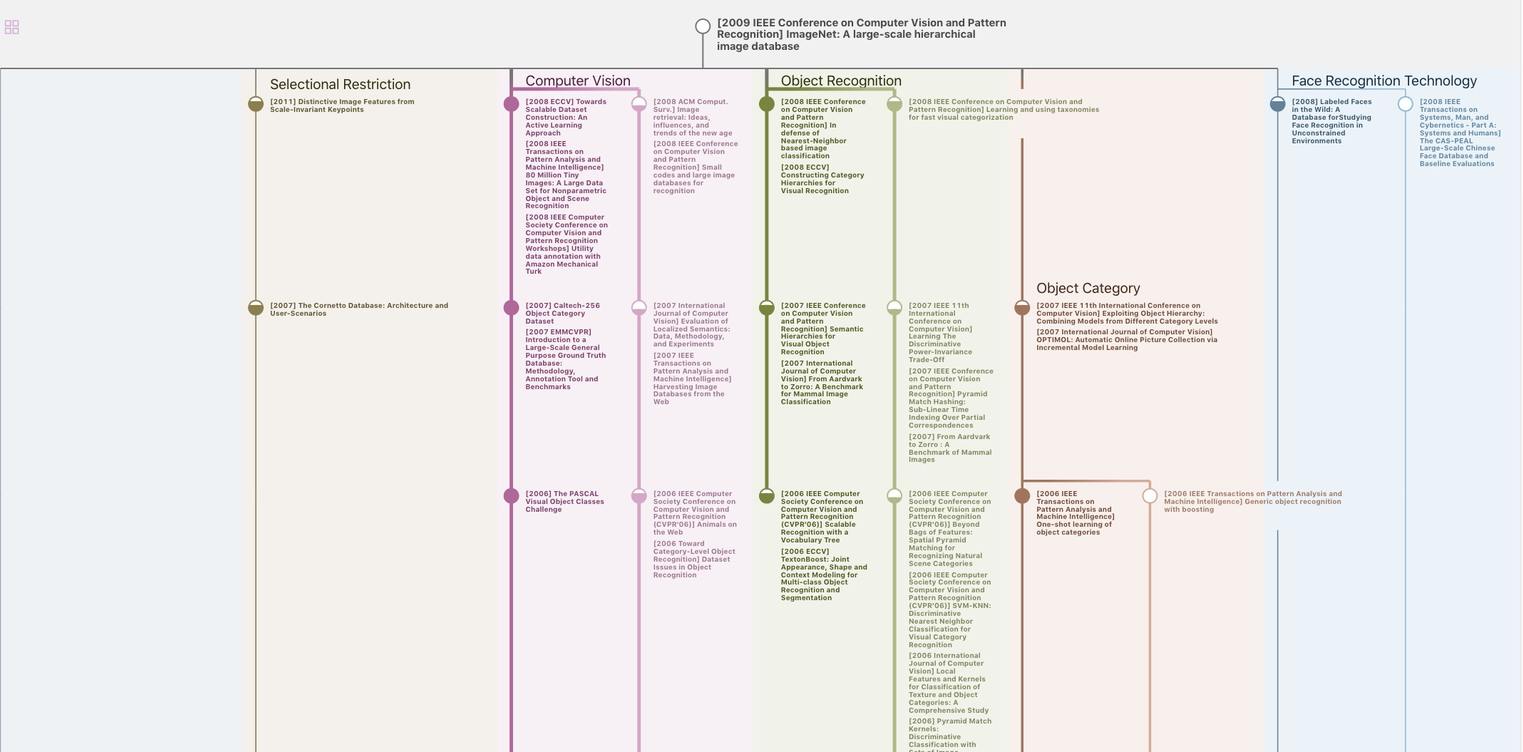
生成溯源树,研究论文发展脉络
Chat Paper
正在生成论文摘要