Building Detection And Segmentation Using A Cnn With Automatically Generated Training Data
IGARSS 2018 - 2018 IEEE INTERNATIONAL GEOSCIENCE AND REMOTE SENSING SYMPOSIUM(2018)
摘要
Significantly outperforming traditional machine learning methods, deep convolutional neural networks have gained increasing popularity in the application of image classification and segmentation. Nevertheless, deep learning-based methods usually require a large amount of training data, which is quite labor-intensive and time-demanding. To deal with the problem in generating training data, we propose in this paper a novel approach to generate image annotations by transferring labels from aerial images to UAV images and refine the annotations using a densely connected CRF model with an embedded naive Bayes classifier. The generated annotations not only present correct semantic labels, but also preserve accurate class boundaries. To validate the utility of these automatic annotations, we deploy them as training data for pixel-wise image segmentation and compare the results with the segmentation using manual annotations. Experiment results demonstrate that the automatic annotations can achieve comparable segmentation accuracy as the manual annotations.
更多查看译文
关键词
Image segmentation, Automatic image annotation, Label propagation, Deep learning
AI 理解论文
溯源树
样例
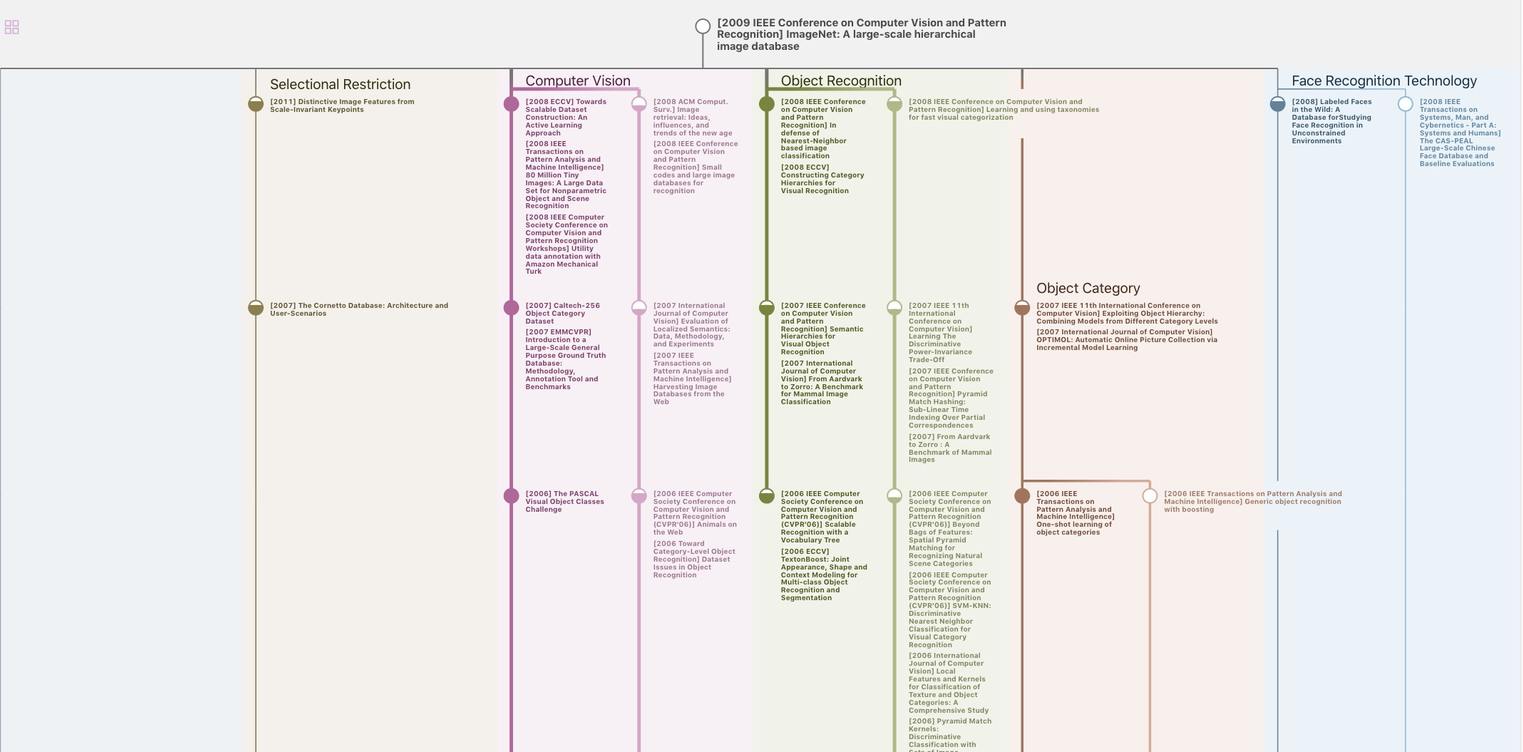
生成溯源树,研究论文发展脉络
Chat Paper
正在生成论文摘要