Spectral-Spatial Hyperspectral Image Classification Via Locality And Structure Constrained Low-Rank Representation
IGARSS 2018 - 2018 IEEE INTERNATIONAL GEOSCIENCE AND REMOTE SENSING SYMPOSIUM(2018)
摘要
Low-rank representation (LRR) has been applied widely in most fields due to its considerable ability to explore the low-dimensional subspace embedding in high-dimensional data. However, there are still some problems that LRR can't effectively exploit the local structure and the representation for the given data is not discriminative enough. To tackle the above issues, we propose a novel locality and structure constrained low-rank representation (LSLRR) for hyperspectral image (HSI) classification. First, a distance metrics, which combines spectral and spatial similarity, is proposed to constrain the local structure. This makes two pixels in HSI with small distance have high similarity. Second, we exploit the classwise block-diagonal structure for the training data to learn the more discriminative representation for the testing data. And the experimental results verify the effectiveness and superiority of LSLRR comparing with other state-of-the-art methods.
更多查看译文
关键词
hyperspectral image classification, low-rank representation, block-diagonal structure
AI 理解论文
溯源树
样例
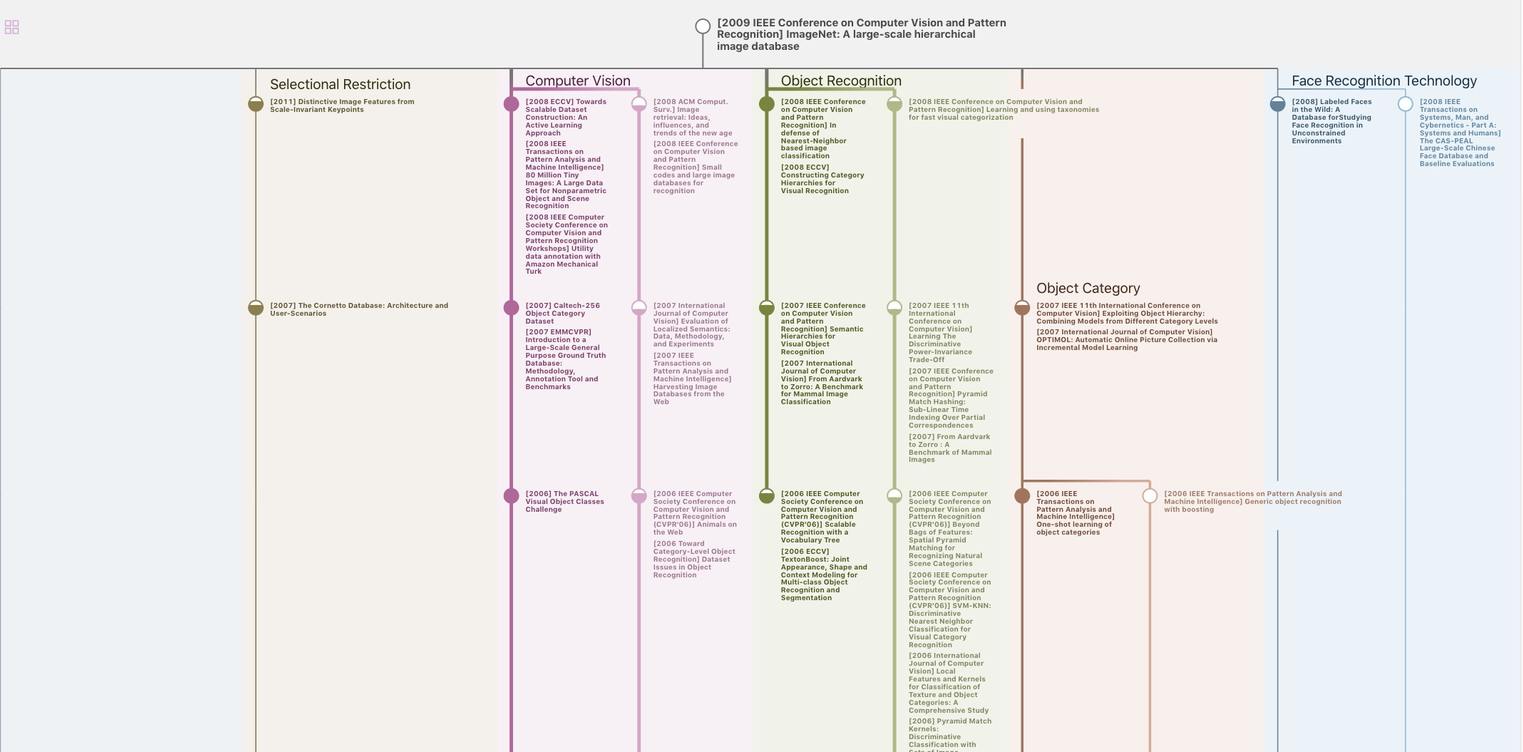
生成溯源树,研究论文发展脉络
Chat Paper
正在生成论文摘要