Patch-Based Residual Networks For Compressively Sensed Hyperspectral Images Restruction
IGARSS 2018 - 2018 IEEE INTERNATIONAL GEOSCIENCE AND REMOTE SENSING SYMPOSIUM(2018)
摘要
Most traditional compressive sensing (CS) reconstruction methods suffer from the intensive computation caused by iterations. This paper aims at presenting a non-iterative algorithm to reconstruct hyperspectral images (HSI) from patch-based compressively sensed measurements. Our method contains two residual convolutional neural networks. One is reconstruction network for compressive sensing reconstruction and the other is deblocking network for removing the blocky effect, which is caused by patch-based sampling. The reconstruction network can efficiently reconstruct all the bands of HSI jointly, thus the spectral correlation is well preserved. In addition, the deblock performance is enhanced by combining more patches into a larger patch in the deblocking network. Experimental results verify that our method outperforms the state-of-the-art compressive sensing reconstruction methods with patch-based CS measurement.
更多查看译文
关键词
residual network, compressive sensing, image deblocking, hyperspectral images
AI 理解论文
溯源树
样例
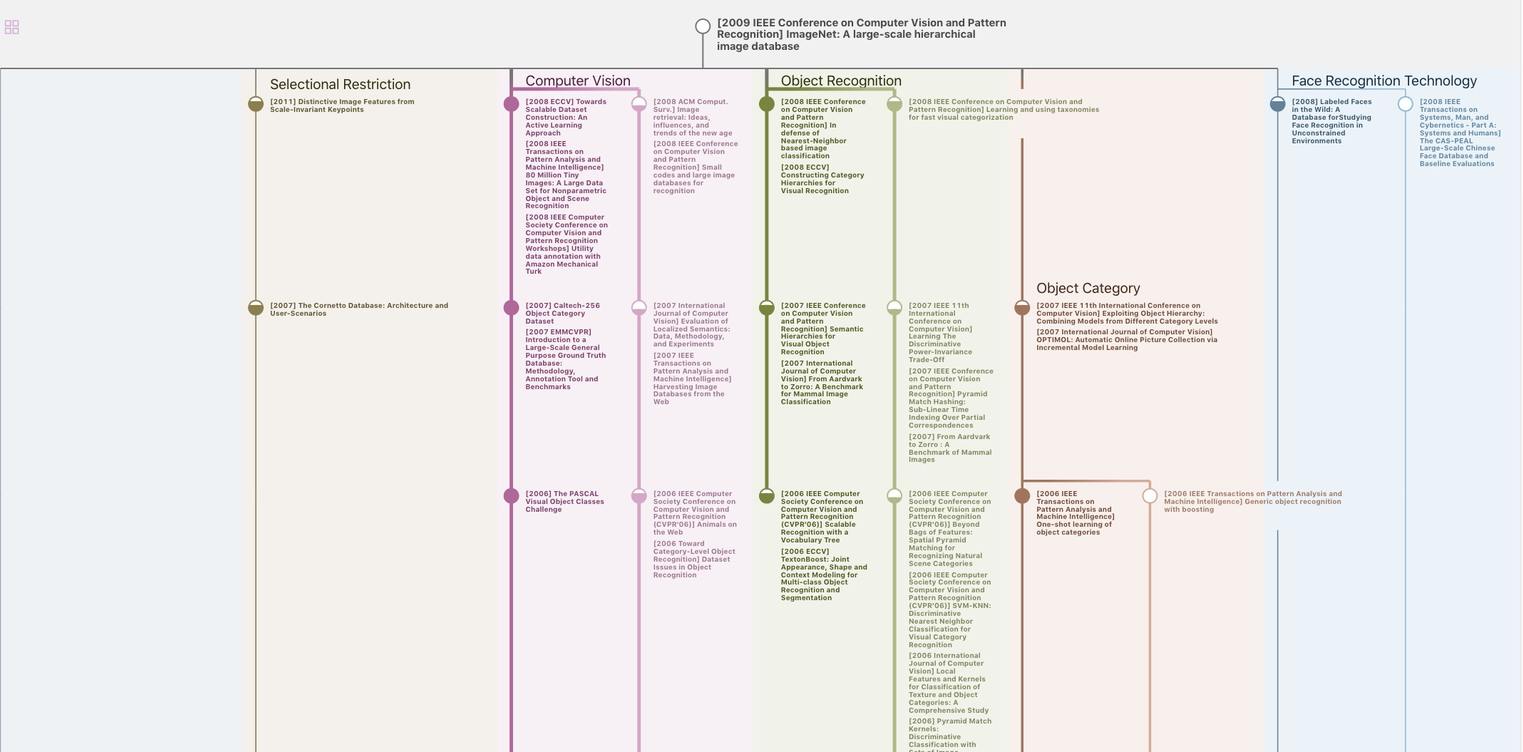
生成溯源树,研究论文发展脉络
Chat Paper
正在生成论文摘要