Spatially Regularzied Sparsecem For Target Detection In Hyperspectral Images
IGARSS 2018 - 2018 IEEE INTERNATIONAL GEOSCIENCE AND REMOTE SENSING SYMPOSIUM(2018)
摘要
Constrained energy minimization (CEM) is a popular method for target detection in hyperspectral images. Its variant SparseCEM uses a sparsity regularization term to promote the sparsity of the detection output. However, these approaches do not consider the spatial correlation of hyperspectral pixels, and target detection can further benefit from exploiting the spatial information. In this paper, we propose a novel constrained detection algorithm, referred to as Spatial-SparseCEM, to simultaneously force the sparsity of the output and piecewise continuity via proper regularizations. The formulated problem is solved efficiently by using alternating direction method of multipliers (ADMM). We illustrate the enhanced performance of the Spatial-SparseCEM algorithm via both synthetic and real hyperspectral data.
更多查看译文
关键词
Hyperspectral image, target detection, l(1)-norm regularization, spatially-regularized detection, constrained energy minimization, ADMM
AI 理解论文
溯源树
样例
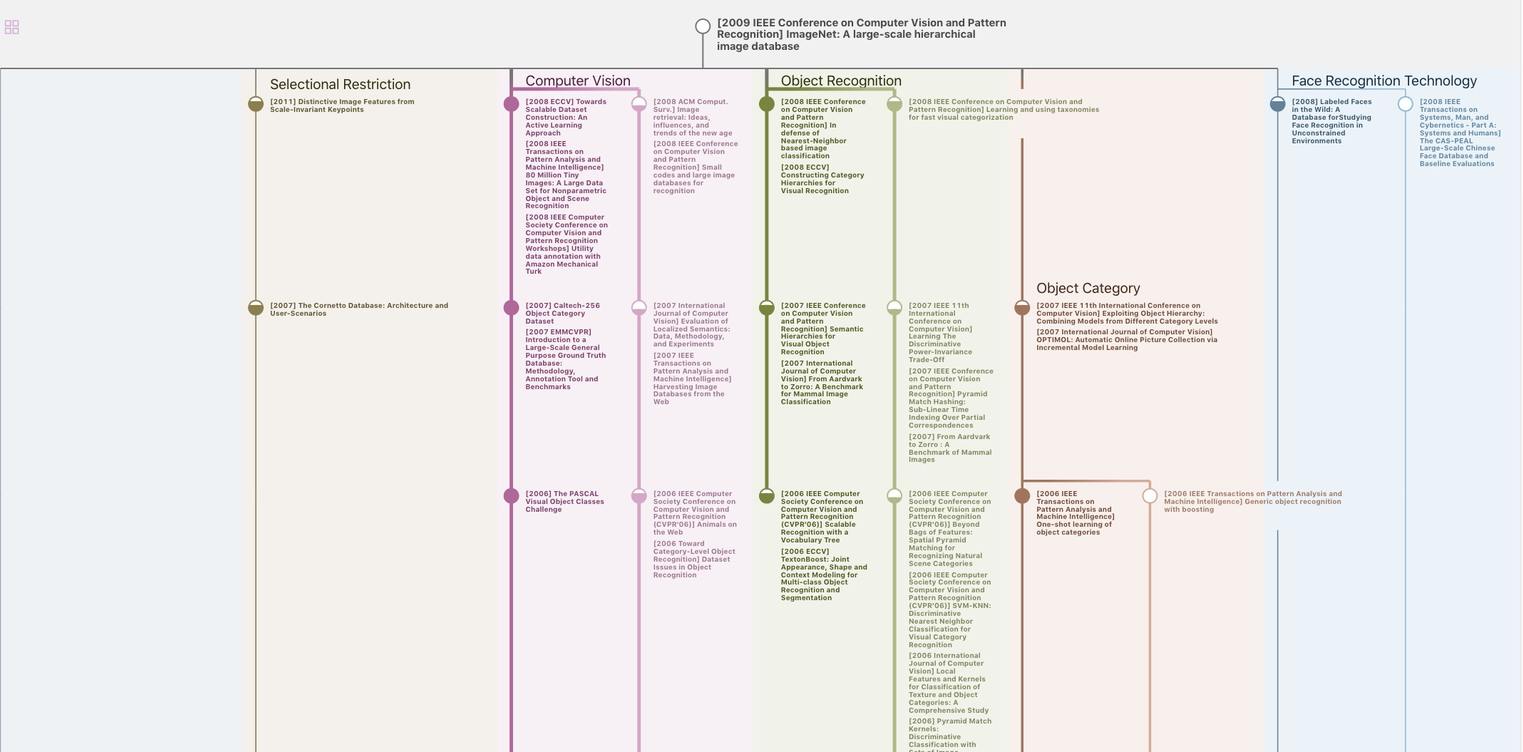
生成溯源树,研究论文发展脉络
Chat Paper
正在生成论文摘要