DeepFense: online accelerated defense against adversarial deep learning.
ICCAD-IEEE ACM International Conference on Computer-Aided Design(2018)
摘要
Recent advances in adversarial Deep Learning (DL) have opened up a largely unexplored surface for malicious attacks jeopardizing the integrity of autonomous DL systems. With the wide -spread usage of DL in critical and time -sensitive applications, including unmanned vehicles, drones, and video surveillance systems, online detection of malicious inputs is of utmost importance. We propose DeepFense, the first end-to-end automated framework that simultaneously enables efficient and safe execution of DL models. DeepFense formalizes the goal of thwarting adversarial attacks as an optimization problem that minimizes the rarely observed regions in the latent feature space spanned by a DL network. To solve the aforementioned minimization problem, a set of complementary but disjoint modular redundancies are trained to validate the legitimacy of the input samples in parallel with the victim DL model. DeepFense leverages hardware/software/algorithm co-design and customized acceleration to achieve just-in-time performance in resource -constrained settings. The proposed countermeasure is unsupervised, meaning that no adversarial sample is leveraged to train modular redundancies. We further provide an accompanying API to reduce the non-recurring engineering cost and ensure automated adaptation to various platforms. Extensive evaluations on FPGAs and GPUs demonstrate up to two orders of magnitude performance improvement while enabling online adversarial sample detection.
更多查看译文
关键词
Adversarial Attacks,Deep Learning,Model Reliability,FPGA Acceleration,Real-time Computing
AI 理解论文
溯源树
样例
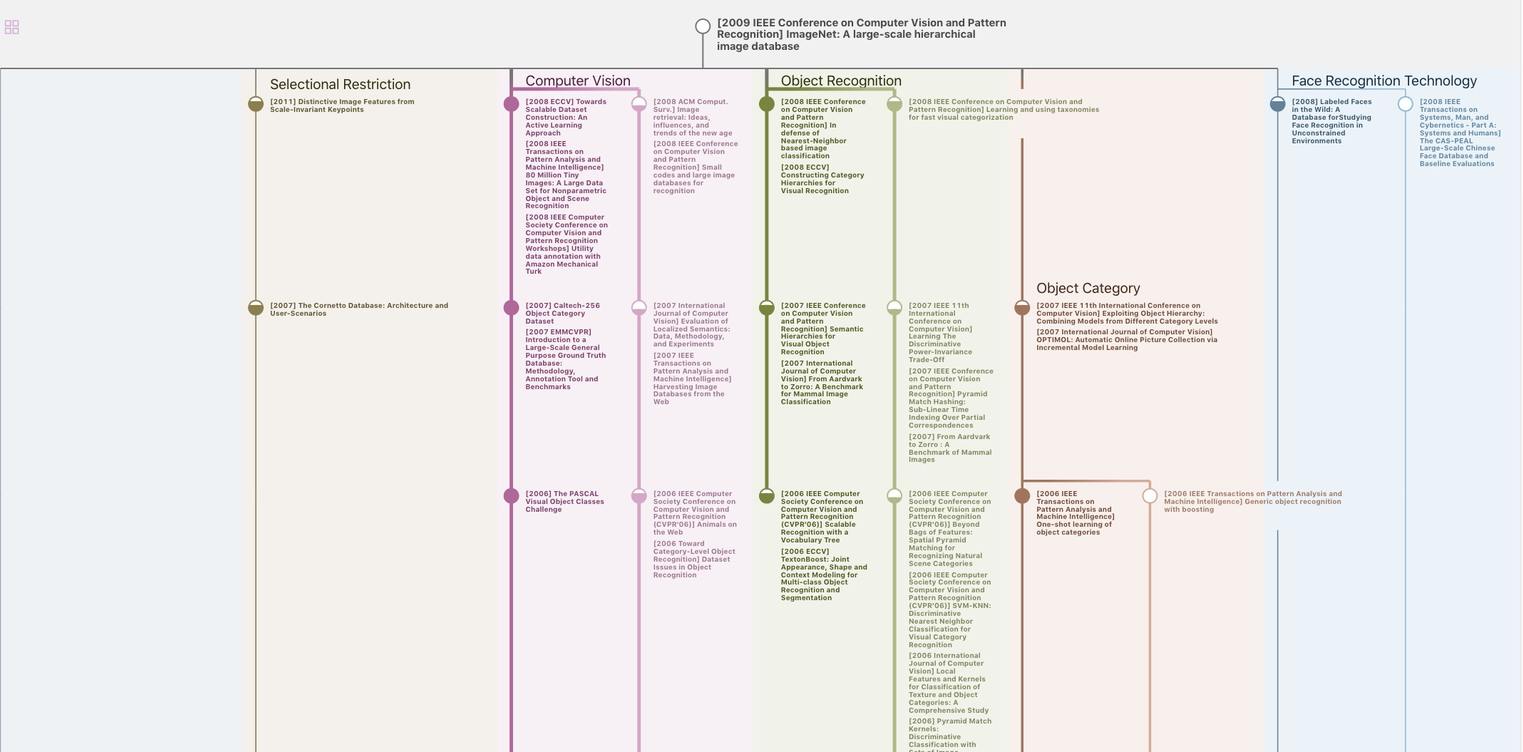
生成溯源树,研究论文发展脉络
Chat Paper
正在生成论文摘要