A Deep Automated Skeletal Bone Age Assessment Model with Heterogeneous Features Learning
J. Medical Systems(2018)
摘要
Skeletal bone age assessment is a widely used standard procedure in both disease detection and growth prediction for children in endocrinology. Conventional manual assessment methods mainly rely on personal experience in observing X-ray images of left hand and wrist to calculate bone age, which show some intrinsic limitations from low efficiency to unstable accuracy. To address these problems, some automated methods based on image processing or machine learning have been proposed, while their performances are not satisfying enough yet in assessment accuracy. Motivated by the remarkable success of deep learning (DL) techniques in the fields of image classification and speech recognition, we develop a deep automated skeletal bone age assessment model based on convolutional neural networks (CNNs) and support vector regression (SVR) using multiple kernel learning (MKL) algorithm to process heterogeneous features in this paper. This deep framework has been constructed, not only exploring the X-ray images of hand and twist but also some other heterogeneous information like race and gender. The experiment results prove its better performance with higher bone age assessment accuracy on two different data sets compared with the state of the art, indicating that the fused heterogeneous features provide a better description of the degree of bones’ maturation.
更多查看译文
关键词
Convolutional neural networks,Heterogeneous features,Skeletal bone age assessment model,Support vector regression
AI 理解论文
溯源树
样例
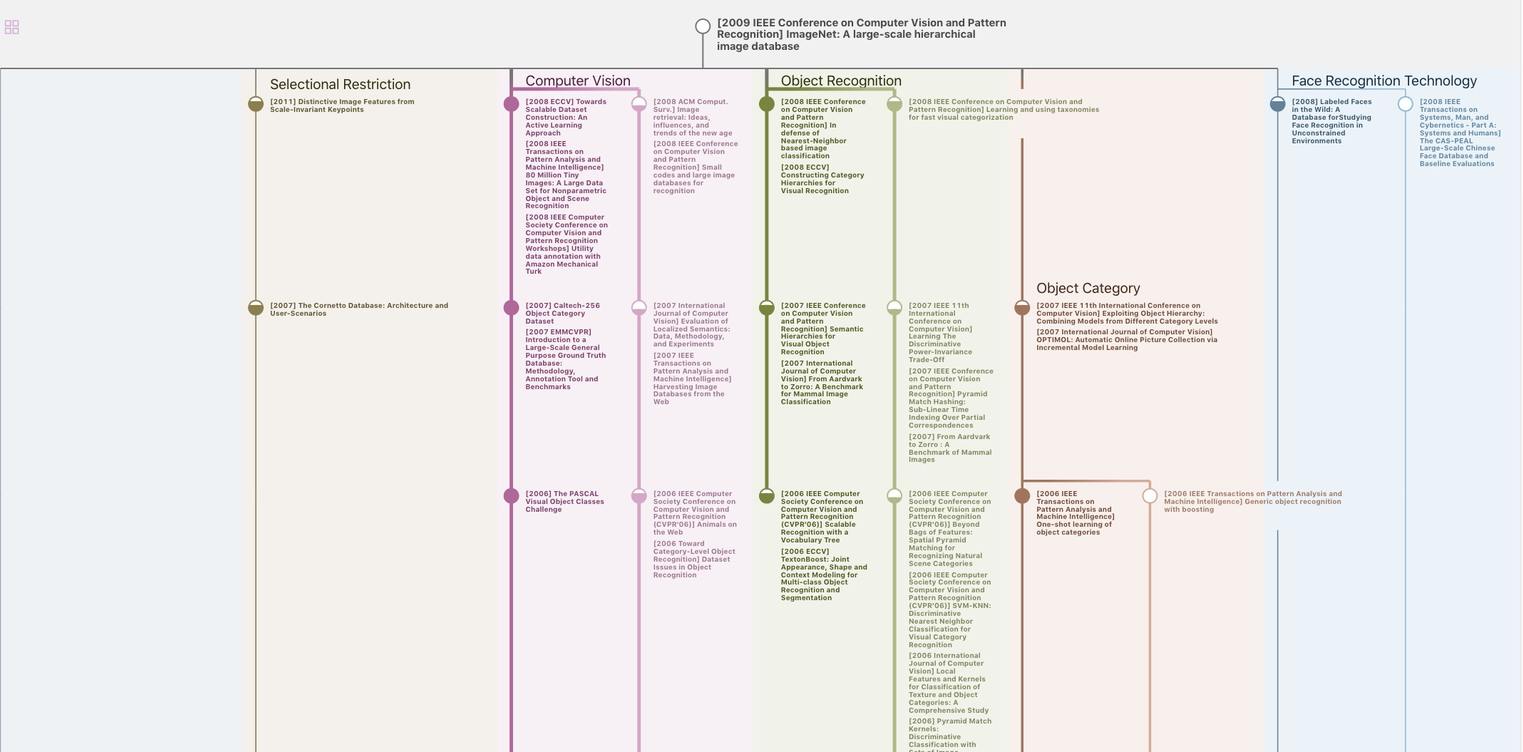
生成溯源树,研究论文发展脉络
Chat Paper
正在生成论文摘要