Countering Android Malware: A Scalable Semi-Supervised Approach for Family-Signature Generation.
IEEE ACCESS(2018)
摘要
Reducing the effort required by humans in countering malware is of utmost practical value. We describe a scalable, semi-supervised framework to dig into massive data sets of Android applications and identify new malware families. Until 2010, the industrial standard for the detection of malicious applications has been mainly based on signatures; as each tiny alteration in malware makes them ineffective, new signatures are frequently created - a task that requires a considerable amount of time and resources from skilled experts. The framework we propose is able to automatically cluster applications in families and suggest formal rules for identifying them with 100% recall and quite high precision. The families are used either to safely extend experts' knowledge on new samples or to reduce the number of applications requiring thorough analyses. We demonstrated the effectiveness and the scalability of the approach running experiments on a database of 1.5 million Android applications. In 2018, the framework has been successfully deployed on Koodous, a collaborative anti-malware platform.
更多查看译文
关键词
Semi-supervised learning,clustering,android,malware,automatic signature generation
AI 理解论文
溯源树
样例
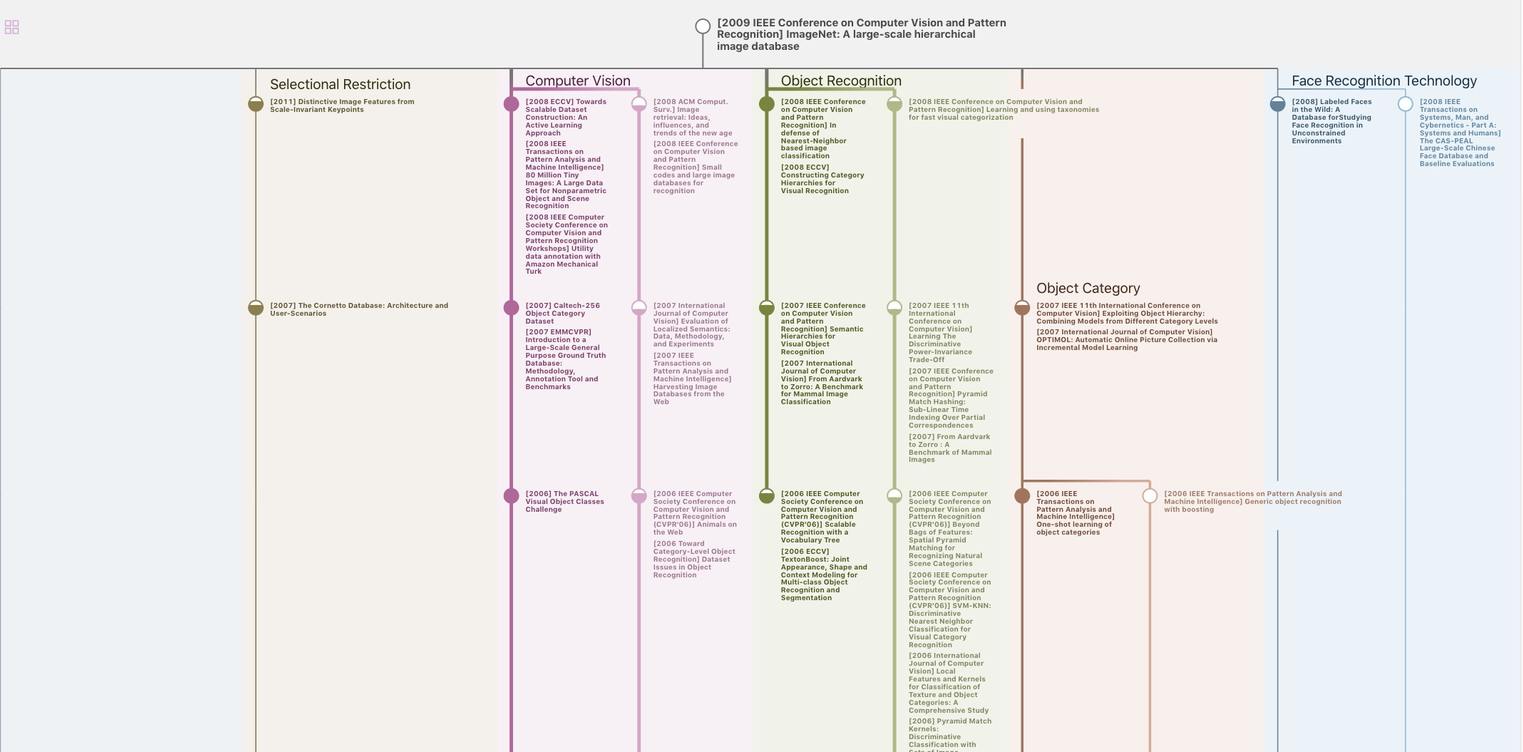
生成溯源树,研究论文发展脉络
Chat Paper
正在生成论文摘要