High-Performance Super-Resolution Via Patch-Based Deep Neural Network for Real-Time Implementation
IEICE transactions on information and systems(2018)
Abstract
Recently, Super-resolution convolutional neural network (SRCNN) is widely known as a state of the art method for achieving single-image super resolution. However, performance problems such as jaggy and ringing artifacts exist in SRCNN. Moreover, in order to realize a real-time upconverting system for high-resolution video streams such as 4K/8K 60 fps, problems such as processing delay and implementation cost remain. In the present paper, we propose high-performance super-resolution via patch-based deep neural network (SR-PDNN) rather than a convolutional neural network (CNN). Despite the very simple end-to-end learning system, the SR-PDNN achieves higher performance than the conventional CNN-based approach. In addition, this system is suitable for ultra-low-delay video processing by hardware implementation using an application-specific integrated circuit (ASIC) or a field-programmable gate array (FPGA).
MoreTranslated text
Key words
super-resolution,deep neural network,deep leaning,real-time processing
AI Read Science
Must-Reading Tree
Example
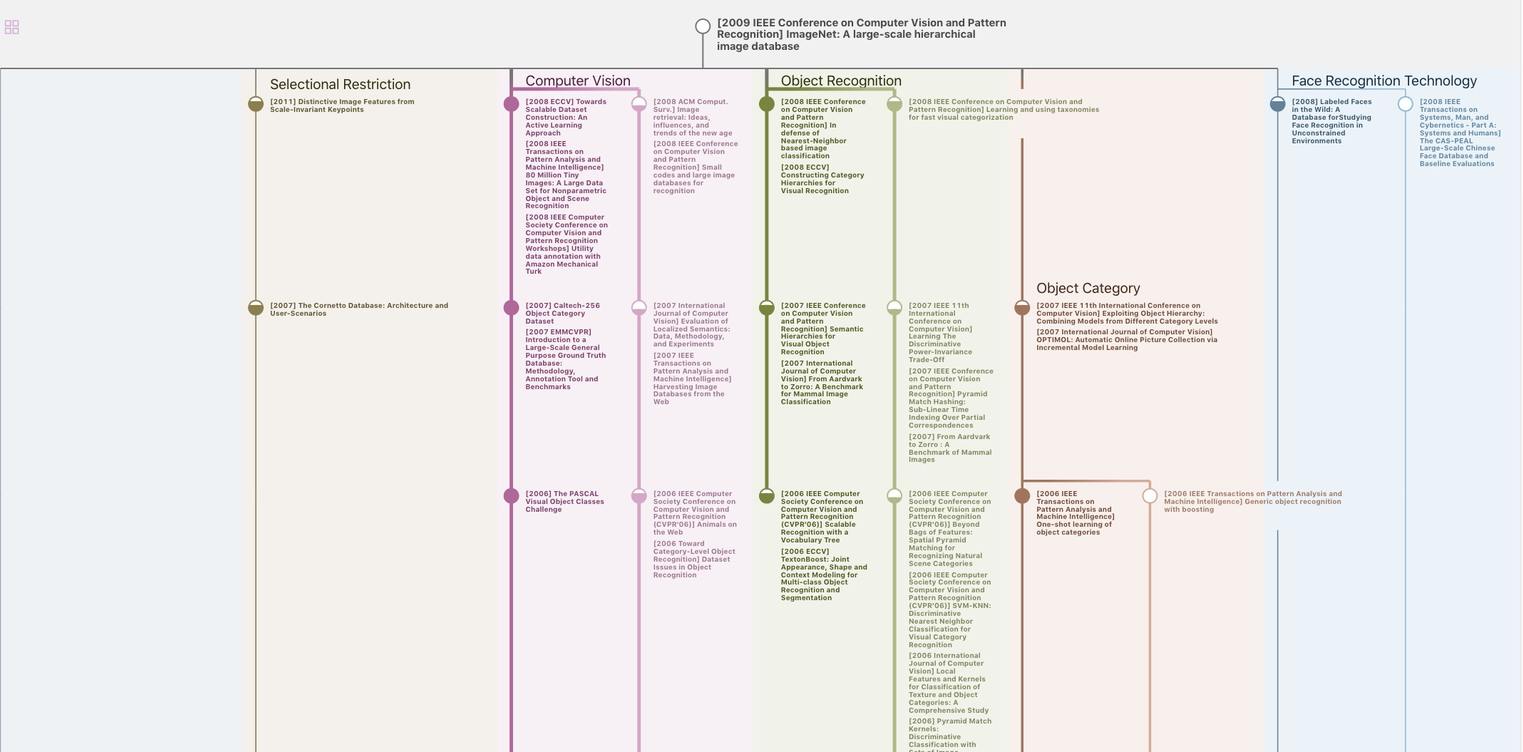
Generate MRT to find the research sequence of this paper
Chat Paper
Summary is being generated by the instructions you defined