A General Theory of Equivariant CNNs on Homogeneous Spaces
ADVANCES IN NEURAL INFORMATION PROCESSING SYSTEMS 32 (NIPS 2019)(2019)
摘要
We present a general theory of Group equivariant Convolutional Neural Networks (G-CNNs) on homogeneous spaces such as Euclidean space and the sphere. Feature maps in these networks represent fields on a homogeneous base space, and layers are equivariant maps between spaces of fields. The theory enables a systematic classification of all existing G-CNNs in terms of their symmetry group, base space, and field type. We also consider a fundamental question: what is the most general kind of equivariant linear map between feature spaces (fields) of given types? Following Mackey, we show that such maps correspond one-to-one with convolutions using equivariant kernels, and characterize the space of such kernels.
更多查看译文
关键词
general theory,homogeneous spaces,base space
AI 理解论文
溯源树
样例
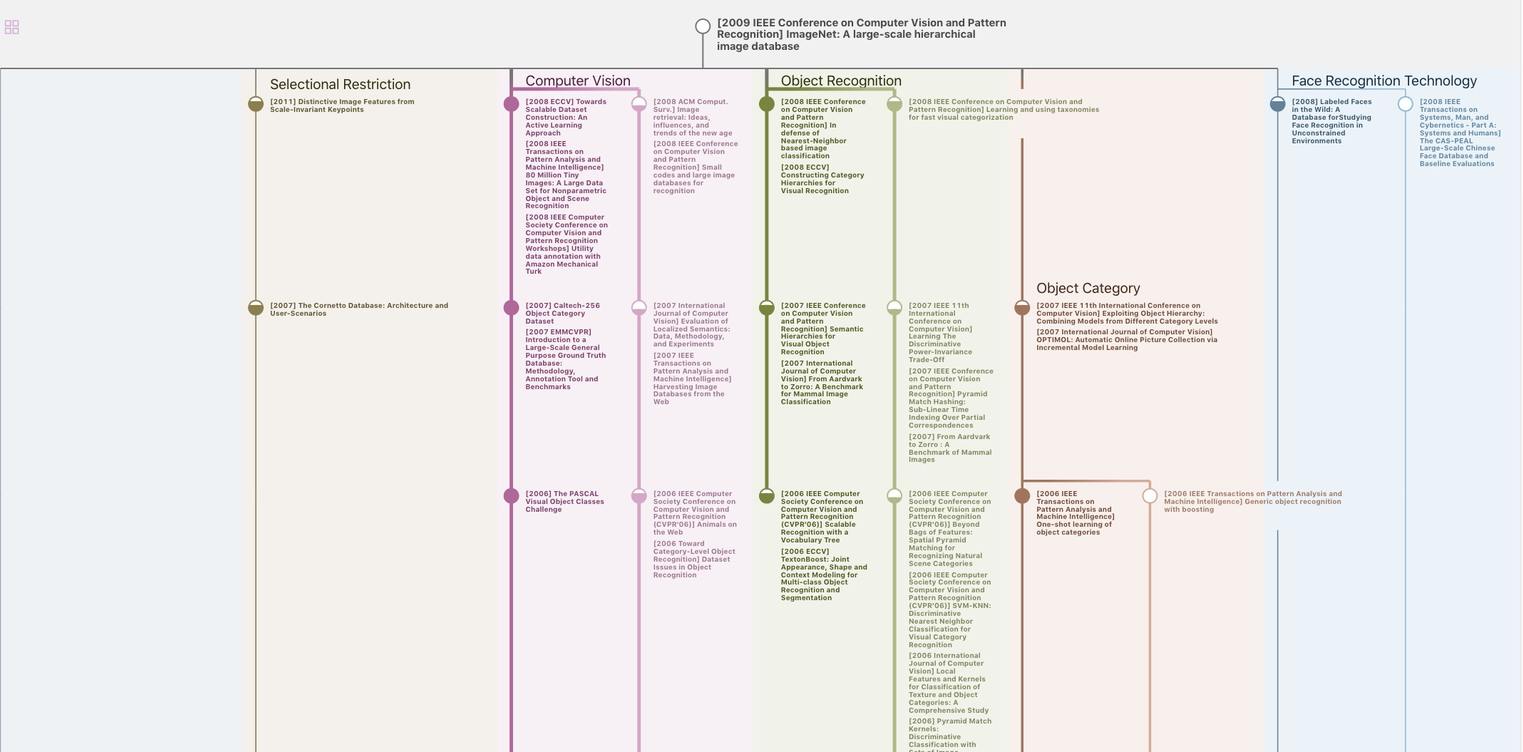
生成溯源树,研究论文发展脉络
Chat Paper
正在生成论文摘要