Understanding Deep Neural Networks through Input Uncertainties
ICASSP 2019 - 2019 IEEE International Conference on Acoustics, Speech and Signal Processing (ICASSP)(2018)
摘要
Techniques for understanding the functioning of complex machine learning models are becoming increasingly popular, not only to improve the validation process, but also to extract new insights about the data via exploratory analysis. Though a large class of such tools currently exists, most assume that predictions are point estimates and use a sensitivity analysis of these estimates to interpret the model. Using lightweight probabilistic networks we show how including prediction uncertainties in the sensitivity analysis leads to: (i) more robust and generalizable models; and (ii) a new approach for model interpretation through uncertainty decomposition. In particular, we introduce a new regularization that takes both the mean and variance of a prediction into account and demonstrate that the resulting networks provide improved generalization to unseen data. Furthermore, we propose a new technique to explain prediction uncertainties through uncertainties in the input domain, thus providing new ways to validate and interpret deep learning models.
更多查看译文
关键词
sensitivity analysis,probabilistic networks,prediction uncertainties,aleatoric uncertainties
AI 理解论文
溯源树
样例
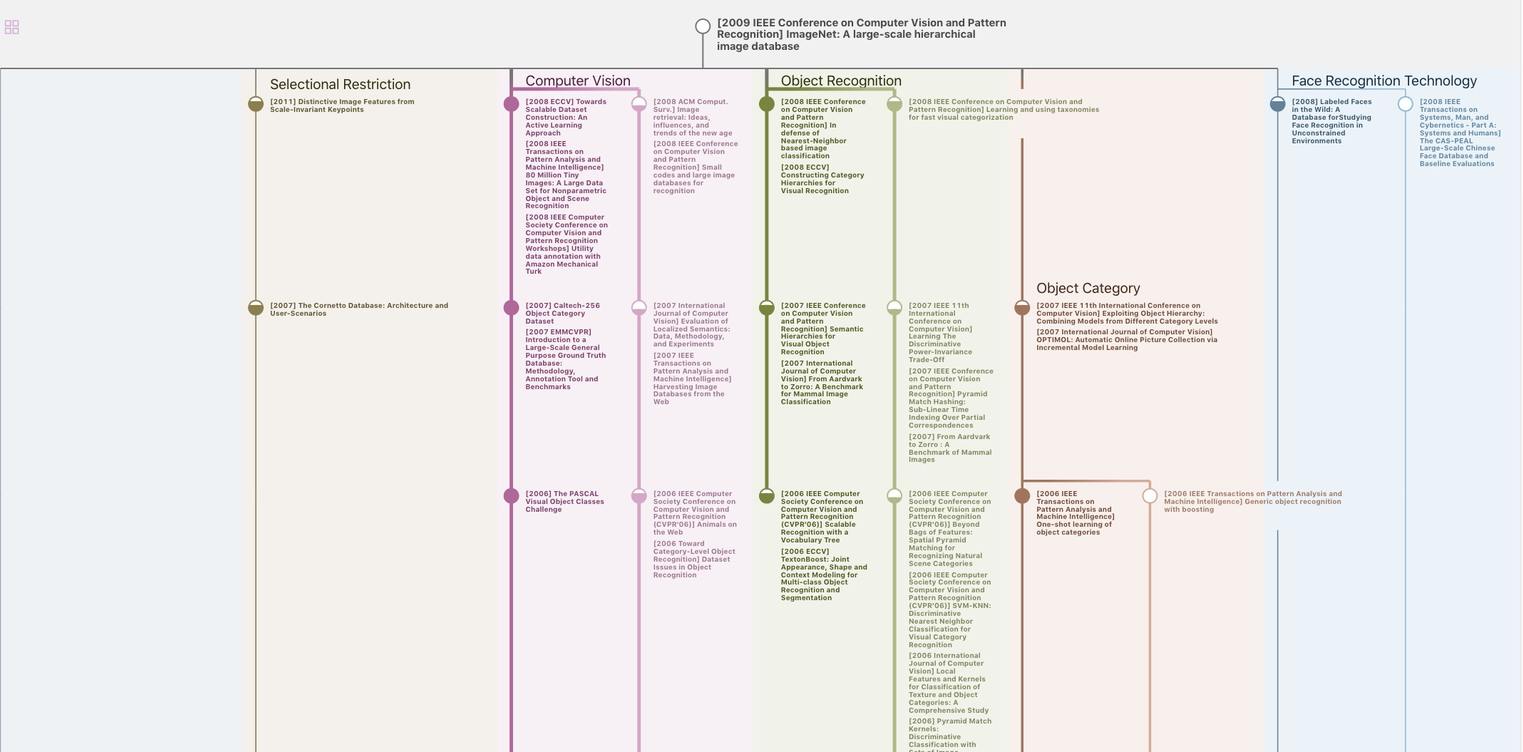
生成溯源树,研究论文发展脉络
Chat Paper
正在生成论文摘要