Deep bv: a fully automated system for brain ventricle localization and segmentation in 3d ultrasound images of embryonic mice.
2018 IEEE SIGNAL PROCESSING IN MEDICINE AND BIOLOGY SYMPOSIUM (SPMB)(2018)
摘要
Volumetric analysis of brain ventricle (BV) structure is a key tool in the study of central nervous system development in embryonic mice. High-frequency ultrasound (HFU) is the only non-invasive, real-time modality available for rapid volumetric imaging of embryos in utero. However, manual segmentation of the BV from HFU volumes is tedious, time-consuming, and requires specialized expertise. In this paper, we propose a novel deep learning based BV segmentation system for whole-body HFU images of mouse embryos. Our fully automated system consists of two modules: localization and segmentation. It first applies a volumetric convolutional neural network on a 3D sliding window over the entire volume to identify a 3D bounding box containing the entire BV. It then employs a fully convolutional network to segment the detected bounding box into BV and background. The system achieves a Dice Similarity Coefficient (DSC) of 0.8956 for BV segmentation on an unseen 111 HFU volume test set surpassing the previous state-of-the-art method (DSC of 0.7119) by a margin of 25%.
更多查看译文
关键词
whole-body HFU images,mouse embryos,fully automated system,volumetric convolutional neural network,3D sliding window,3D bounding box,fully convolutional network,brain ventricle localization,3D ultrasound images,embryonic mice,volumetric analysis,brain ventricle structure,central nervous system development,high-frequency ultrasound,real-time modality,manual segmentation,deep BV,HFU volume test,apid volumetric imaging,utero,Dice similarity coefficient,deep learning based BV segmentation system
AI 理解论文
溯源树
样例
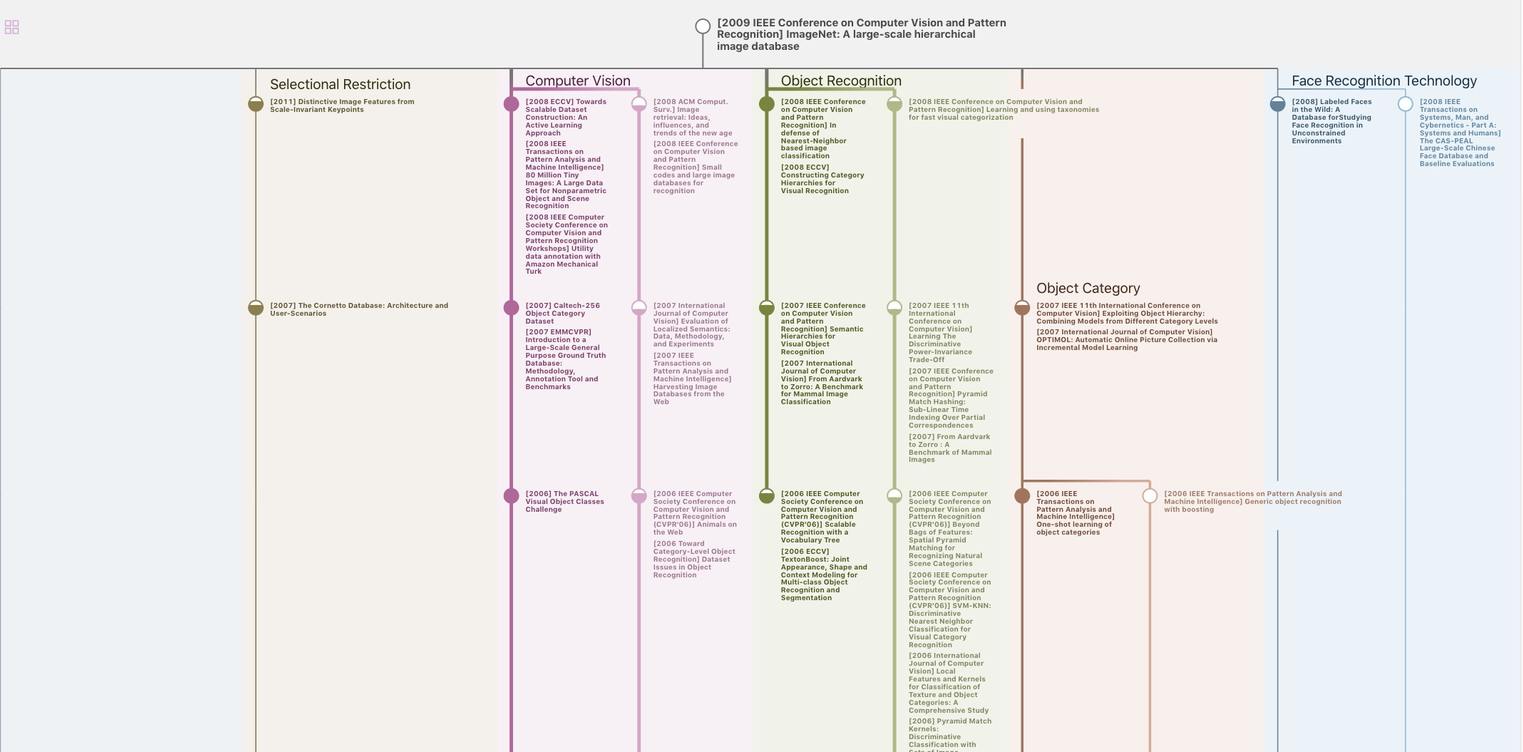
生成溯源树,研究论文发展脉络
Chat Paper
正在生成论文摘要