Understanding the impact of entropy on policy optimization
INTERNATIONAL CONFERENCE ON MACHINE LEARNING, VOL 97(2019)
摘要
Entropy regularization is commonly used to improve policy optimization in reinforcement learning. It is believed to help with \emph{exploration} by encouraging the selection of more stochastic policies. In this work, we analyze this claim using new visualizations of the optimization landscape based on randomly perturbing the loss function. We first show that even with access to the exact gradient, policy optimization is difficult due to the geometry of the objective function. Then, we qualitatively show that in some environments, a policy with higher entropy can make the optimization landscape smoother, thereby connecting local optima and enabling the use of larger learning rates. This manuscript presents new tools for understanding the optimization landscape, shows that policy entropy serves as a regularizer, and highlights the challenge of designing general-purpose policy optimization algorithms.
更多查看译文
AI 理解论文
溯源树
样例
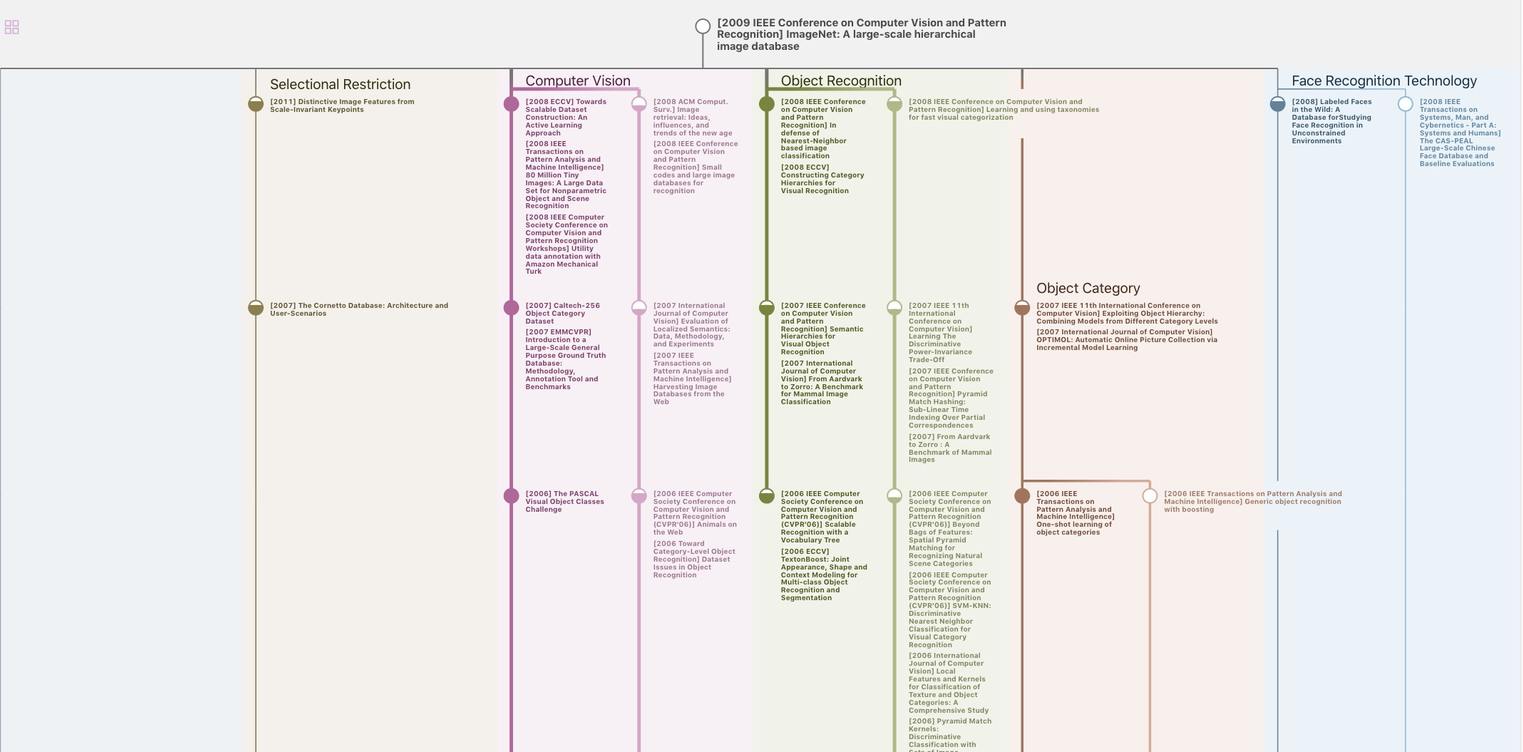
生成溯源树,研究论文发展脉络
Chat Paper
正在生成论文摘要