Demonstrating Advantages of Neuromorphic Computation: A Pilot Study.
FRONTIERS IN NEUROSCIENCE(2019)
摘要
Neuromorphic devices represent an attempt to mimic aspects of the brain's architecture and dynamics with the aim of replicating its hallmark functional capabilities in terms of computational power, robust learning and energy efficiency. We employ a single-chip prototype of the BrainScaleS 2 neuromorphic system to implement a proof-of-concept demonstration of reward-modulated spike-timing-dependent plasticity in a spiking network that learns to play a simplified version of the Pong video game by smooth pursuit. This system combines an electronic mixed-signal substrate for emulating neuron and synapse dynamics with an embedded digital processor for on-chip learning, which in this work also serves to simulate the virtual environment and learning agent. The analog emulation of neuronal membrane dynamics enables a 1000-fold acceleration with respect to biological real-time, with the entire chip operating on a power budget of 57 mW. Compared to an equivalent simulation using state-of-the-art software, the on-chip emulation is at least one order of magnitude faster and three orders of magnitude more energy-efficient. We demonstrate how on-chip learning can mitigate the effects of fi xed-pattern noise, which is unavoidable in analog substrates, while making use of temporal variability for action exploration. Learning compensates imperfections of the physical substrate, as manifested in neuronal parameter variability, by adapting synaptic weights to match respective excitability of individual neurons.
更多查看译文
关键词
BrainScaleS,mixed-signal,neuromorphic computing,spiking neural networks,reinforcement learning,STDP,plasticity
AI 理解论文
溯源树
样例
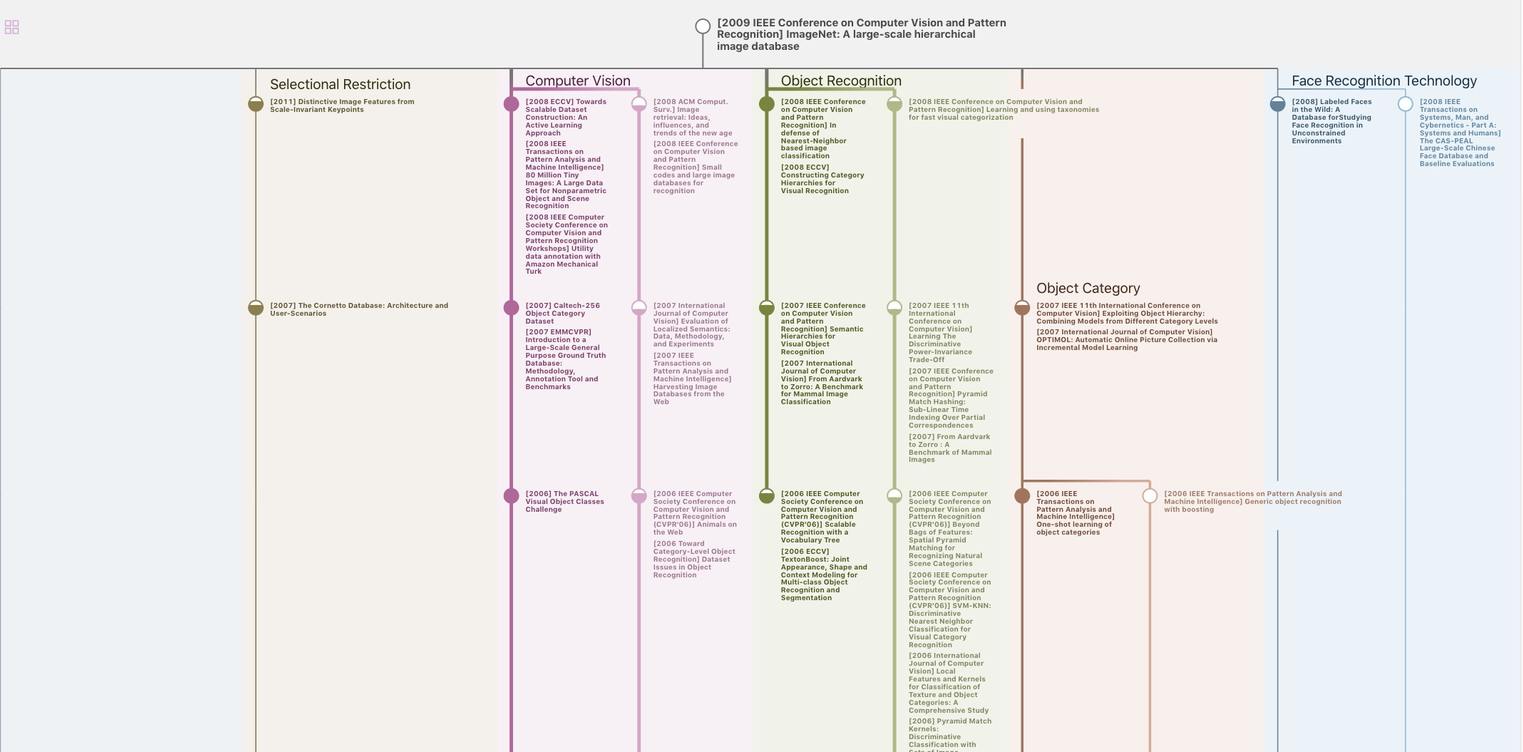
生成溯源树,研究论文发展脉络
Chat Paper
正在生成论文摘要