Locally Private Gaussian Estimation
ADVANCES IN NEURAL INFORMATION PROCESSING SYSTEMS 32 (NIPS 2019)(2019)
摘要
We study a basic private estimation problem: each of n users draws a single i.i.d. sample from an unknown Gaussian distribution N(mu, sigma(2)), and the goal is to estimate mu while guaranteeing local differential privacy for each user. As minimizing the number of rounds of interaction is important in the local setting, we provide adaptive two-round solutions and nonadaptive one-round solutions to this problem. We match these upper bounds with an information-theoretic lower bound showing that our accuracy guarantees are tight up to logarithmic factors for all sequentially interactive locally private protocols.
更多查看译文
关键词
local differential privacy
AI 理解论文
溯源树
样例
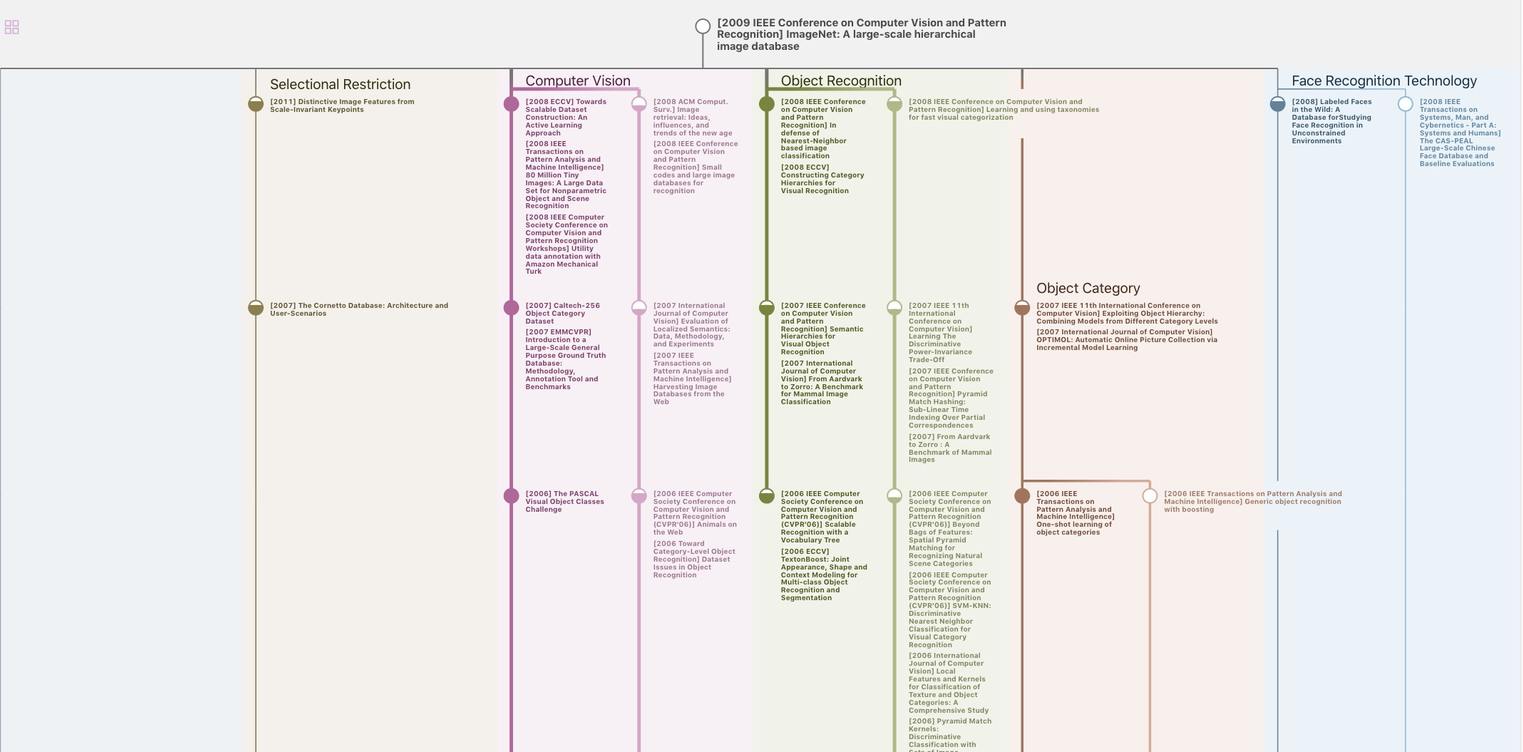
生成溯源树,研究论文发展脉络
Chat Paper
正在生成论文摘要