Differentiable MPC for End-to-end Planning and Control.
ADVANCES IN NEURAL INFORMATION PROCESSING SYSTEMS 31 (NIPS 2018)(2018)
摘要
We present foundations for using Model Predictive Control (MPC) as a differentiable policy class for reinforcement learning in continuous state and action spaces. This provides one way of leveraging and combining the advantages of model-free and model-based approaches. Specifically, we differentiate through MPC by using the KKT conditions of the convex approximation at a fixed point of the controller. Using this strategy, we are able to learn the cost and dynamics of a controller via end-to-end learning. Our experiments focus on imitation learning in the pendulum and cartpole domains, where we learn the cost and dynamics terms of an MPC policy class. We show that our MPC policies are significantly more data-efficient than a generic neural network and that our method is superior to traditional system identification in a setting where the expert is unrealizable.
更多查看译文
关键词
reinforcement learning,model predictive control,fixed point,kkt conditions,end-to-end learning
AI 理解论文
溯源树
样例
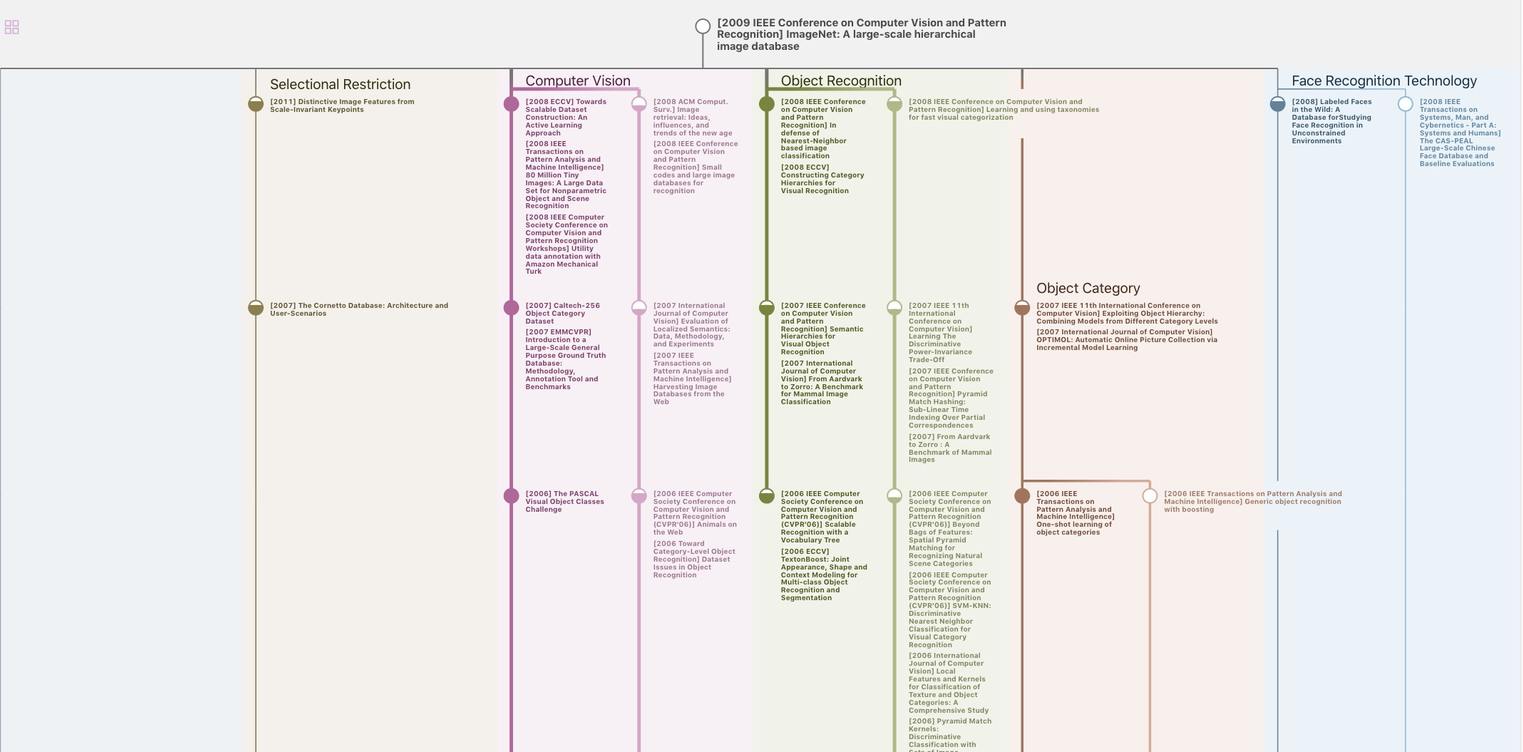
生成溯源树,研究论文发展脉络
Chat Paper
正在生成论文摘要