Self-Paced Adversarial Training for Multimodal Few-Shot Learning
CoRR(2019)
摘要
State-of-the-art deep learning algorithms yield remark-able results in many visual recognition tasks. However, they still fail to provide satisfactory results in scarce data regimes. To a certain extent this lack of data can be compensated by multimodal information. Missing information in one modality of a single data point (e.g. an image) can be made up for in another modality (e.g. a textual description). Therefore, we design a few-shot learning task that is multimodal during training (i.e. image and text) and single-modal during test time (i.e. image). In this regard, we pro-pose a self-paced class-discriminative generative adversarial network incorporating multimodality in the context off ew-shot learning. The proposed approach builds upon the idea of cross-modal data generation in order to alleviate the data sparsity problem. We improve few-shot learning accuracies on the fine grained CUB and Oxford-102 datasets.
更多查看译文
关键词
Training,Gallium nitride,Generators,Visualization,Task analysis,Generative adversarial networks,Training data
AI 理解论文
溯源树
样例
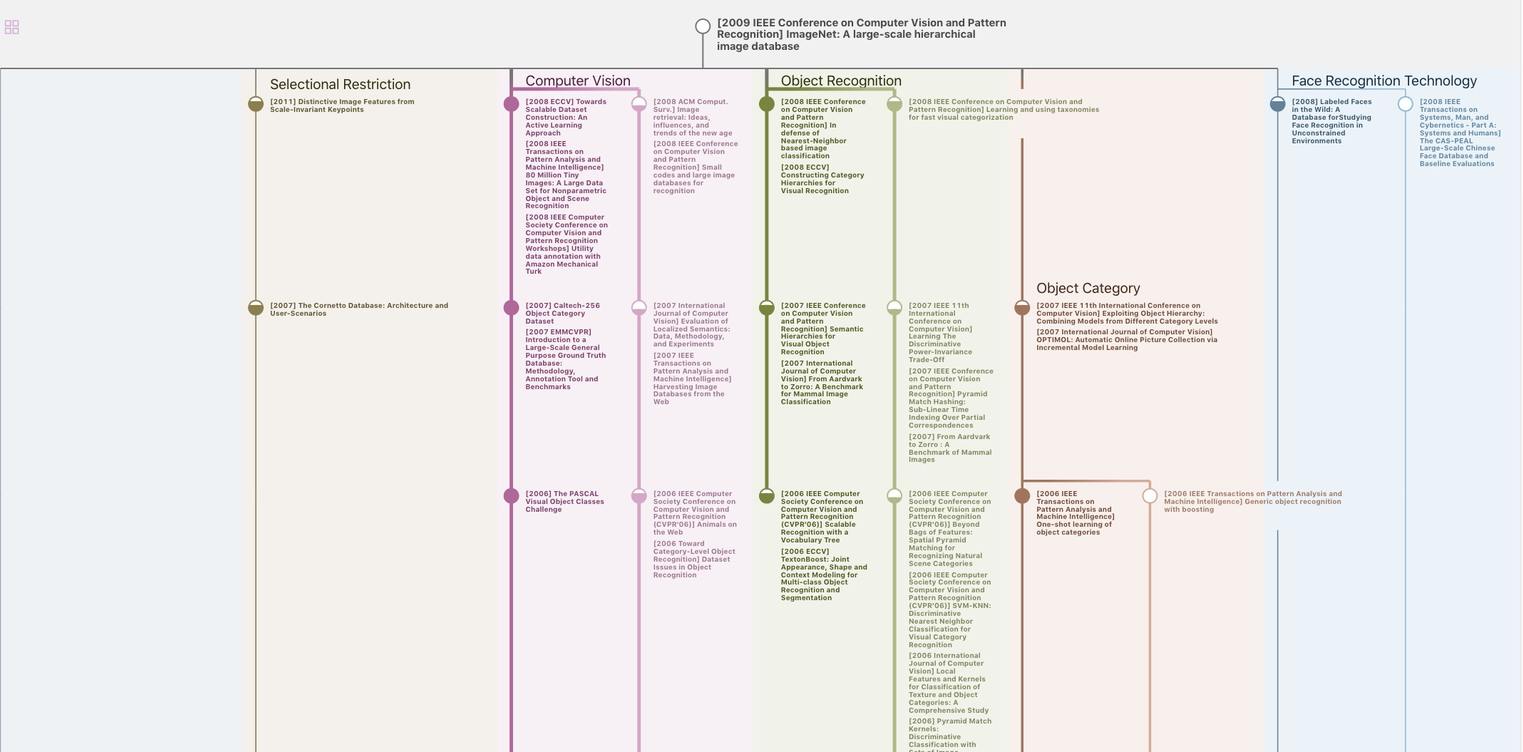
生成溯源树,研究论文发展脉络
Chat Paper
正在生成论文摘要