Temporal Recurrent Networks for Online Action Detection
2019 IEEE/CVF International Conference on Computer Vision (ICCV)(2019)
摘要
Most work on temporal action detection is formulated as an offline problem, in which the start and end times of actions are determined after the entire video is fully observed. However, important real-time applications including surveillance and driver assistance systems require identifying actions as soon as each video frame arrives, based only on current and historical observations. In this paper, we propose a novel framework, Temporal Recurrent Network (TRN), to model greater temporal context of a video frame by simultaneously performing online action detection and anticipation of the immediate future. At each moment in time, our approach makes use of both accumulated historical evidence and predicted future information to better recognize the action that is currently occurring, and integrates both of these into a unified end-to-end architecture. We evaluate our approach on two popular online action detection datasets, HDD and TVSeries, as well as another widely used dataset, THUMOS'14. The results show that TRN significantly outperforms the state-of-the-art.
更多查看译文
关键词
Temporal Recurrent Networks,temporal action detection,offline problem,real-time applications,driver assistance systems,video frame,historical observations,temporal context,accumulated historical evidence,unified end-to-end architecture,online action detection datasets,surveillance,HDD,TVSeries
AI 理解论文
溯源树
样例
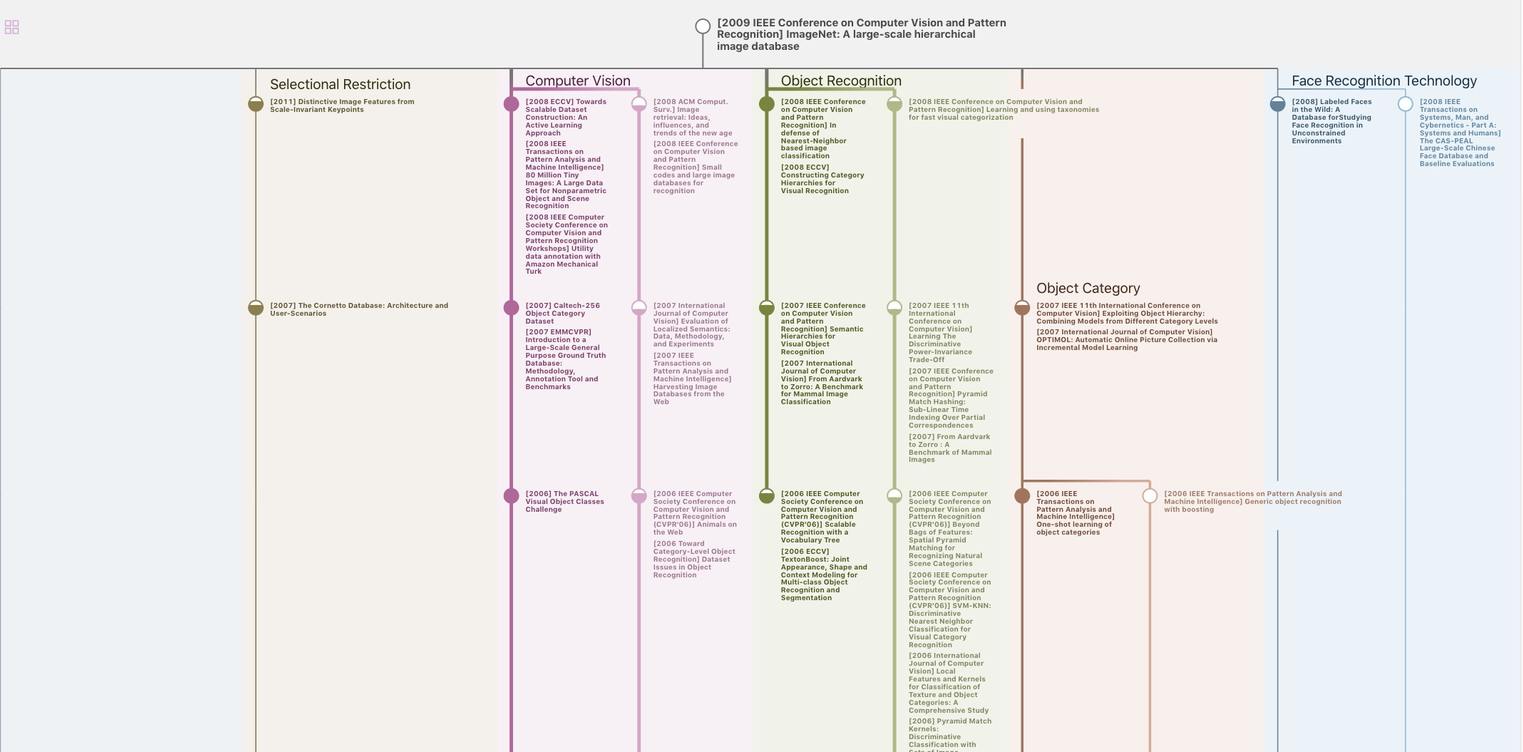
生成溯源树,研究论文发展脉络
Chat Paper
正在生成论文摘要