An Online-Learning Approach to Inverse Optimization
arXiv: Optimization and Control(2018)
摘要
In this paper, we demonstrate how to learn the objective function of a decision-maker while only observing the problem input data and the decision-maker's corresponding decisions over multiple rounds. We present exact algorithms for this online version of inverse optimization which converge at a rate of 𝒪(1/√(T)) in the number of observations T and compare their further properties. Especially, they all allow taking decisions which are essentially as good as those of the observed decision-maker already after relatively few iterations, but are suited best for different settings each. Our approach is based on online learning and works for linear objectives over arbitrary feasible sets for which we have a linear optimization oracle. As such, it generalizes previous approaches based on KKT-system decomposition and dualization. We also introduce several generalizations, such as the approximate learning of non-linear objective functions, dynamically changing as well as parameterized objectives and the case of suboptimal observed decisions. When applied to the stochastic offline case, our algorithms are able to give guarantees on the quality of the learned objectives in expectation. Finally, we show the effectiveness and possible applications of our methods in indicative computational experiments.
更多查看译文
关键词
optimization,online-learning online-learning
AI 理解论文
溯源树
样例
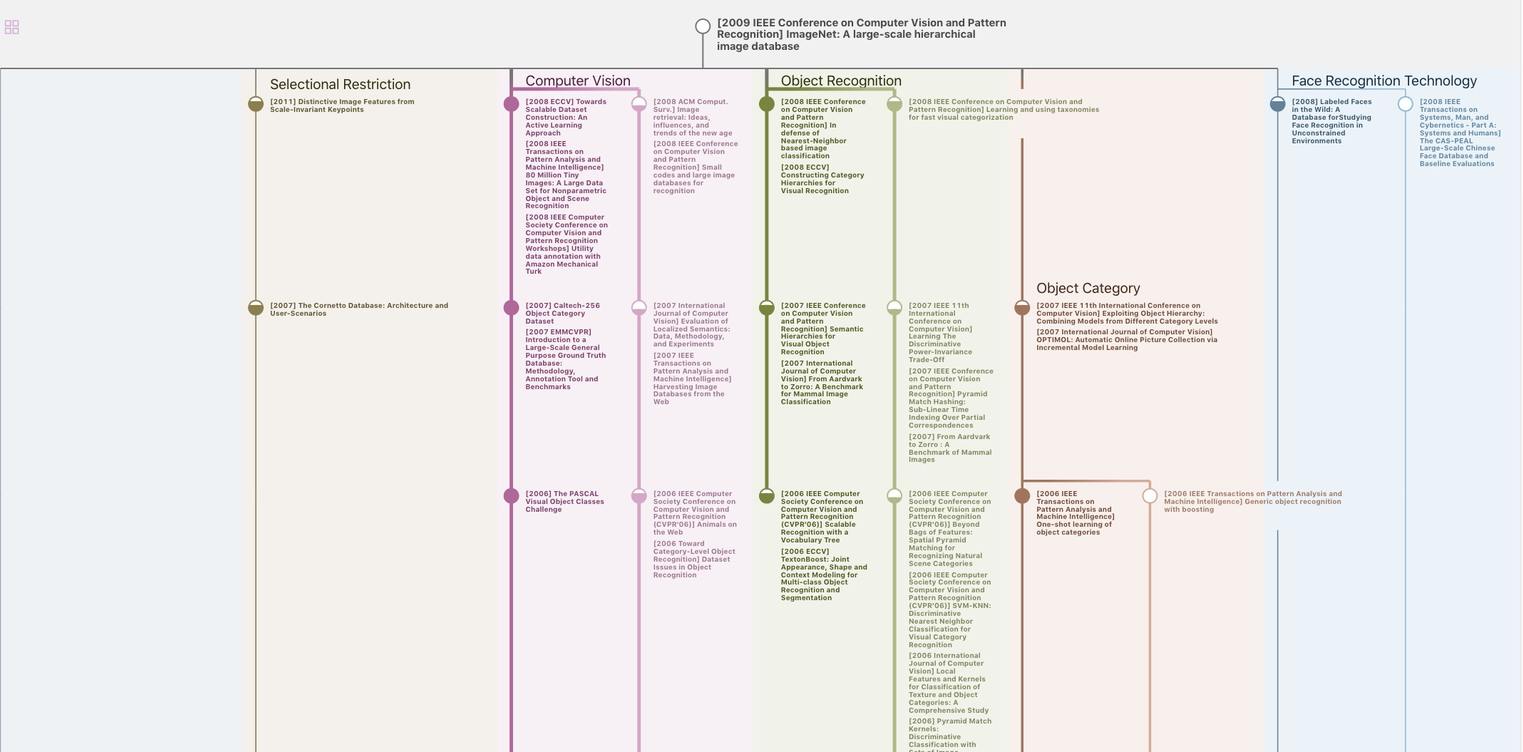
生成溯源树,研究论文发展脉络
Chat Paper
正在生成论文摘要