On The Inductive Bias of Words in Acoustics-to-Word Models.
arXiv: Audio and Speech Processing(2018)
摘要
Acoustics-to-word models are end-to-end speech recognizers that use words as targets without relying on pronunciation dictionaries or graphemes. These models are notoriously difficult to train due to the lack of linguistic knowledge. It is also unclear how the amount of training data impacts the optimization and generalization of such models. In this work, we study the optimization and generalization of acoustics-to-word models under different amounts of training data. In addition, we study three types of inductive bias, leveraging a pronunciation dictionary, word boundary annotations, and constraints on word durations. We find that constraining word durations leads to the most improvement. Finally, we analyze the word embedding space learned by the model, and find that the space has a structure dominated by the pronunciation of words. This suggests that the contexts of words, instead of their phonetic structure, should be the future focus of inductive bias in acoustics-to-word models.
更多查看译文
AI 理解论文
溯源树
样例
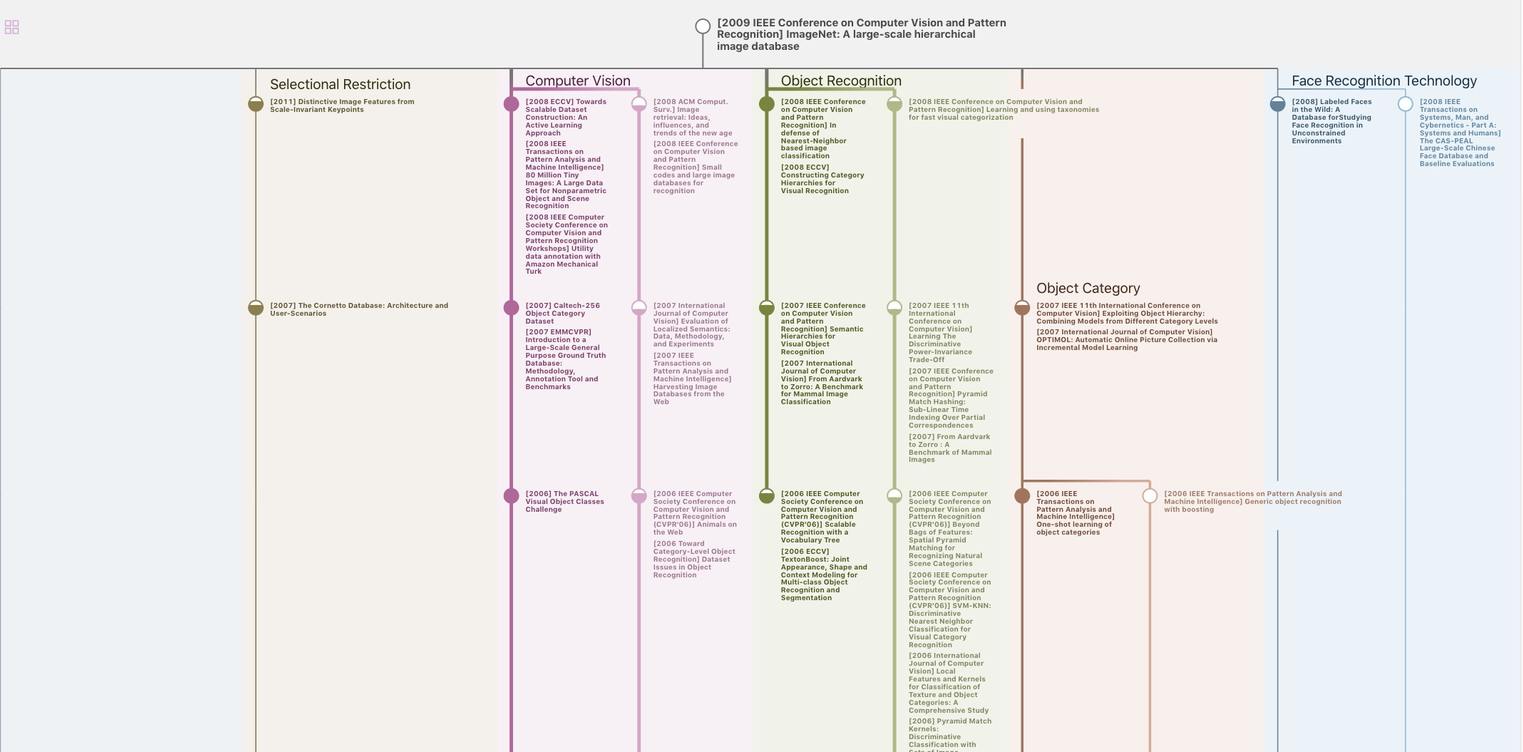
生成溯源树,研究论文发展脉络
Chat Paper
正在生成论文摘要