Affinity propagation clustering-aided two-label hierarchical extreme learning machine for Wi-Fi fingerprinting-based indoor positioning
Journal of Ambient Intelligence and Humanized Computing(2022)
摘要
With the rapid growth of indoor location-based services (LBS) along with the internet of things (IoT) applications in daily life, the development of indoor positioning systems has attracted significant attention. Since the global positioning system (GPS) struggles to perform indoors, cost-effective Wi-Fi fingerprinting has become a popular alternative for indoor positioning over the past decade. Accurate identification of the floor level in complex multi-floor buildings is a prerequisite for the success of the positioning systems. Nevertheless, Wi-Fi positioning accuracy is not satisfactory, mainly due to the signal overlap in the adjacent floors with hollow areas, device heterogeneity, and signal attenuation. In this paper, to overcome these issues, we propose a two-label hierarchical extreme learning machine. The affinity propagation clustering is applied to place overlapping signal points in the same cluster and improve deep learning-based ELM performance reducing the learning parameters of the classification layer. Then, two clusters and a floor label are assigned to each sample. In the training phase, the proposed model is trained based on the new labeled data. The final location is calculated based on the determined cluster and floor, in the positioning phase. The experimental result shows that the proposed method increases the average positioning accuracy by up to 40% compared to state-of-the-art methods. Also, in terms of floor identification, it outperforms compared methods.
更多查看译文
关键词
Affinity propagation clustering, Indoor positioning, Multi-floor building, Two-label hierarchical extreme learning machine, Wi-Fi fingerprinting
AI 理解论文
溯源树
样例
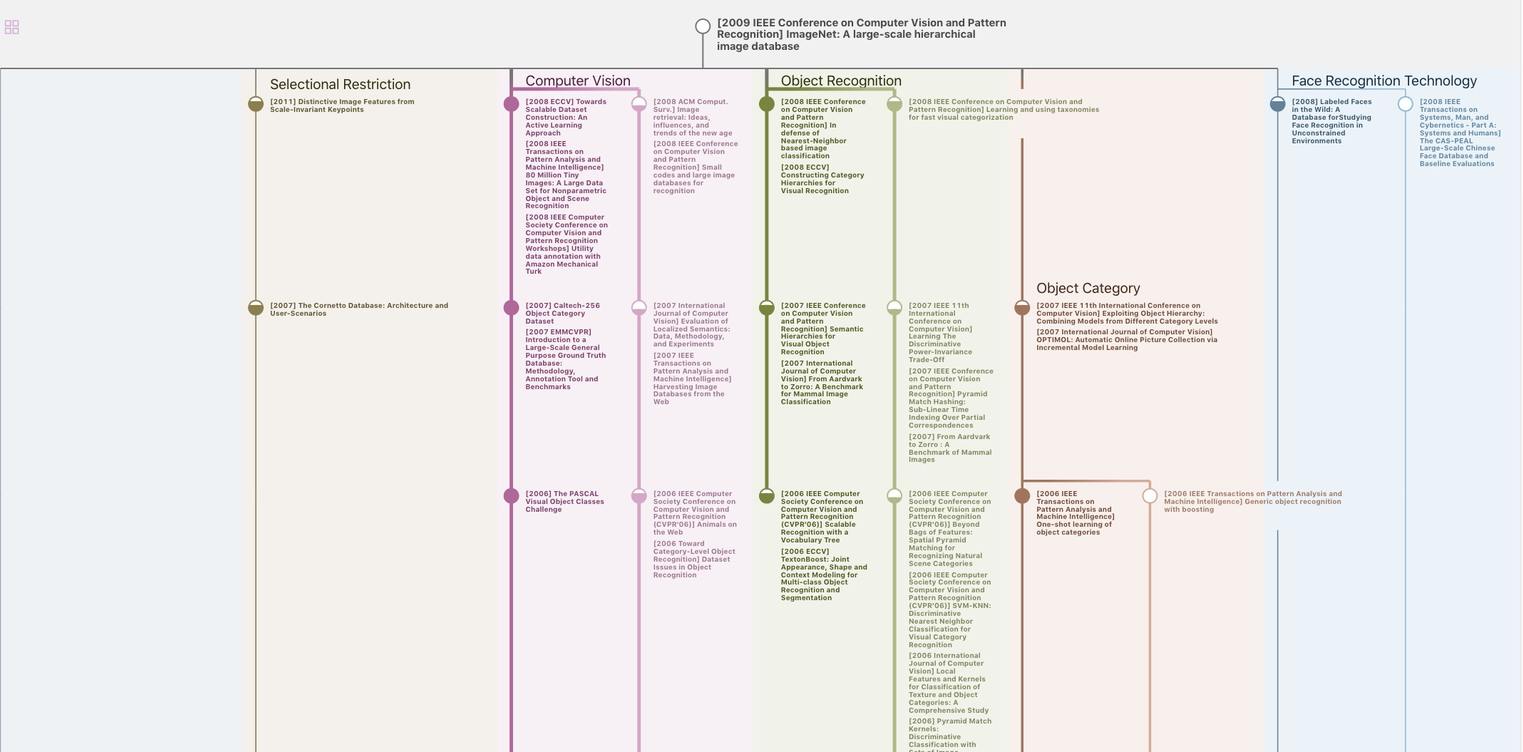
生成溯源树,研究论文发展脉络
Chat Paper
正在生成论文摘要