Distribution Discrepancy Maximization for Image Privacy Preserving
2019 IEEE INTERNATIONAL CONFERENCE ON MULTIMEDIA & EXPO WORKSHOPS (ICMEW)(2019)
摘要
With the rapid increase in online photo sharing activities, image obfuscation algorithms become particularly important for protecting the sensitive information in the shared photos. However, existing image obfuscation methods based on hand-crafted principles are challenged by the dramatic development of deep learning techniques. To address this problem, we propose to maximize the distribution discrepancy between the original image domain and the encrypted image domain. Accordingly, we introduce a collaborative training scheme: a discriminator D is trained to discriminate the reconstructed image from the encrypted image, and an encryption model G_e is required to generate these two kinds of images to maximize the recognition rate of D, leading to the same training objective for both D and G_e. We theoretically prove that such a training scheme maximizes two distributions' discrepancy. Compared with commonly-used image obfuscation methods, our model can produce satisfactory defense against the attack of deep recognition models indicated by significant accuracy decreases on Casia-WebFace and LFW datasets.
更多查看译文
关键词
Privacy Preserving, Collaborative Training, Distribution Discrepancy Maximization
AI 理解论文
溯源树
样例
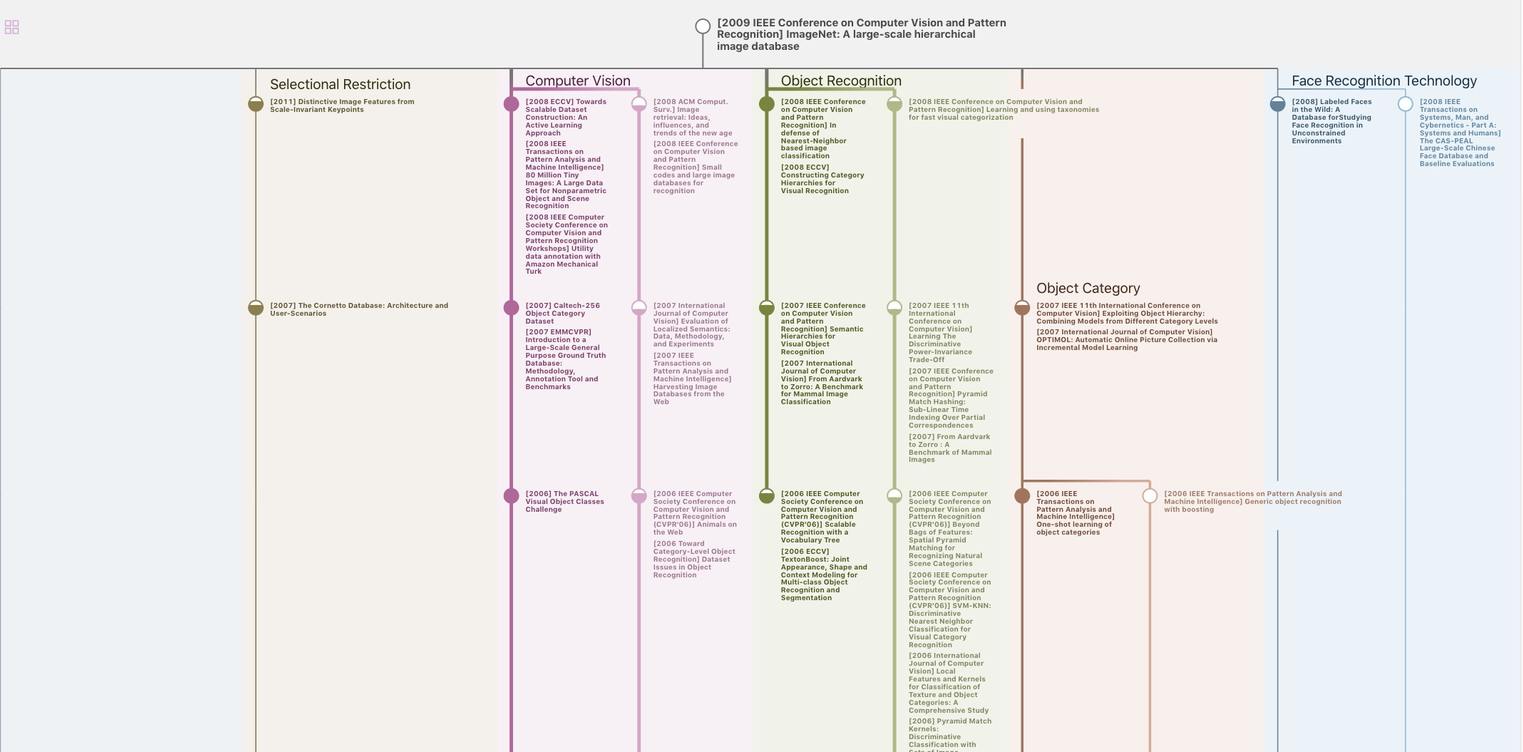
生成溯源树,研究论文发展脉络
Chat Paper
正在生成论文摘要