Ensemble Machine Learning And Forecasting Can Achieve 99% Uptime For Rural Handpumps
PLOS ONE(2017)
摘要
Broken water pumps continue to impede efforts to deliver clean and economically-viable water to the global poor. The literature has demonstrated that customers' health benefits and willingness to pay for clean water are best realized when clean water infrastructure performs extremely well (> 99% uptime). In this paper, we used sensor data from 42 Afridevbrand handpumps observed for 14 months in western Kenya to demonstrate how sensors and supervised ensemble machine learning could be used to increase total fleet uptime from a best-practices baseline of about 70% to > 99%. We accomplish this increase in uptime by forecasting pump failures and identifying existing failures very quickly. Comparing the costs of operating the pump per functional year over a lifetime of 10 years, we estimate that implementing this algorithm would save 7% on the levelized cost of water relative to a sensor-less scheduled maintenance program. Combined with a rigorous system for dispatching maintenance personnel, implementing this algorithm in a real-world program could significantly improve health outcomes and customers' willingness to pay for water services.
更多查看译文
关键词
ensemble machine learning,forecasting,machine learning
AI 理解论文
溯源树
样例
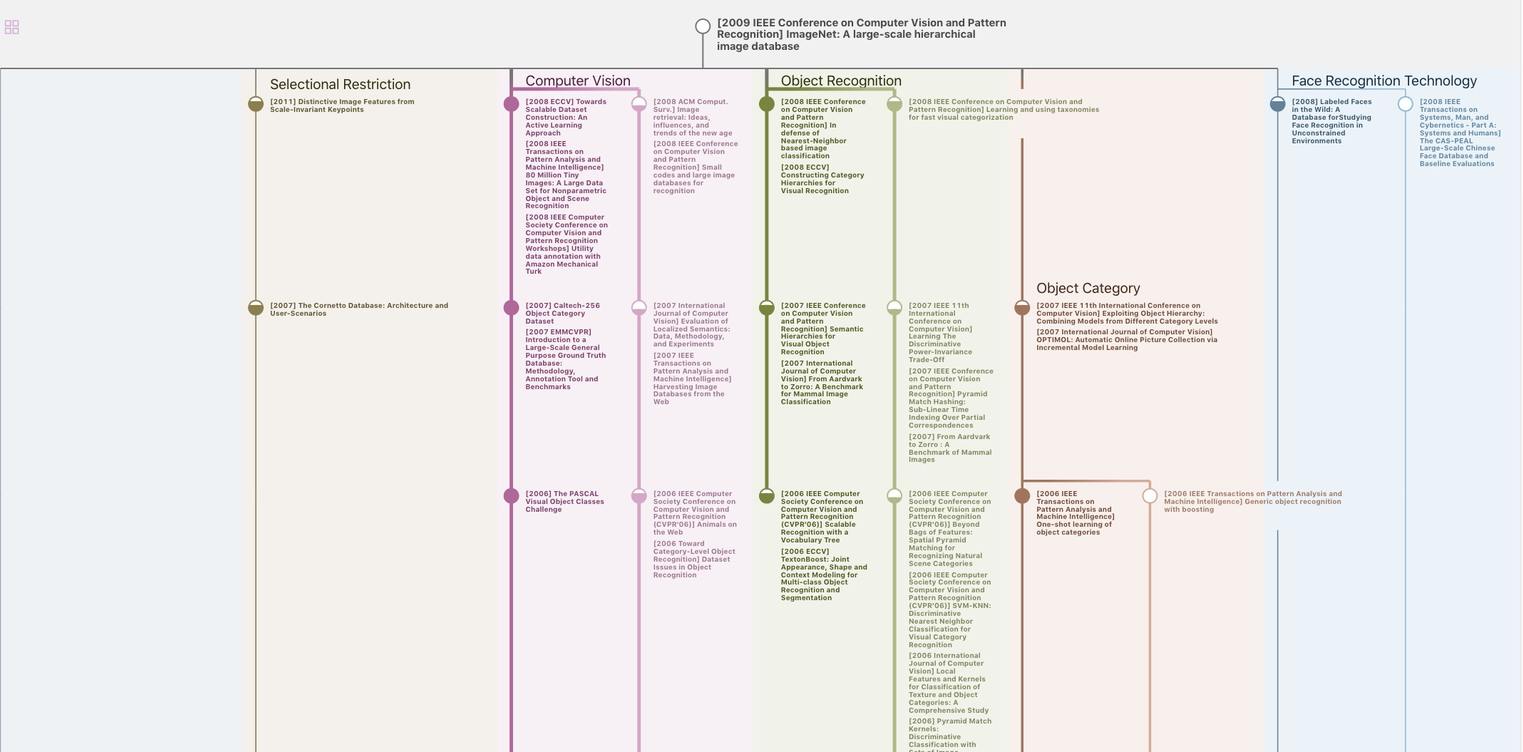
生成溯源树,研究论文发展脉络
Chat Paper
正在生成论文摘要