MAP approximation to the variational Bayes Gaussian mixture model and application
Soft Comput.(2017)
摘要
The learning of variational inference can be widely seen as first estimating the class assignment variable and then using it to estimate parameters of the mixture model. The estimate is mainly performed by computing the expectations of the prior models. However, learning is not exclusive to expectation. Several authors report other possible configurations that use different combinations of maximization or expectation for the estimation. For instance, variational inference is generalized under the expectation–expectation (EE) algorithm. Inspired by this, another variant known as the maximization–maximization (MM) algorithm has been recently exploited on various models such as Gaussian mixture, Field-of-Gaussians mixture, and sparse-coding-based Fisher vector. Despite the recent success, MM is not without issue. Firstly, it is very rare to find any theoretical study comparing MM to EE. Secondly, the computational efficiency and accuracy of MM is seldom compared to EE. Hence, it is difficult to convince the use of MM over a mainstream learner such as EE or even Gibbs sampling. In this work, we revisit the learning of EE and MM on a simple Bayesian GMM case. We also made theoretical comparison of MM with EE and found that they in fact obtain near identical solutions. In the experiments, we performed unsupervised classification, comparing the computational efficiency and accuracy of MM and EE on two datasets. We also performed unsupervised feature learning, comparing Bayesian approach such as MM with other maximum likelihood approaches on two datasets.
更多查看译文
关键词
Variational Bayes, Gaussian mixture model, Expectation maximization, Image classification
AI 理解论文
溯源树
样例
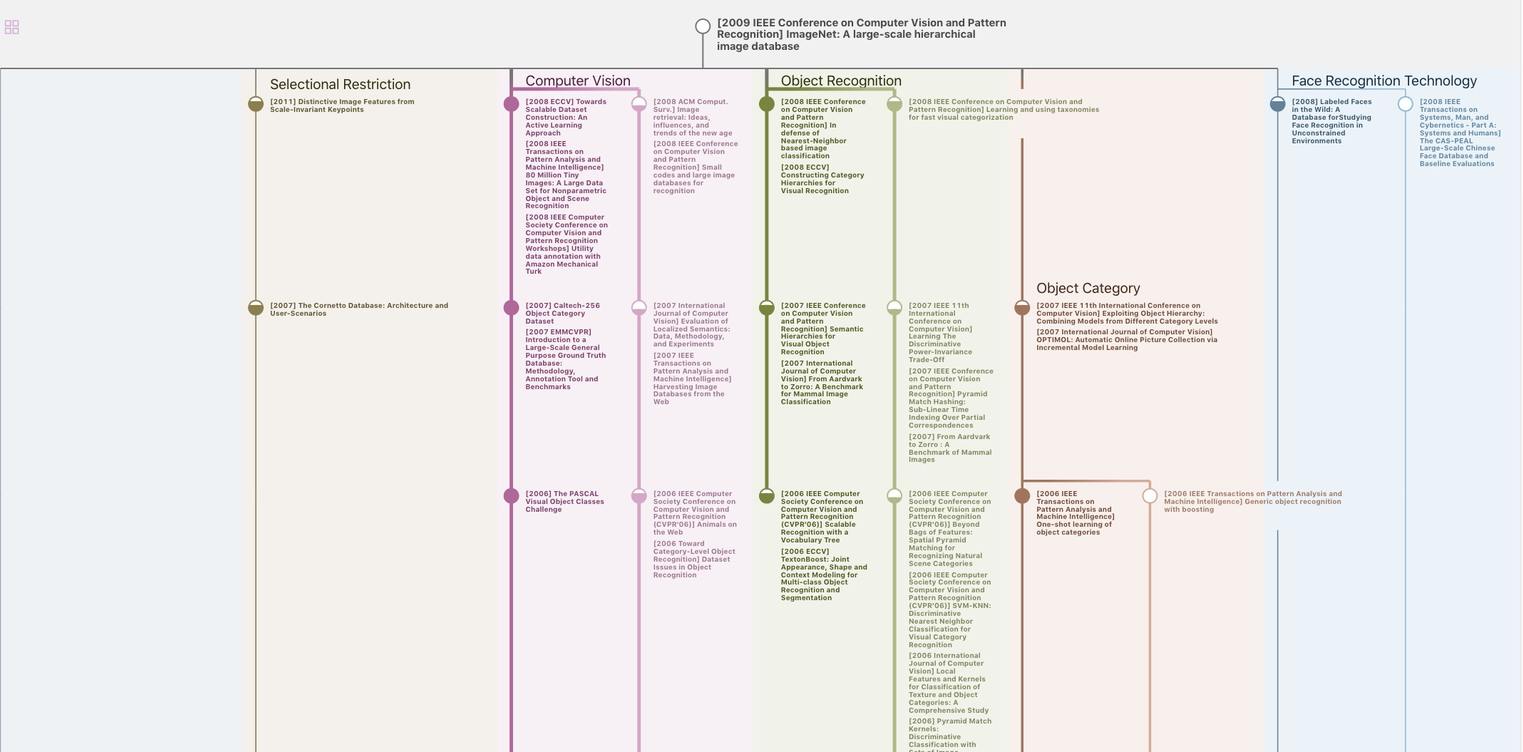
生成溯源树,研究论文发展脉络
Chat Paper
正在生成论文摘要