Isometric parameters in the monitoring of maximal strength, power, and hypertrophic resistance-training.
APPLIED PHYSIOLOGY NUTRITION AND METABOLISM(2018)
摘要
This study monitored strength-training adaptations via isometric parameters throughout 2 x 10 weeks of hypertrophic (HYP I-II) or 10 weeks maximum strength (MS) followed by 10 weeks power (P) training with untrained controls. Trainees performed bilateral isometric leg press tests analyzed for peak force (maximal voluntary contraction (MVC)) and rate of force development (RFD) every 3.5 weeks. These parameters were compared with dynamic performance, voluntary and electrically induced isometric contractions, muscle activity, and cross-sectional area (CSA) in the laboratory before and after 10 and 20 weeks. RFD increased similarly during the first 7 weeks (HYP I, 44% +/- 53%; MS, 48% +/- 55%, P < 0.05), but RFD continued to increase up to 65% +/- 61% from baseline (P < 0.01) only during P. These increases were concomitant with enhanced dynamic performances of 1-repetition maximum (1RM) (HYP I, 8% +/- 6%; MS, 11% +/- 6%, P < 0.001), and explosive repetitions during P (11% +/- 15%, P < 0.05). Time to reach peak RFD differed (P < 0.001) between HYP (mean 42 +/- 20 ms) and MS-P (mean 31 +/- 12 ms) groups because of training. The changes in MVC correlated with the changes in CSA during weeks 1-20 (HYP I-II, r = 0.664; MS-P, r = 0.595, P = 0.05), as well as changes in 1RM (r = 0.724, P < 0.05) during weeks 11-20 (HYP II). Muscle activity increased during MS and P only. Both MVC and RFD improvements reflected combinations of central and peripheral adaptations. RFD parameters may be effective tools to evaluate adaptations, particularly during maximal strength/power training, while MVC cannot distinguish between strength or muscle mass changes. Monitoring RFD provided important information regarding plateaus in RFD improvement, which were observed in dynamic explosive performances after HYP II compared with P.
更多查看译文
关键词
RFD,MVC,strength training,monitoring
AI 理解论文
溯源树
样例
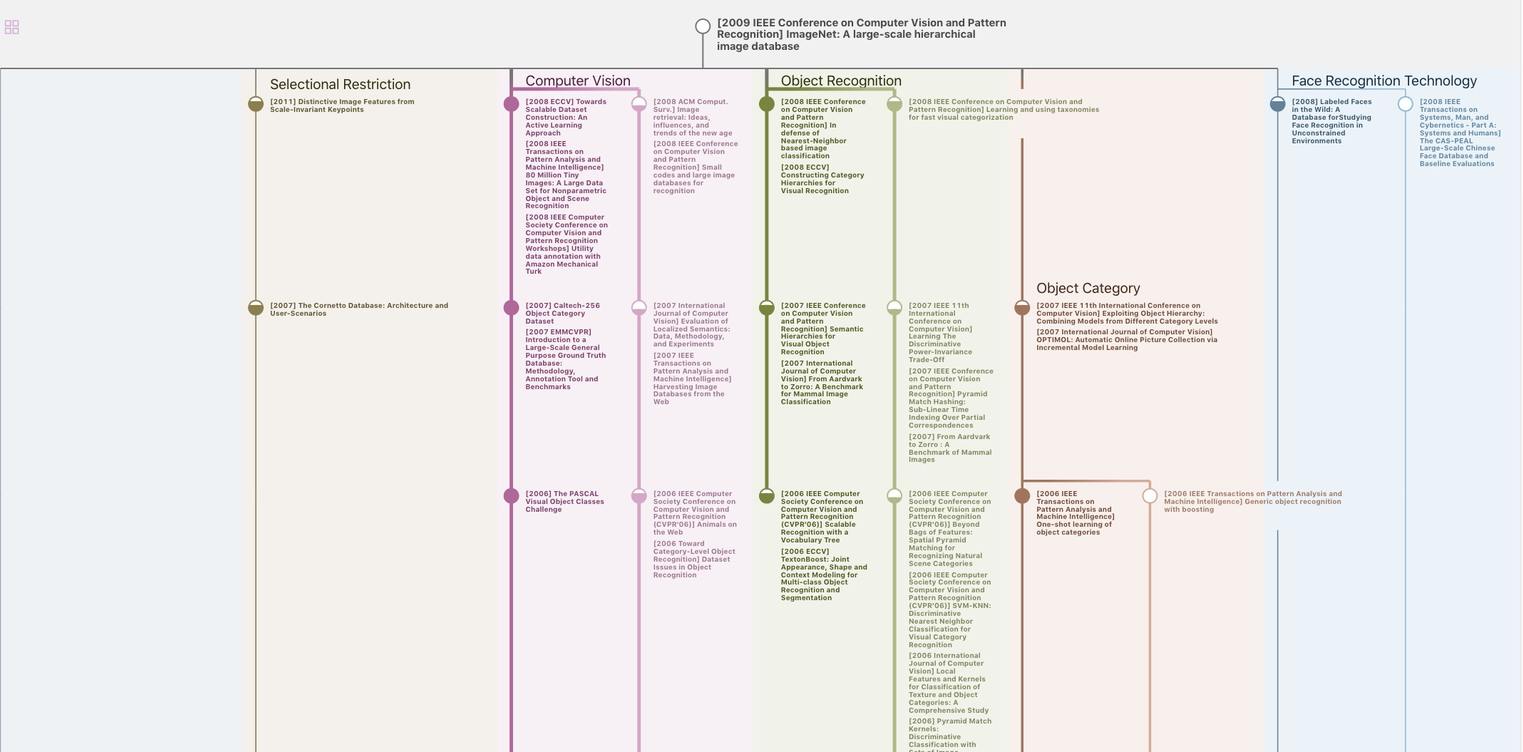
生成溯源树,研究论文发展脉络
Chat Paper
正在生成论文摘要