Singular value decomposition-based 2D image reconstruction for computed tomography.
JOURNAL OF X-RAY SCIENCE AND TECHNOLOGY(2017)
摘要
Singular value decomposition (SVD)-based 2D image reconstruction methods are developed and evaluated for a broad class of inverse problems for which there are no analytical solutions. The proposed methods are fast and accurate for reconstructing images in a non-iterative fashion. The multi-resolution strategy is adopted to reduce the size of the system matrix to reconstruct large images using limited memory capacity. A modified high-contrast Shepp-Logan phantom, a lowcontrast FORBILD head phantom, and a physical phantom are employed to evaluate the proposed methods with different system configurations. The results show that the SVD methods can accurately reconstruct images from standard scan and interior scan projections and that they outperform other benchmark methods. The general SVD method outperforms the other SVD methods. The truncated SVD and Tikhonov regularized SVD methods accurately reconstruct a region-of-interest (ROI) from an internal scan with a known sub-region inside the ROI. Furthermore, the SVD methods are much faster and more flexible than the benchmark algorithms, especially in the ROI reconstructions in our experiments.
更多查看译文
关键词
Computed tomography,singular value decomposition,a region of interest,interior tomography,Tikhonov regularization
AI 理解论文
溯源树
样例
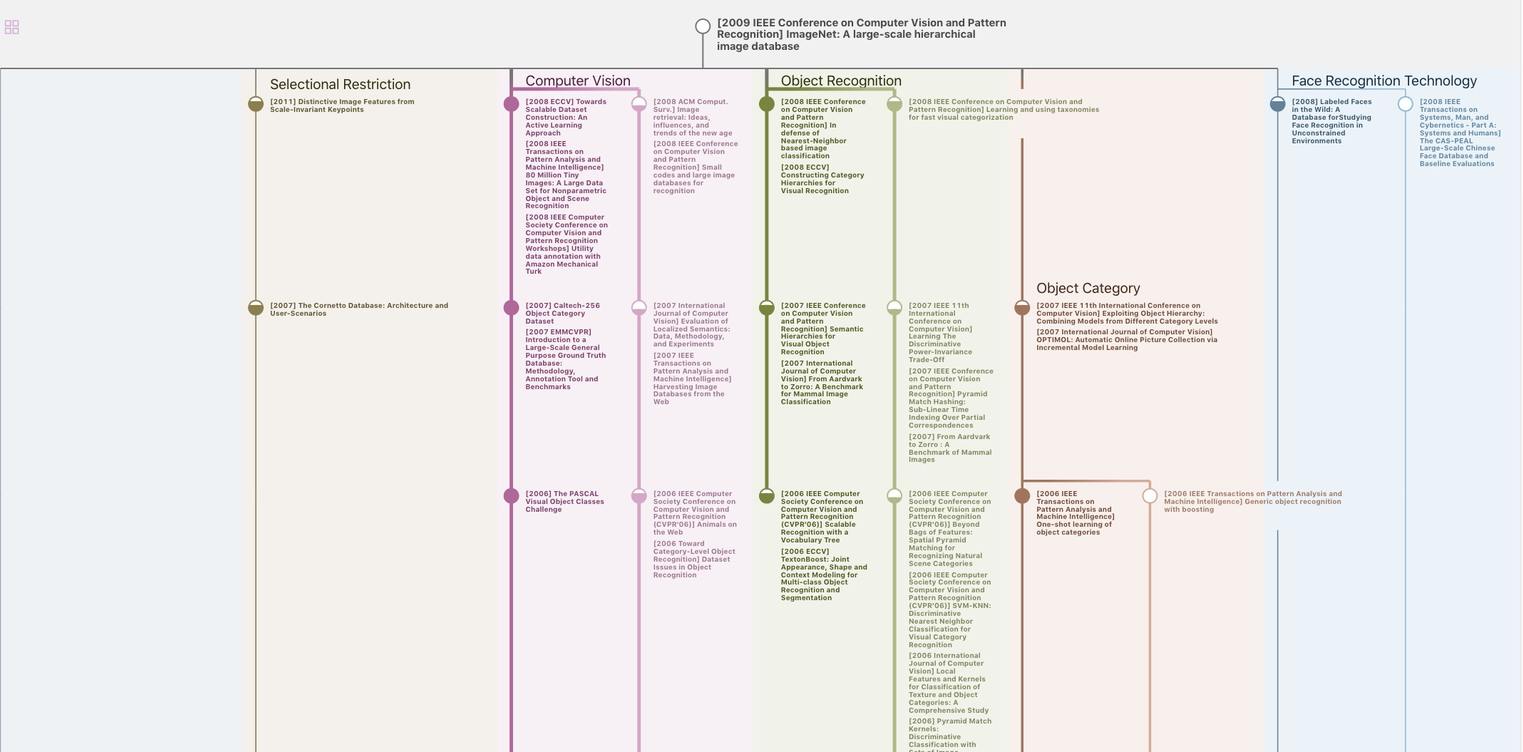
生成溯源树,研究论文发展脉络
Chat Paper
正在生成论文摘要