Identification and Correction of Additive and Multiplicative Spatial Biases in Experimental High-Throughput Screening.
SLAS DISCOVERY(2018)
摘要
Data generated by high-throughput screening (HTS) technologies are prone to spatial bias. Traditionally, bias correction methods used in HTS assume either a simple additive or, more recently, a simple multiplicative spatial bias model. These models do not, however, always provide an accurate correction of measurements in wells located at the intersection of rows and columns affected by spatial bias. The measurements in these wells depend on the nature of interaction between the involved biases. Here, we propose two novel additive and two novel multiplicative spatial bias models accounting for different types of bias interactions. We describe a statistical procedure that allows for detecting and removing different types of additive and multiplicative spatial biases from multiwell plates. We show how this procedure can be applied by analyzing data generated by the four HTS technologies (homogeneous, microorganism, cell-based, and gene expression HTS), the three high-content screening (HCS) technologies (area, intensity, and cell-count HCS), and the only small-molecule microarray technology available in the ChemBank small-molecule screening database. The proposed methods are included in the AssayCorrector program, implemented in R, and available on CRAN.
更多查看译文
关键词
Anderson-Darling test,Cramer-von Mises test,data correction,high-content screening,high-throughput screening,Mann-Whitney U test,partial mean polish,small-molecule microarray,spatial bias
AI 理解论文
溯源树
样例
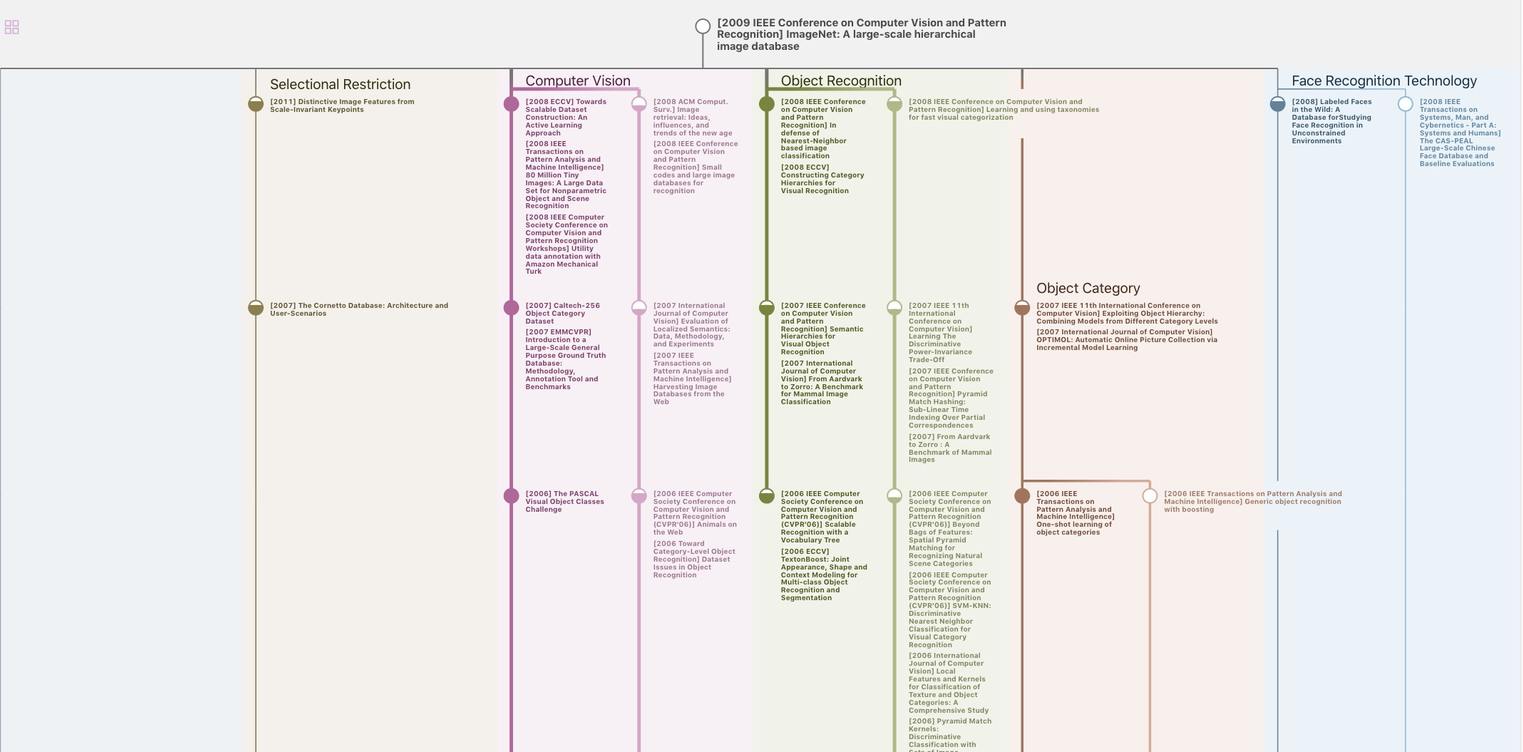
生成溯源树,研究论文发展脉络
Chat Paper
正在生成论文摘要