When Neuroscience Met Clinical Pathology: Partitioning Experimental Variation To Aid Data Interpretation In Neuroscience
EUROPEAN JOURNAL OF NEUROSCIENCE(2018)
摘要
In animal experiments, neuroscientists typically assess the effectiveness of interventions by comparing the average response of groups of treated and untreated animals. While providing useful insights, focusing only on group effects risks overemphasis of small, statistically significant but physiologically unimportant, differences. Such differences can be created by analytical variability or physiological within-individual variation, especially if the number of animals in each group is small enough that one or two outlier values can have considerable impact on the summary measures for the group. Physicians face a similar dilemma when comparing two results from the same patient. To determine whether the change between two values reflects disease progression or known analytical and physiological variation, the magnitude of the difference between two results is compared to the reference change value. These values are generated by quantifying analytical and within-individual variation, and differences between two results from the same patient are considered clinically meaningful only if they exceed the combined effect of these two sources of noise'. In this article, we describe how the reference change interval can be applied within neuroscience. This form of analysis provides a measure of outcome at an individual level that complements traditional group-level comparisons, and therefore, introduction of this technique into neuroscience can enrich interpretation of experimental data. It can also safeguard against some of the possible misinterpretations that may occur during analysis of the small experimental groups that are common in neuroscience and, by illuminating analytical error, may aid in design of more efficient experimental methods.
更多查看译文
关键词
intraindividual, partition, reference change value, translation, variability
AI 理解论文
溯源树
样例
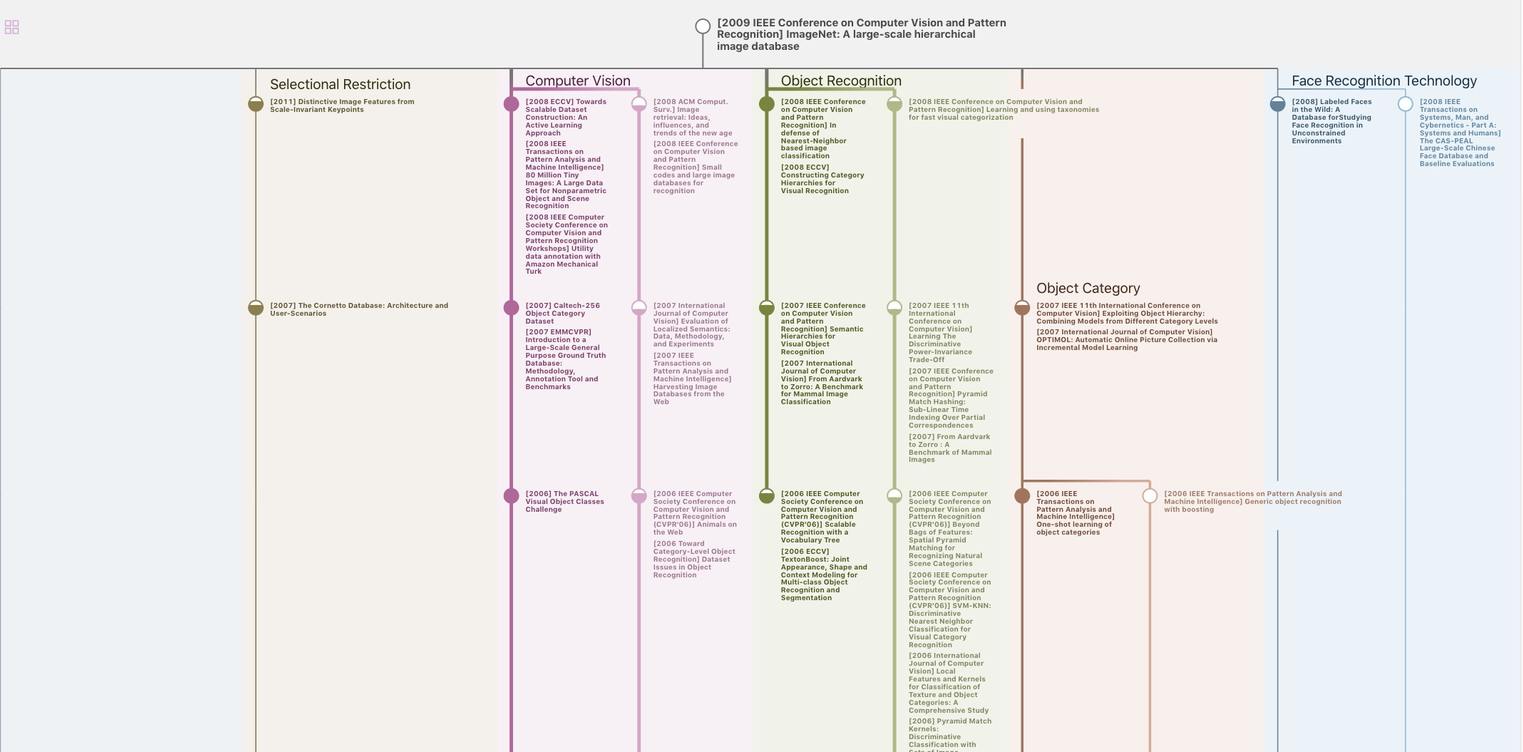
生成溯源树,研究论文发展脉络
Chat Paper
正在生成论文摘要