A Robust and Efficient Feature Selection Algorithm for Microarray Data.
MOLECULAR INFORMATICS(2017)
摘要
In the past decades, a few synergistic feature selection algorithms have been published, which includes Cooperative Index (CI) and K-Top Scoring Pair (k-TSP). These algorithms consider the synergistic behavior of features when they are included in a feature panel. Although promising results have been shown for these algorithms, there is lack of a comprehensive and fair comparison with other feature selection algorithms across a large number of microarray datasets in terms of classification accuracy and computational complexity. There is a need in evaluating their performance and reducing the complexity of such algorithms. We compared the performance of synergistic feature selection algorithms with 11 other commonly used algorithms based on 22 microarray gene expression binary class datasets. The evaluation confirms that synergistic algorithms such as CI and k-TSP will gradually increase the classification performance as more features are used in the classifiers. Also, in order to cut down computational cost, we proposed a new feature selection ranking score called Positive Synergy Index (PSI). Testing results show that features selected using PSI as well as synergistic feature selection algorithms provide better performance compared to with all other methods, while PSI has a computational complexity significantly lower than that of other synergistic algorithms.
更多查看译文
关键词
Feature selection,microarray binary class dataset,Synergy based methods
AI 理解论文
溯源树
样例
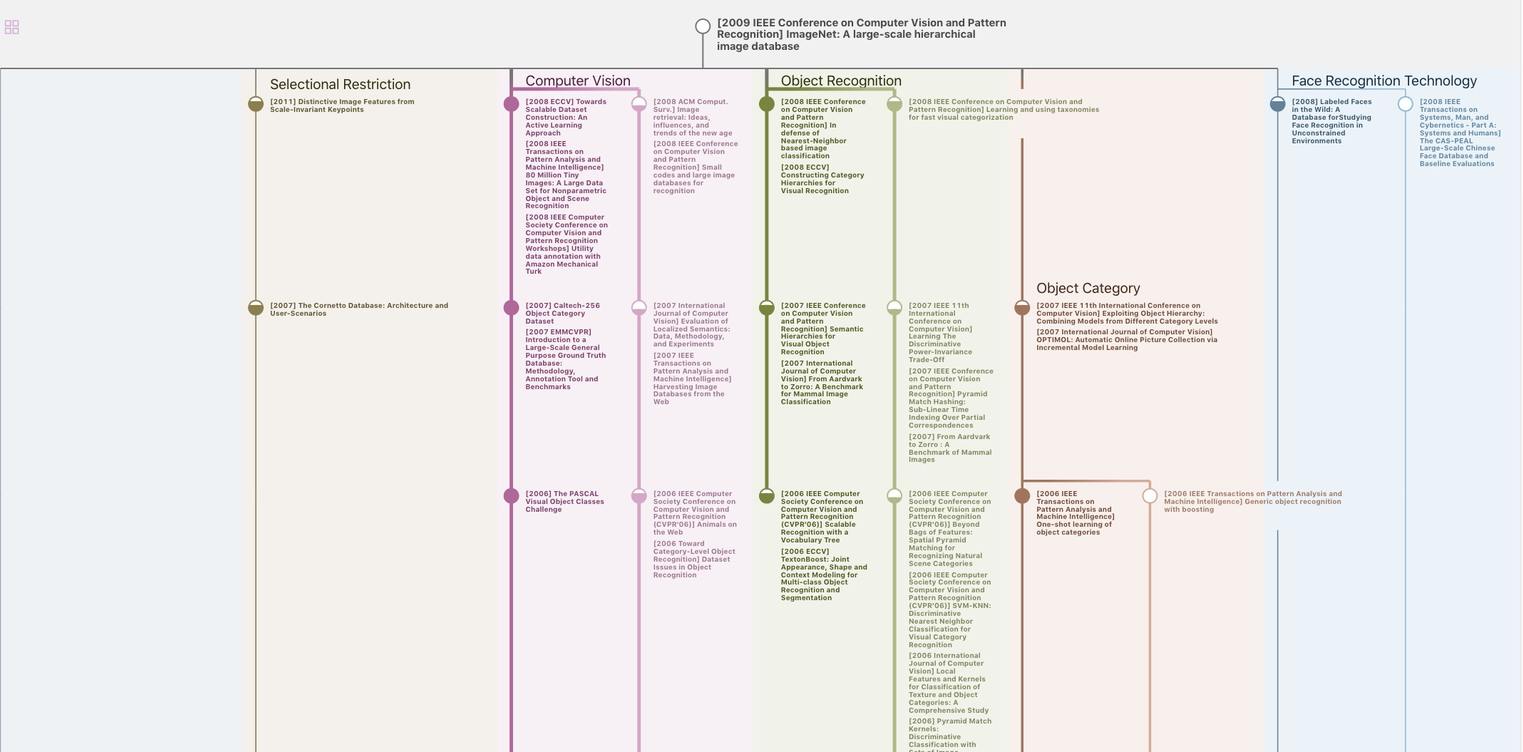
生成溯源树,研究论文发展脉络
Chat Paper
正在生成论文摘要