Family of boundary overlap metrics for the evaluation of medical image segmentation.
JOURNAL OF MEDICAL IMAGING(2018)
摘要
All medical image segmentation algorithms need to be validated and compared, yet no evaluation framework is widely accepted within the imaging community. None of the evaluation metrics that are popular in the literature are consistent in the way they rank segmentation results: they tend to be sensitive to one or another type of segmentation error (size, location, and shape) but no single metric covers all error types. We introduce a family of metrics, with hybrid characteristics. These metrics quantify the similarity or difference of segmented regions by considering their average overlap in fixed-size neighborhoods of points on the boundaries of those regions. Our metrics are more sensitive to combinations of segmentation error types than other metrics in the existing literature. We compare the metric performance on collections of segmentation results sourced from carefully compiled two-dimensional synthetic data and three-dimensional medical images. We show that our metrics: (1) penalize errors successfully, especially those around region boundaries; (2) give a low similarity score when existing metrics disagree, thus avoiding overly inflated scores; and (3) score segmentation results over a wider range of values. We analyze a representative metric from this family and the effect of its free parameter on error sensitivity and running time. (C) 2018 Society of Photo-Optical Instrumentation Engineers (SPIE)
更多查看译文
关键词
image segmentation evaluation,medical image segmentation,evaluation metrics,boundary overlap,symmetric boundary Dice
AI 理解论文
溯源树
样例
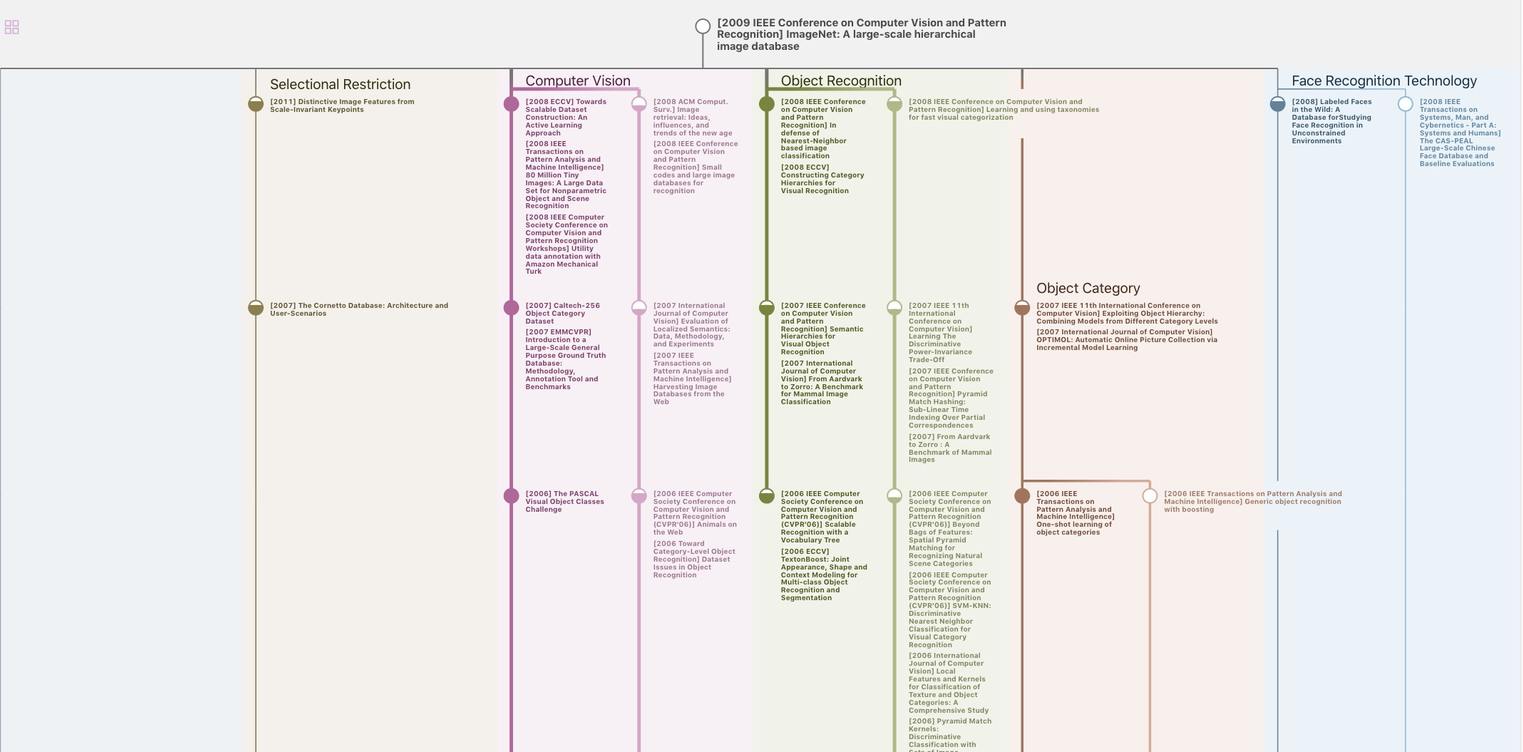
生成溯源树,研究论文发展脉络
Chat Paper
正在生成论文摘要