Development and Evaluation of Novel Statistical Methods in Urine Biomarker-Based Hepatocellular Carcinoma Screening
SCIENTIFIC REPORTS(2018)
摘要
Hepatocellular carcinoma is one of the fastest growing cancers in the US and has a low survival rate, partly due to difficulties in early detection. Because of HCC’s high heterogeneity, it has been suggested that multiple biomarkers would be needed to develop a sensitive HCC screening test. This study applied random forest (RF), a machine learning technique, and proposed two novel models, fixed sequential (FS) and two-step (TS), for comparison with two commonly used statistical techniques, logistic regression (LR) and classification and regression trees (CART), in combining multiple urine DNA biomarkers for HCC screening using biomarker values obtained from 137 HCC and 431 non-HCC (224 hepatitis and 207 cirrhosis) subjects. The sensitivity, specificity, area under the receiver operating curve, and variability were estimated through repeated 10-fold cross-validation to compare the models’ performances in accuracy and robustness. We show that RF and TS have higher accuracy and stability; specifically, they reach 90% specificity and 86%/87% sensitivity respectively along with 15% higher sensitivity and 10% higher specificity than LR in cross-validation. The potential of RF and TS to develop a panel of multiple biomarkers and the possibility for self-training, cloud-based models for HCC screening are discussed.
更多查看译文
关键词
carcinoma,novel statistical methods,biomarker-based
AI 理解论文
溯源树
样例
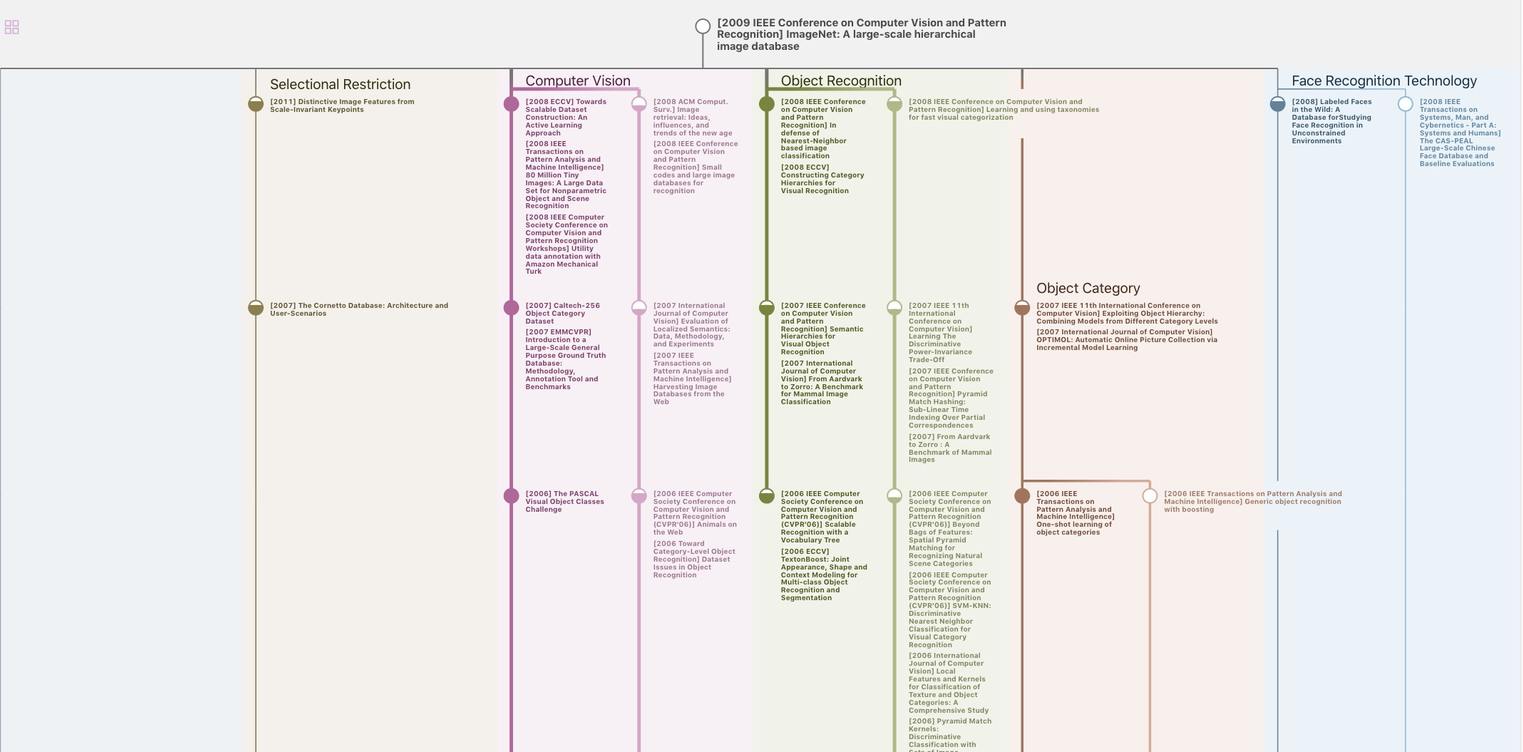
生成溯源树,研究论文发展脉络
Chat Paper
正在生成论文摘要