Peptide identifications and false discovery rates using different mass spectrometry platforms.
Talanta(2018)
摘要
Characterization of endogenous neuropeptides produced from post-translational proteolytic processing of precursor proteins is a demanding task. A variety of complex prohormone processing steps generate molecular diversity from neuropeptide prohormones, making in silico neuropeptide discovery difficult. In addition, the wide range of endogenous peptide concentrations as well as significant peptide complexity further challenge the structural characterization of neuropeptides. Liquid chromatography-mass spectrometry (MS), performed in conjunction with bioinformatics, allows for high-throughput characterization of peptides. Mass analyzers and molecular dissociation techniques render specific characteristics to the acquired data and thus, influence the analysis of the MS data using bioinformatic algorithms for follow-up peptide identification. Here we evaluated the efficacy of several distinct peptidomic workflows using two mass spectrometers, the Thermo Orbitrap Fusion Tribrid and Bruker Impact HD UHR-QqTOF, for confident peptide discovery and characterization. We compared the results in several categories, including the numbers of identified peptides, full-length mature neuropeptides among all identifications, and precursor proteins mapped by the identified peptides. We also characterized the peptide false discovery rate (FDR) based on the occurrence of amidation, a known post-translational modification (PTM) that has been shown to require the presence of a C-terminal glycine. Thus, amidation events without a preceding glycine were considered false-positive amidation assignments. We compared the FDR calculated by the search engine used here to the minimum FDR estimated via false amidation assignments. The search engine severely underestimated the rate of false PTM assignments among the identified peptides, regardless of the specific MS platform used.
更多查看译文
关键词
Neuropeptides,Amidation,De novo sequencing,Peptidomics,Proteomics
AI 理解论文
溯源树
样例
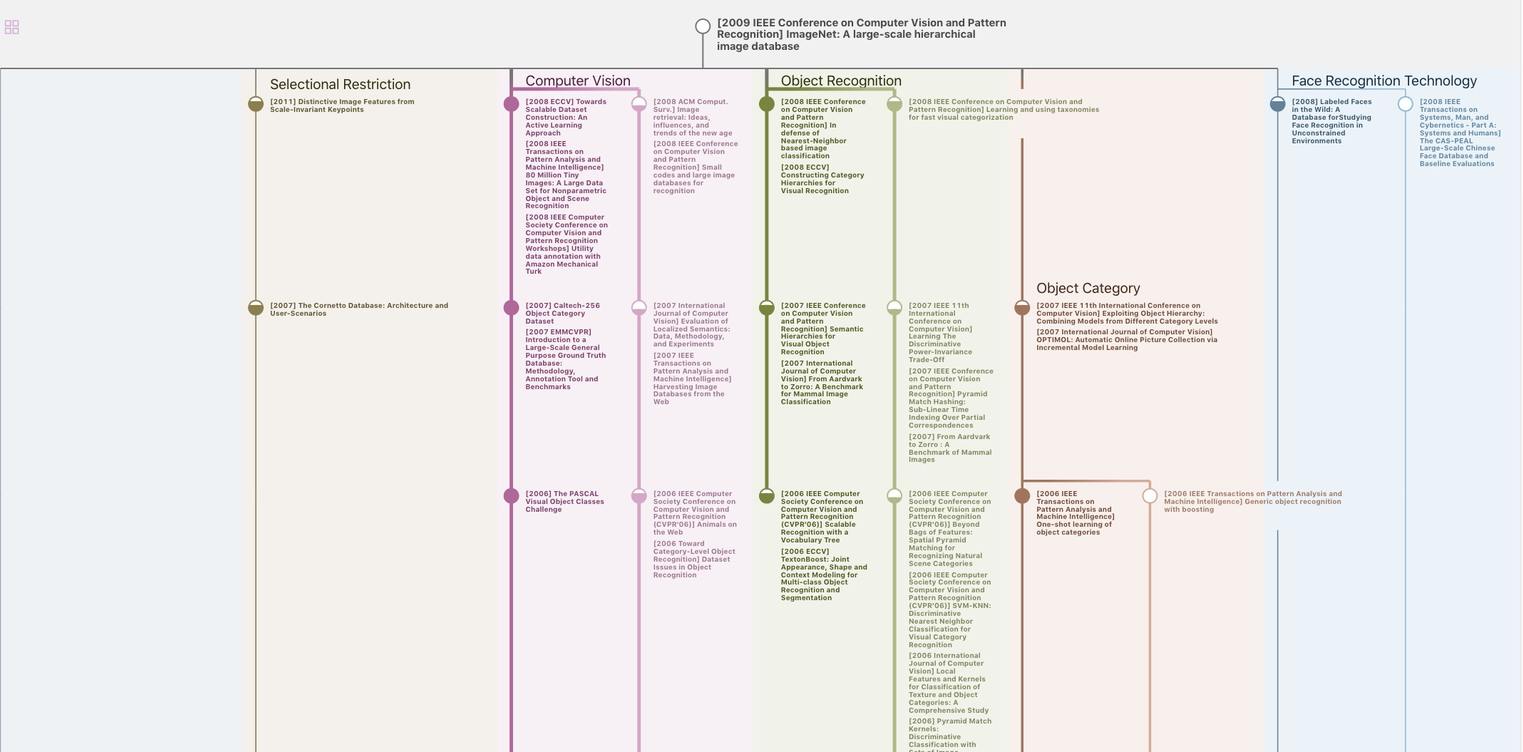
生成溯源树,研究论文发展脉络
Chat Paper
正在生成论文摘要