Repurposing High-Throughput Image Assays Enables Biological Activity Prediction for Drug Discovery.
Cell Chemical Biology(2018)
摘要
In both academia and the pharmaceutical industry, large-scale assays for drug discovery are expensive and often impractical, particularly for the increasingly important physiologically relevant model systems that require primary cells, organoids, whole organisms, or expensive or rare reagents. We hypothesized that data from a single high-throughput imaging assay can be repurposed to predict the biological activity of compounds in other assays, even those targeting alternate pathways or biological processes. Indeed, quantitative information extracted from a three-channel microscopy-based screen for glucocorticoid receptor translocation was able to predict assay-specific biological activity in two ongoing drug discovery projects. In these projects, repurposing increased hit rates by 50- to 250-fold over that of the initial project assays while increasing the chemical structure diversity of the hits. Our results suggest that data from high-content screens are a rich source of information that can be used to predict and replace customized biological assays.
更多查看译文
关键词
drug discovery,high-throughput screening,high-content imaging,machine learning,matrix factorization,Bayesian matrix factorization,deep learning,computational chemistry
AI 理解论文
溯源树
样例
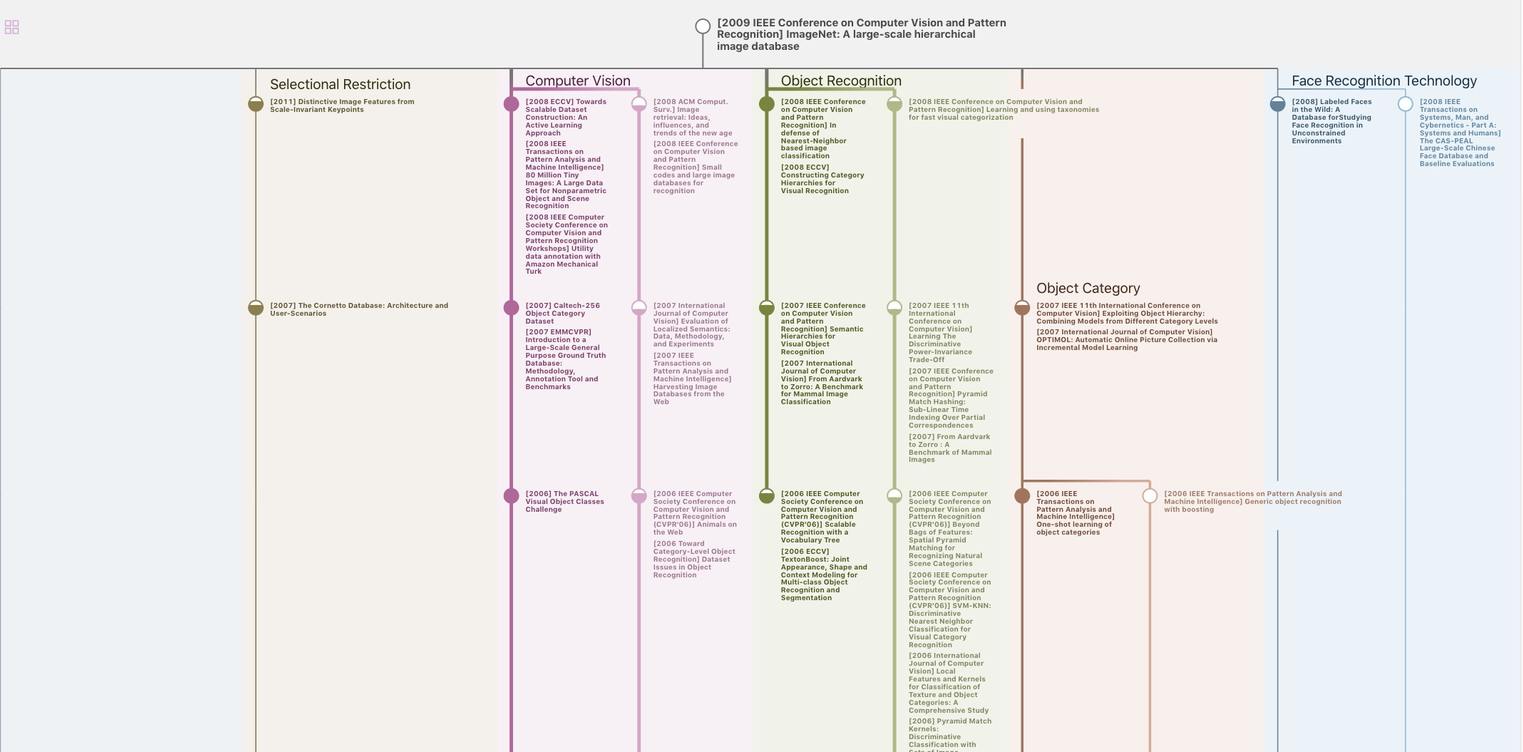
生成溯源树,研究论文发展脉络
Chat Paper
正在生成论文摘要