Multi-Modality Sparse Representation for Alzheimer's Disease Classification.
JOURNAL OF ALZHEIMERS DISEASE(2018)
摘要
Background: Alzheimer's disease (AD) and mild cognitive impairment (MCI) are age-related neurodegenerative diseases characterized by progressive loss of memory and irreversible cognitive functions. The hippocampus, a brain area critical for learning and memory processes, is especially susceptible to damage at early stages of AD. Objective: We aimed to develop prediction model using a multi-modality sparse representation approach. Methods: We proposed a sparse representation approach to the hippocampus using structural Tl-weighted magnetic resonance imaging (MRI) and 18-fluorodeoxyglucose-positron emission tomography (FDG-PET) to distinguish AD/MCI from healthy control subjects (HCs). We considered structural and function information for the hippocampus and applied a sparse patch-based approach to effectively reduce the dimensions of neuroimaging biomarkers. Results: In experiments using Alzheimer's Disease Neuroimaging Initiative data, our proposed method demonstrated more reliable than previous classification studies. The effects of different parameters on segmentation accuracy were also evaluated. The mean classification accuracy obtained with our proposed method was 0.94 for AD/HCs, 0.82 for MCI/HCs, and 0.86 for AD/MCI. Conclusion: We extracted multi-modal features from automatically defined hippocampal regions of training subjects and found this method to be discriminative and robust for AD and MCI classification. The extraction of features in T1 and FDG-PET images is expected to improve classification performance due to the relationship between brain structure and function.
更多查看译文
关键词
Alzheimer's disease,mild cognitive impairment,prediction model,sparse representation
AI 理解论文
溯源树
样例
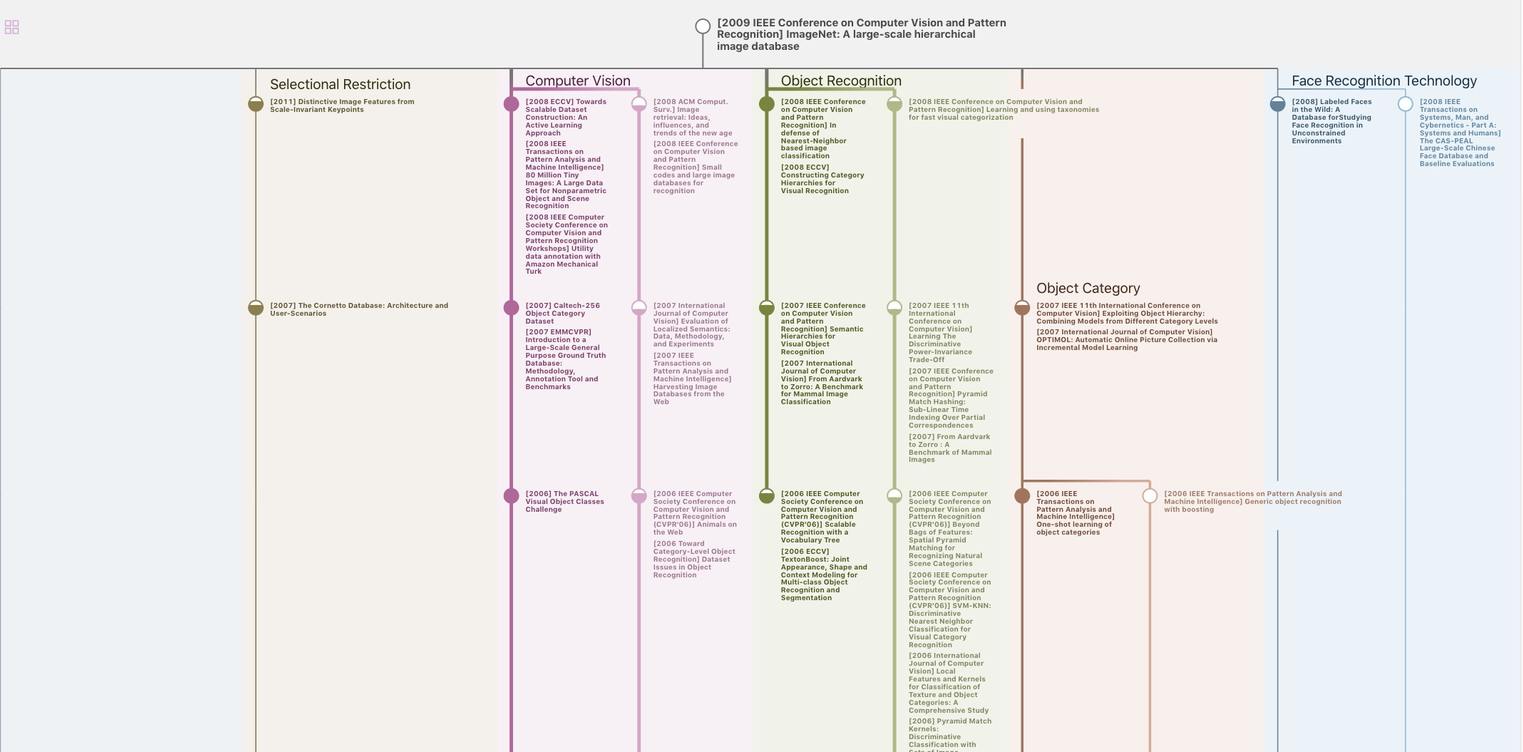
生成溯源树,研究论文发展脉络
Chat Paper
正在生成论文摘要